Propagating variational model uncertainty for bioacoustic call label smoothing
Patterns(2024)
摘要
Along with propagating the input toward making a prediction, Bayesian neural networks also propagate uncertainty. This has the potential to guide the training process by rejecting predictions of low confidence, and recent variational Bayesian methods can do so without Monte Carlo sampling of weights. Here, we apply sample-free methods for wildlife call detection on recordings made via passive acoustic monitoring equipment in the animals’ natural habitats. We further propose uncertainty-aware label smoothing, where the smoothing probability is dependent on sample-free predictive uncertainty, in order to downweigh data samples that should contribute less to the loss value. We introduce a bioacoustic dataset recorded in Malaysian Borneo, containing overlapping calls from 30 species. On that dataset, our proposed method achieves an absolute percentage improvement of around 1.5 points on area under the receiver operating characteristic (AU-ROC), 13 points in F1, and 19.5 points in expected calibration error (ECE) compared to the point-estimate network baseline averaged across all target classes.
更多查看译文
关键词
variational Bayesian deep learning,uncertainty propagation,adaptive label smoothing,epistemic uncertainty,calibrated deep learning,bioacoustics,wildlife call detection,passive acoustic monitoring,machine audition
AI 理解论文
溯源树
样例
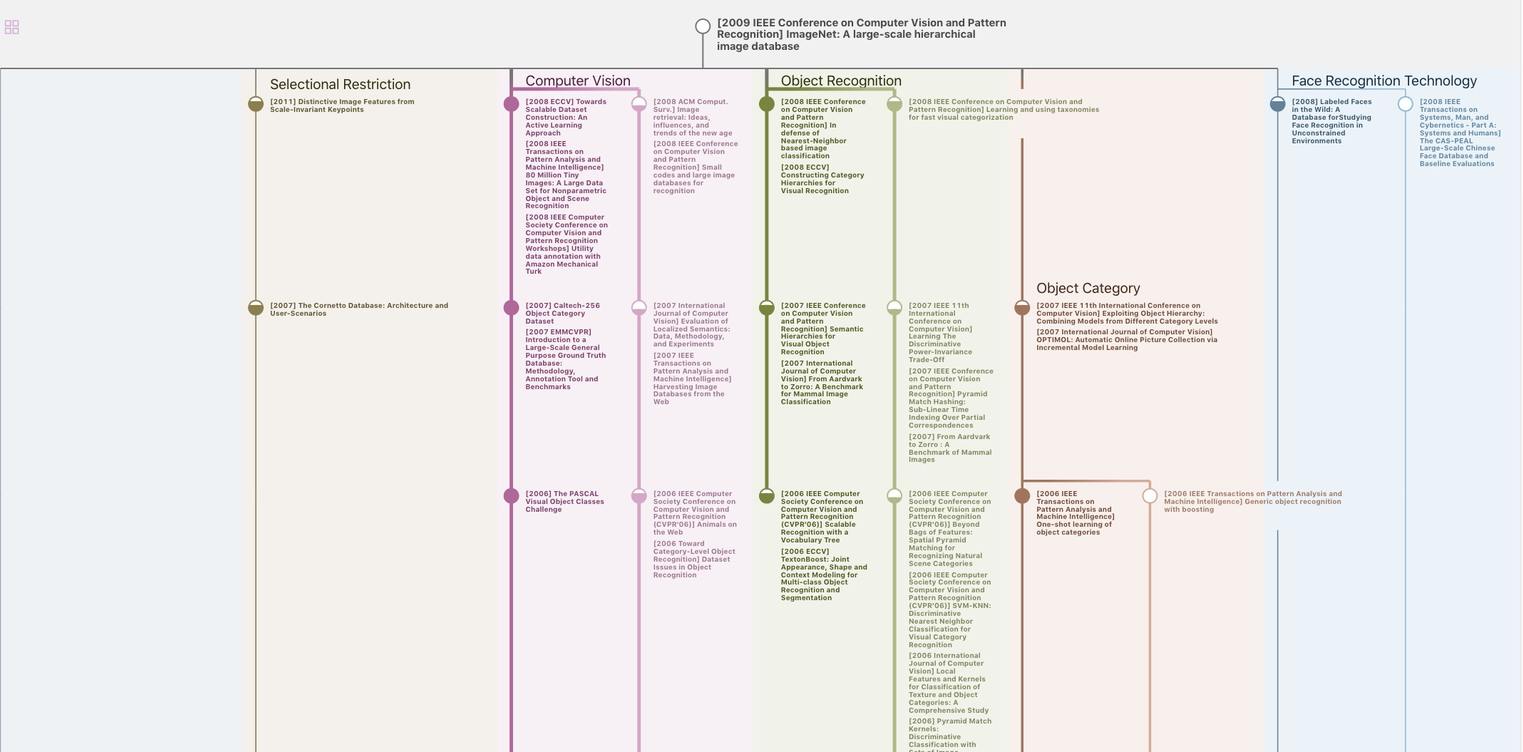
生成溯源树,研究论文发展脉络
Chat Paper
正在生成论文摘要