End-to-End Integration of Speech Recognition, Dereverberation, Beamforming, and Self-Supervised Learning Representation
2022 IEEE Spoken Language Technology Workshop (SLT)(2023)
摘要
Self-supervised learning representation (SSLR) has demonstrated its significant effectiveness in automatic speech recognition (ASR), mainly with clean speech. Recent work pointed out the strength of integrating SSLR with single-channel speech enhancement for ASR in noisy environments. This paper further advances this integration by dealing with multi-channel input. We propose a novel end-to-end architecture by integrating dereverberation, beamforming, SSLR, and ASR within a single neural network. Our system achieves the best performance reported in the literature on the CHiME-4 6-channel track with a word error rate (WER) of 1.77%. While the WavLM-based strong SSLR demonstrates promising results by itself, the end-to-end integration with the weighted power minimization distortionless response beamformer, which simultaneously performs dereverberation and denoising, improves WER significantly. Its effectiveness is also validated on the REVERB dataset.
更多查看译文
关键词
Robust automatic speech recognition,self-supervised learning,end-to-end,denoising,dereverberation
AI 理解论文
溯源树
样例
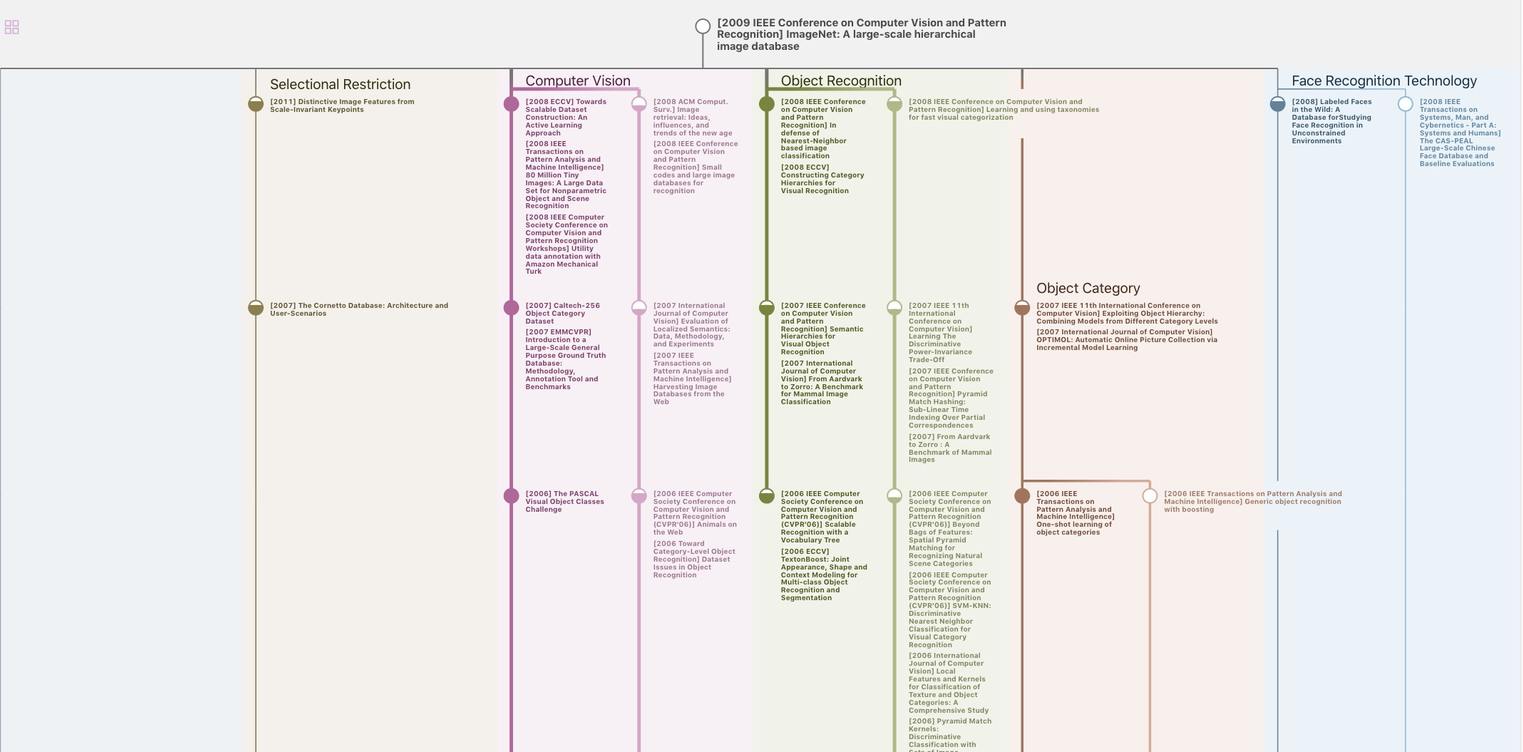
生成溯源树,研究论文发展脉络
Chat Paper
正在生成论文摘要