Towards Reliable Baselines for Document-Level Sentiment Analysis in the Czech and Slovak Languages.
Future Internet(2022)
摘要
This article helps establish reliable baselines for document-level sentiment analysis in highly inflected languages like Czech and Slovak. We revisit an earlier study representing the first comprehensive formulation of such baselines in Czech and show that some of its reported results need to be significantly revised. More specifically, we show that its online product review dataset contained more than 18% of non-trivial duplicates, which incorrectly inflated its macro F1-measure results by more than 19 percentage points. We also establish that part-of-speech-related features have no damaging effect on machine learning algorithms (contrary to the claim made in the study) and rehabilitate the Chi-squared metric for feature selection as being on par with the best performing metrics such as Information Gain. We demonstrate that in feature selection experiments with Information Gain and Chi-squared metrics, the top 10% of ranked unigram and bigram features suffice for the best results regarding online product and movie reviews, while the top 5% of ranked unigram and bigram features are optimal for the Facebook dataset. Finally, we reiterate an important but often ignored warning by George Forman and Martin Scholz that different possible ways of averaging the F1-measure in cross-validation studies of highly unbalanced datasets can lead to results differing by more than 10 percentage points. This can invalidate the comparisons of F1-measure results across different studies if incompatible ways of averaging F1 are used.
更多查看译文
关键词
document-level sentiment analysis,natural language processing,machine learning,highly inflected languages,Czech language,Slovak language,baseline correction,duplicate records
AI 理解论文
溯源树
样例
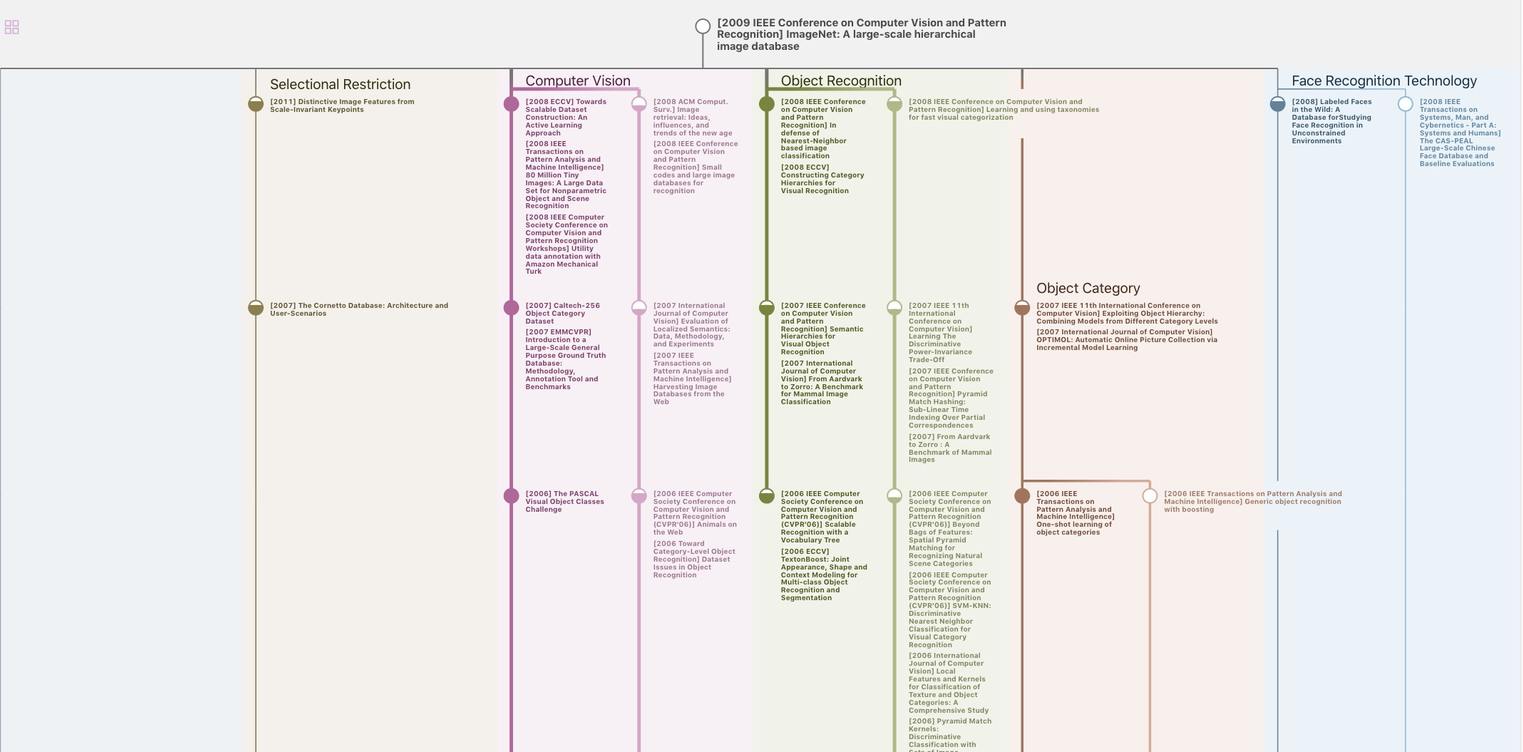
生成溯源树,研究论文发展脉络
Chat Paper
正在生成论文摘要