Multi-hazard risk analysis for the U.S. department of the interior: An integration of expert elicitation, planning priorities, and geospatial analysis
International Journal of Disaster Risk Reduction(2022)
摘要
An integral part of disaster risk management is identifying and prioritizing hazards and their potential impacts in a meaningful way to support risk-reduction planning. There has been considerable use and subsequent criticism of threat prioritization efforts that simply compare likelihoods and consequences of plausible threats. This article summarizes a new mixed-methods and scalable approach for prioritizing risks in a multi-hazard, multi-objective, and multi-criteria organizational context. This approach integrates (1) hazard characterizations using subject-matter-expert (SME) elicitation, (2) expressed preferences in planning priorities provided by emergency managers, and (3) quantitative estimates of asset exposure to hazards using geospatial data and geographic-information-systems (GIS) software. We demonstrate this approach with a case study designed to support multi-hazard mitigation and response planning done by the U.S. Department of the Interior (DOI) Office of Emergency Management, which required a national understanding of the risks posed by 75 natural, technological, and adversarial hazards to DOI managed and administered lands, facilities, people, revenues, and resources. Results demonstrate that hazard priorities vary depending on the asset, scale, and risk-management context, thereby making the case that “one-size-fits-all” hazard rankings have limited utility or relevance to real-world, risk mitigation and response planning. Our results suggest that recognizing the risk-management context provides greater transparency, flexibility, and relevance in comparing threats than traditional likelihood-threat matrices or the use of hazard SMEs to decide for planners which hazard scenarios are emphasized in risk planning.
更多查看译文
关键词
Risk,Prioritization,Hazard,Assessment,Emergency management
AI 理解论文
溯源树
样例
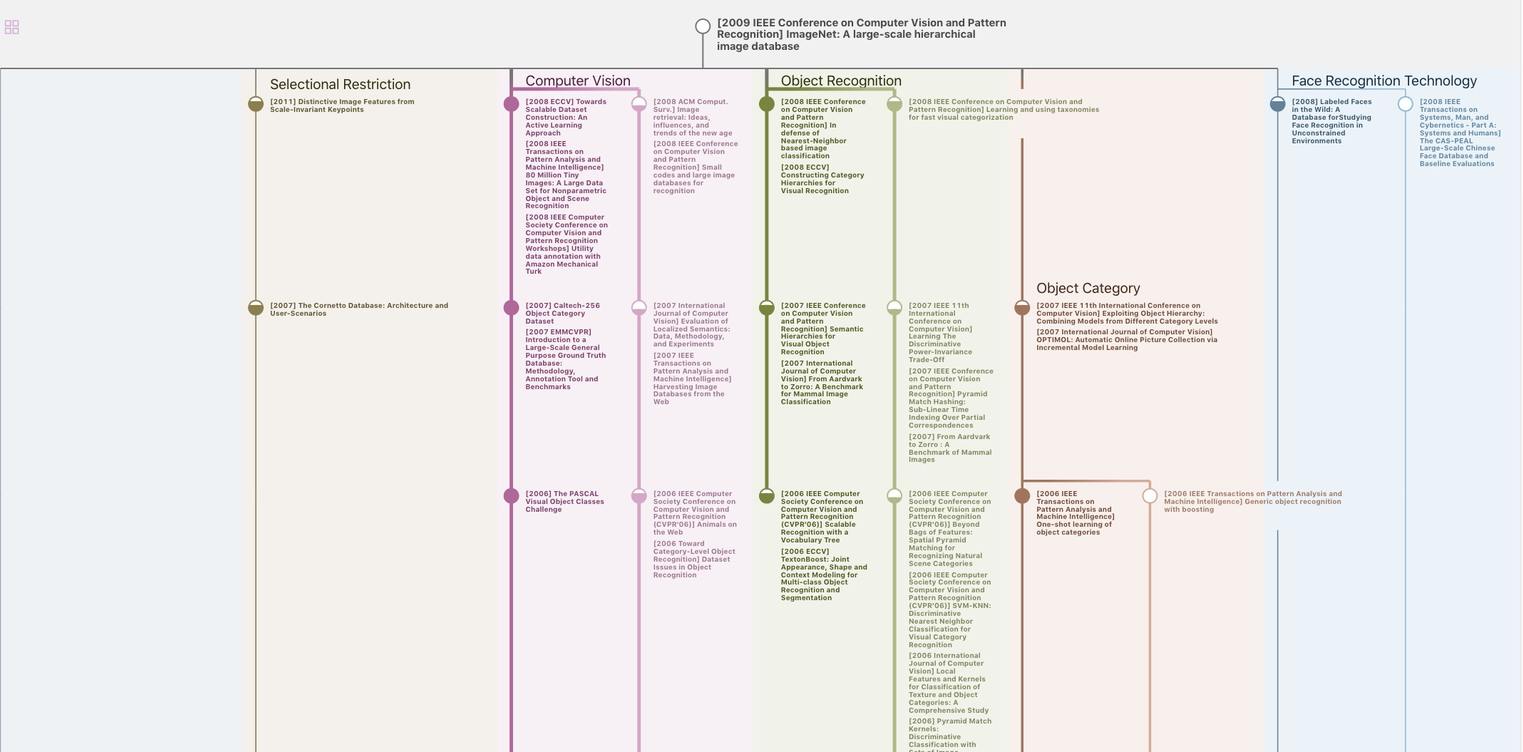
生成溯源树,研究论文发展脉络
Chat Paper
正在生成论文摘要