XAI & I: Self-explanatory AI facilitating mutual understanding between AI and human experts
Procedia Computer Science(2022)
Abstract
Traditionally, explainable artificial intelligence seeks to provide explanation and interpretability of high-performing black-box models such as deep neural networks. Interpretation of such models remains difficult, because of their high complexity. An alternative method is to instead force a deep-neural network to use human-intelligible features as the basis for its decisions. We tested this approach using the natural category domain of rock types. We compared the performance of a black-box implementation of transfer-learning using Resnet50 to that of a network first trained to predict expert-identified features and then forced to use these features to categorise rock images. The performance of this feature-constrained network was virtually identical to that of the unconstrained network. Further, a partially constrained network forced to condense down to a small number of features that was not trained with expert features did not result in these abstracted features being intelligible; nevertheless, an affine transformation of these features could be found that aligned well with expert-intelligible features. These findings show that making an AI intrinsically intelligible need not be at the cost of performance.
MoreTranslated text
Key words
Self-explanatory AI,Deep neural networks,Transfer learning,XAI,Category learning
AI Read Science
Must-Reading Tree
Example
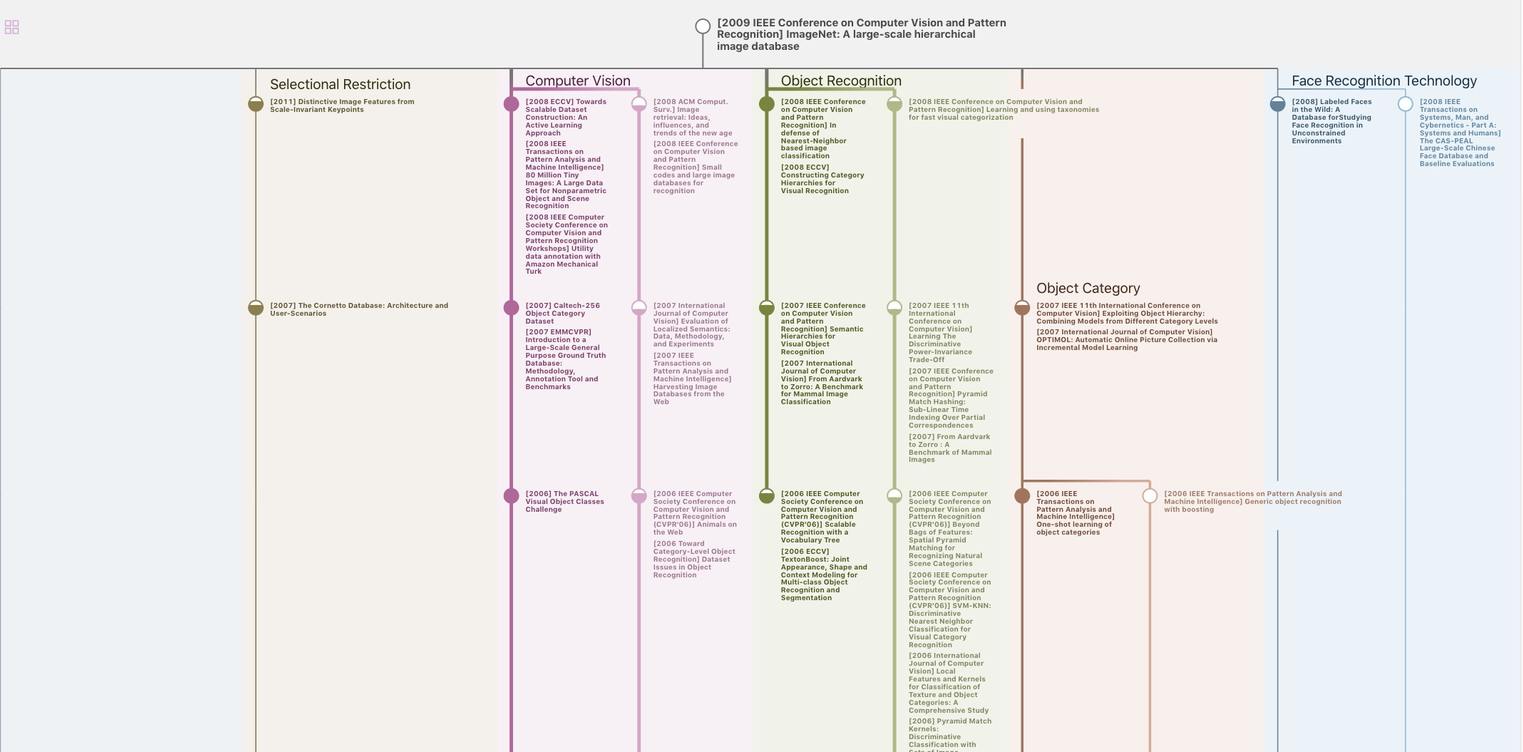
Generate MRT to find the research sequence of this paper
Chat Paper
Summary is being generated by the instructions you defined