Leveraging spatial residual attention and temporal Markov networks for video action understanding
NEURAL NETWORKS(2024)
摘要
The effective use of temporal relationships while extracting fertile spatial features is the key to video action understanding. Video action understanding is a challenging visual task because it generally necessitates not only the features of individual key frames but also the contextual understanding of the entire video and the relationships among key frames. Temporal relationships pose a challenge to video action understanding. However, existing 3D convolutional neural network approaches are limited, with a great deal of redundant spatial and temporal information. In this paper, we present a novel two-stream approach that incorporates Spatial Residual Attention and Temporal Markov (SRATM) to learn complementary features to achieve stronger video action understanding performance. Specifically, the proposed SRATM consists of spatial residual attention and temporal Markov. Firstly, the spatial residual attention network captures effective spatial feature representation. Further, the temporal Markov network enhances the model by learning the temporal relationships via conducting probabilistic logic calculation among frames in a video. Finally, we conduct extensive experiments on four video action datasets, namely, Something-Something-V1, Something-Something-V2, Diving48, and Mini-Kinetics, show that the proposed SRATM method achieves competitive results.
更多查看译文
关键词
Video action understanding,Spatial residual attention,Temporal Markov
AI 理解论文
溯源树
样例
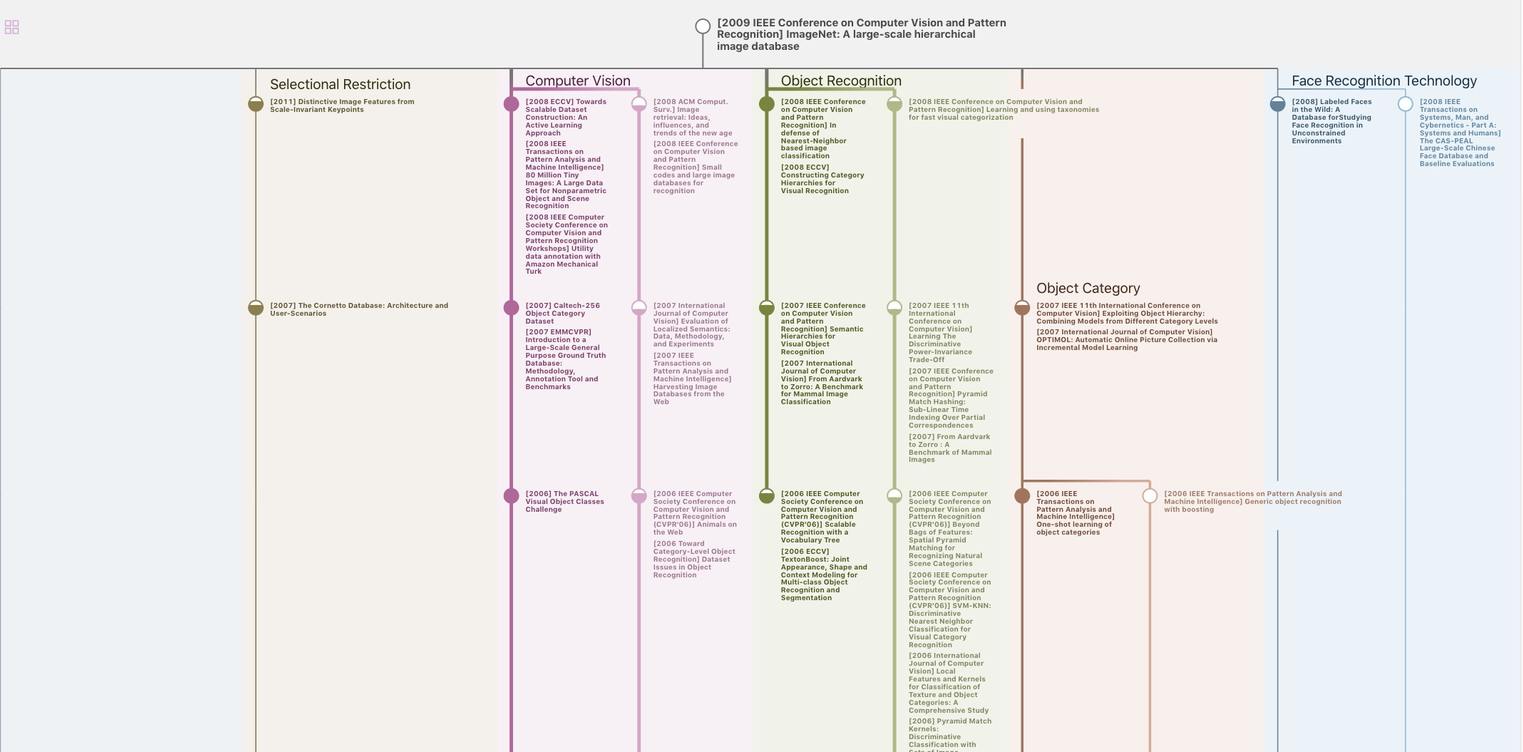
生成溯源树,研究论文发展脉络
Chat Paper
正在生成论文摘要