LightEA: A Scalable, Robust, and Interpretable Entity Alignment Framework via Three-view Label Propagation
emnlp 2022(2022)
摘要
Entity Alignment (EA) aims to find equivalent entity pairs between KGs, which is the core step of bridging and integrating multi-source KGs. In this paper, we argue that existing GNN-based EA methods inherit the inborn defects from their neural network lineage: weak scalability and poor interpretability. Inspired by recent studies, we reinvent the Label Propagation algorithm to effectively run on KGs and propose a non-neural EA framework -- LightEA, consisting of three efficient components: (i) Random Orthogonal Label Generation, (ii) Three-view Label Propagation, and (iii) Sparse Sinkhorn Iteration. According to the extensive experiments on public datasets, LightEA has impressive scalability, robustness, and interpretability. With a mere tenth of time consumption, LightEA achieves comparable results to state-of-the-art methods across all datasets and even surpasses them on many.
更多查看译文
关键词
interpretable entity alignment
AI 理解论文
溯源树
样例
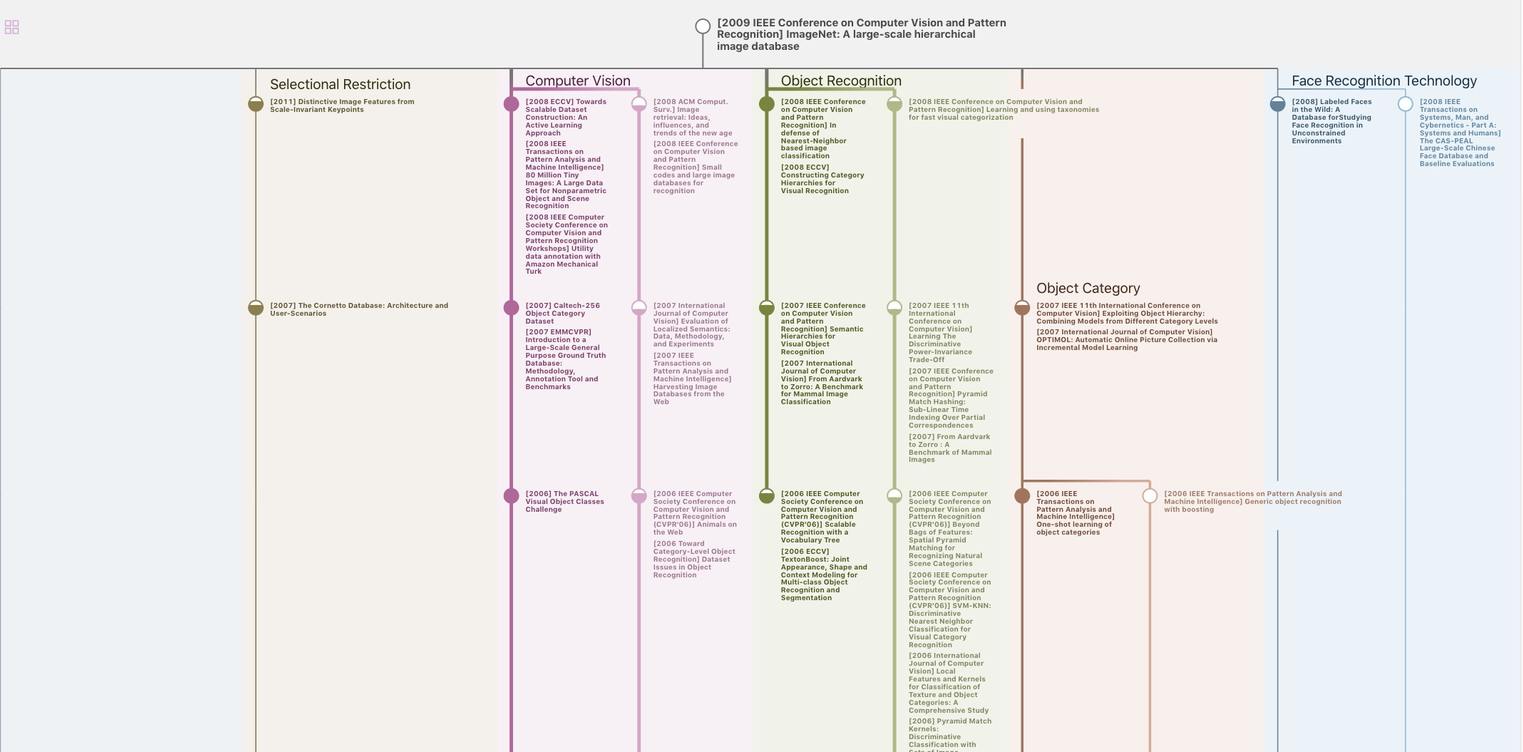
生成溯源树,研究论文发展脉络
Chat Paper
正在生成论文摘要