Deep metric learning via group channel-wise ensemble
Knowledge-Based Systems(2023)
摘要
Deep metric learning aims at learning the distance metric for data samples by deep neural networks. Essentially, it derives an embedding space where the mappings of semantically related samples are much closer than those of irrelevant ones. When learning a single metric using all samples, it fails to well encode the sample similarity in several aspects, such as color, shape, and appearance. To handle this issue, some works simply divide the linear layer into multiple sub-layers, and the data subsets for learning multiple metrics are randomly generated at sample level. This usually makes the samples in the same class appear in different subspaces. Therefore, we propose a Group Channel-wise Ensemble (GCE) method that devises a group channel-wise convolution block in convolution networks for learning multiple distance metrics. Its core idea is that the channels of convolution layer encode different similarity metrics in groups. Specifically, the embedding space is partitioned into several subspaces, where one base learner captures one distance metric. Moreover, the class-proxy based meta-class update strategy is adopted for generating data subsets. This strategy learns discriminative distance metrics by optimizing the triplet loss on training samples. Empirical studies on four benchmarks demonstrate that GCE achieves promising performances in image retrieval and clustering tasks.
更多查看译文
关键词
Deep metric learning,Group convolution,Ensemble learning,Embedding subspace,Image retrieval
AI 理解论文
溯源树
样例
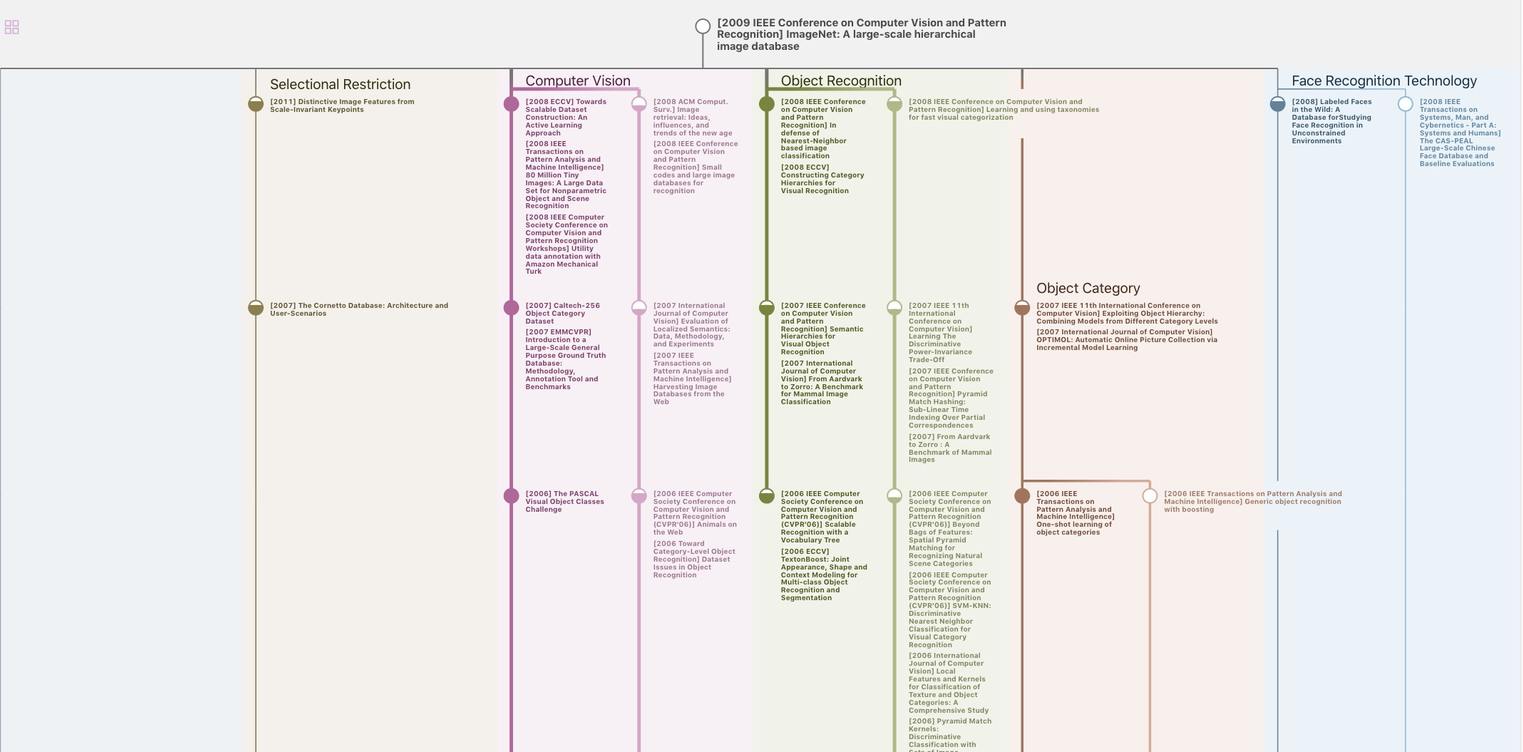
生成溯源树,研究论文发展脉络
Chat Paper
正在生成论文摘要