MPOGames: Efficient Multimodal Partially Observable Dynamic Games
2023 IEEE INTERNATIONAL CONFERENCE ON ROBOTICS AND AUTOMATION, ICRA(2023)
摘要
Game theoretic methods have become popular for planning and prediction in situations involving rich multi-agent interactions. However, these methods often assume the existence of a single local Nash equilibria and are hence unable to handle uncertainty in the intentions of different agents. While maximum entropy (MaxEnt) dynamic games try to address this issue, practical approaches solve for MaxEnt Nash equilibria using linear-quadratic approximations which are restricted to unimodal responses and unsuitable for scenarios with multiple local Nash equilibria. By reformulating the problem as a POMDP, we propose MPOGames, a method for efficiently solving MaxEnt dynamic games that captures the interactions between local Nash equilibria. We show the importance of uncertainty-aware game theoretic methods via a two-agent merge case study. Finally, we prove the real-time capabilities of our approach with hardware experiments on a 1/10th scale car platform.
更多查看译文
关键词
efficient multimodal,mpogames
AI 理解论文
溯源树
样例
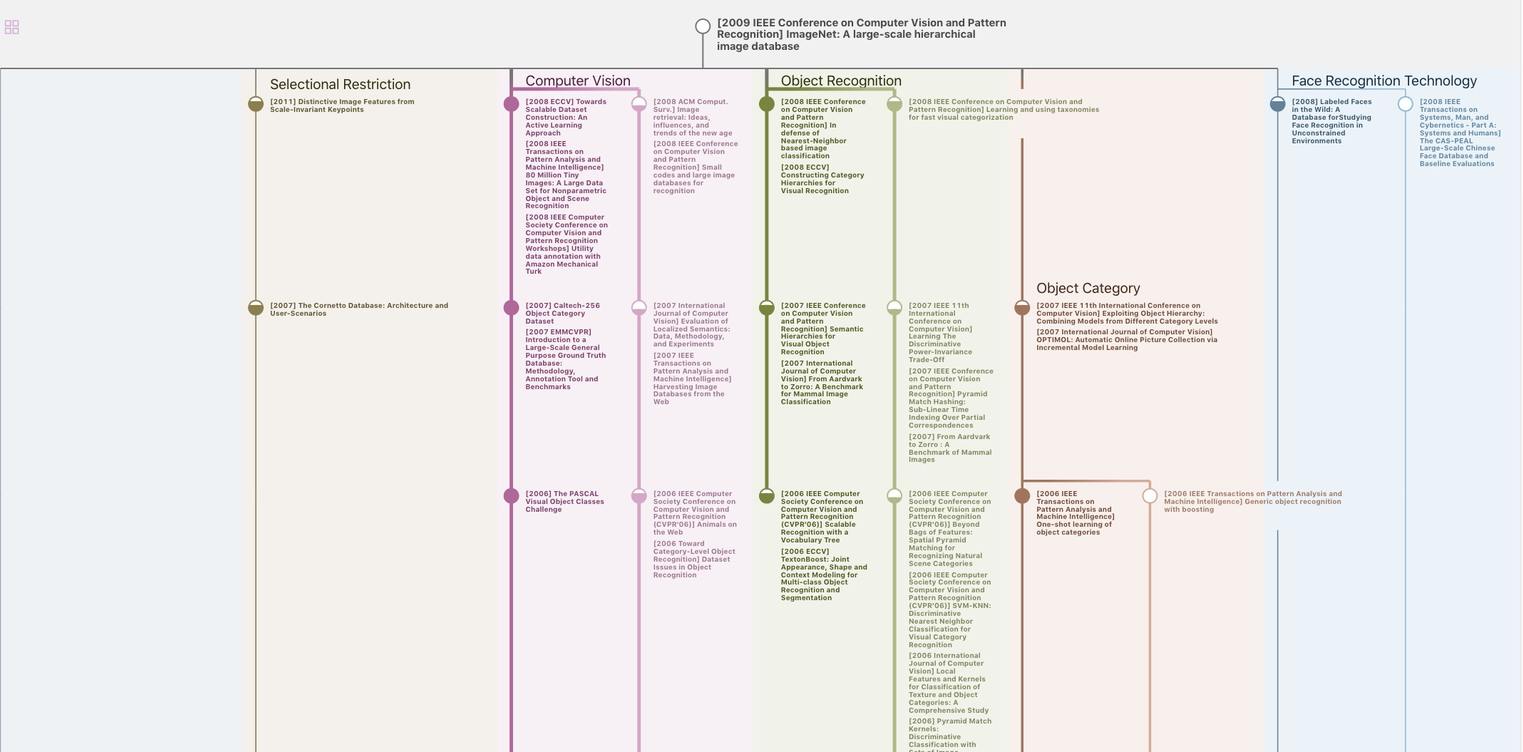
生成溯源树,研究论文发展脉络
Chat Paper
正在生成论文摘要