Geo6D: Geometric Constraints Learning for 6D Pose Estimation
CoRR(2023)
Abstract
Existing direct 6D pose estimation methods regress target 6D poses without the need for post-processing, making them effective and easy to develop. However, due to the lack of geometric constraints, the precision of these approaches remains limited, and more training data are required to achieve better performance. To this end, we propose a geometric constraint-based 6D pose estimation method (Geo6D), which establishes an explicit geometric constraint between the input and the regression target. Specifically, a Geo head is proposed to build a point-to-point relationship between the camera frame and the object frame. And additional inputs are introduced as supplementary information to facilitate the learning of the geometric correlation. The proposed Geo6D can be used as a plugin in mainstream direct 6D pose estimation methods. Extensive experimental results show that when equipped with Geo6D, the direct 6D method achieves state-of-the-art performance on multiple datasets and shows significant effectiveness with limited data volume.
MoreTranslated text
AI Read Science
Must-Reading Tree
Example
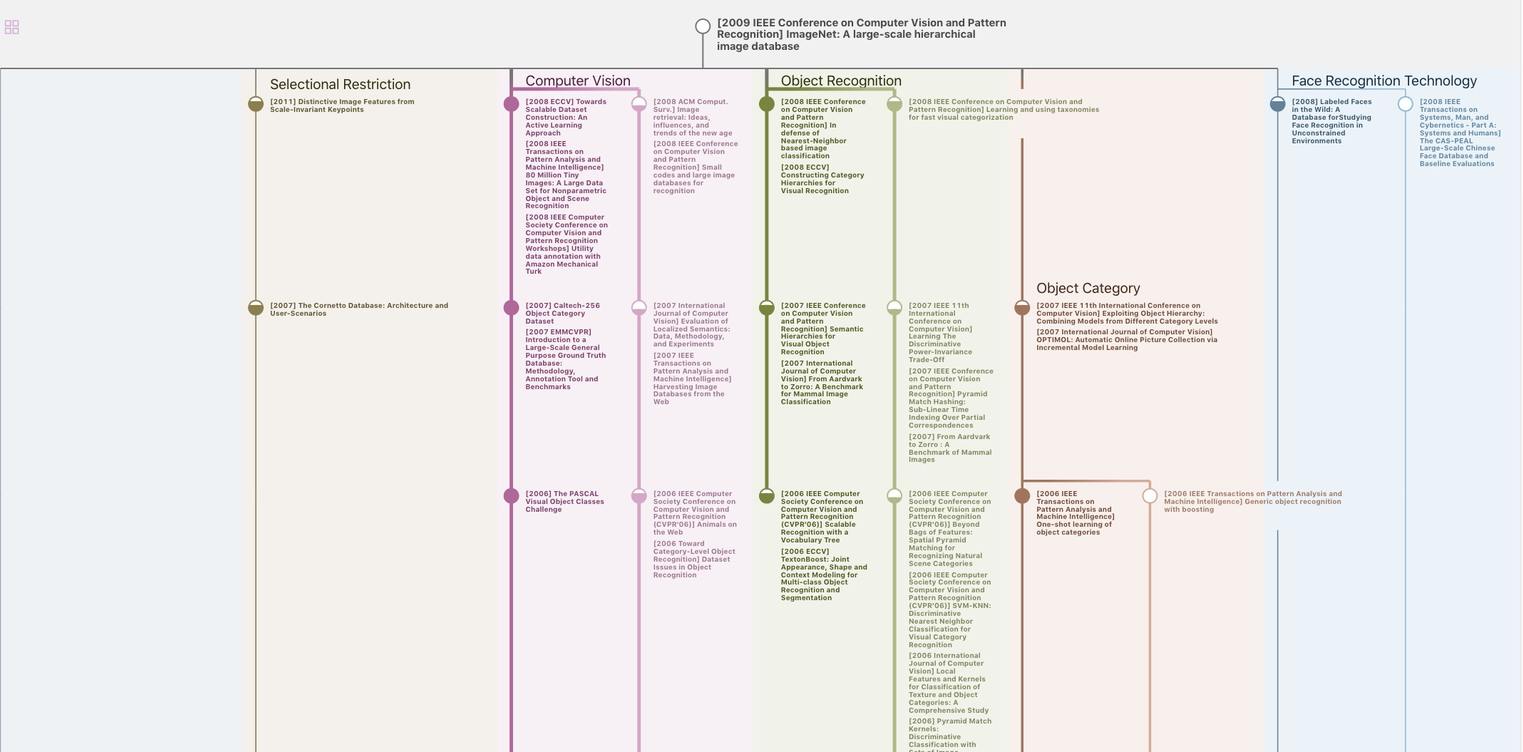
Generate MRT to find the research sequence of this paper
Chat Paper
Summary is being generated by the instructions you defined