Molecular index modulation using convolutional neural networks
Nano Communication Networks(2022)
摘要
As the potential of molecular communication via diffusion (MCvD) systems at nano-scale communication increases, designing molecular schemes robust to the inevitable effects of molecular interference has become of vital importance. There are numerous molecular approaches in literature aiming to mitigate the effects of interference, namely inter-symbol interference. Moreover, for molecular multiple-input–multiple-output systems, interference among antennas, namely inter-link interference, becomes of significance. Inspired by the state-of-the-art performances of machine learning algorithms on making decisions, we propose a novel approach of a convolutional neural network (CNN)-based architecture. The proposed approach is for a uniquely-designed molecular multiple-input–single-output topology in order to alleviate the damaging effects of molecular interference. In this study, we compare the performance of the proposed network with that of an index modulation approach and a symbol-by-symbol maximum likelihood estimation and show that the proposed method yields better performance.
更多查看译文
关键词
Molecular communications,Multiple-input–single-output systems,Index modulation,Machine learning
AI 理解论文
溯源树
样例
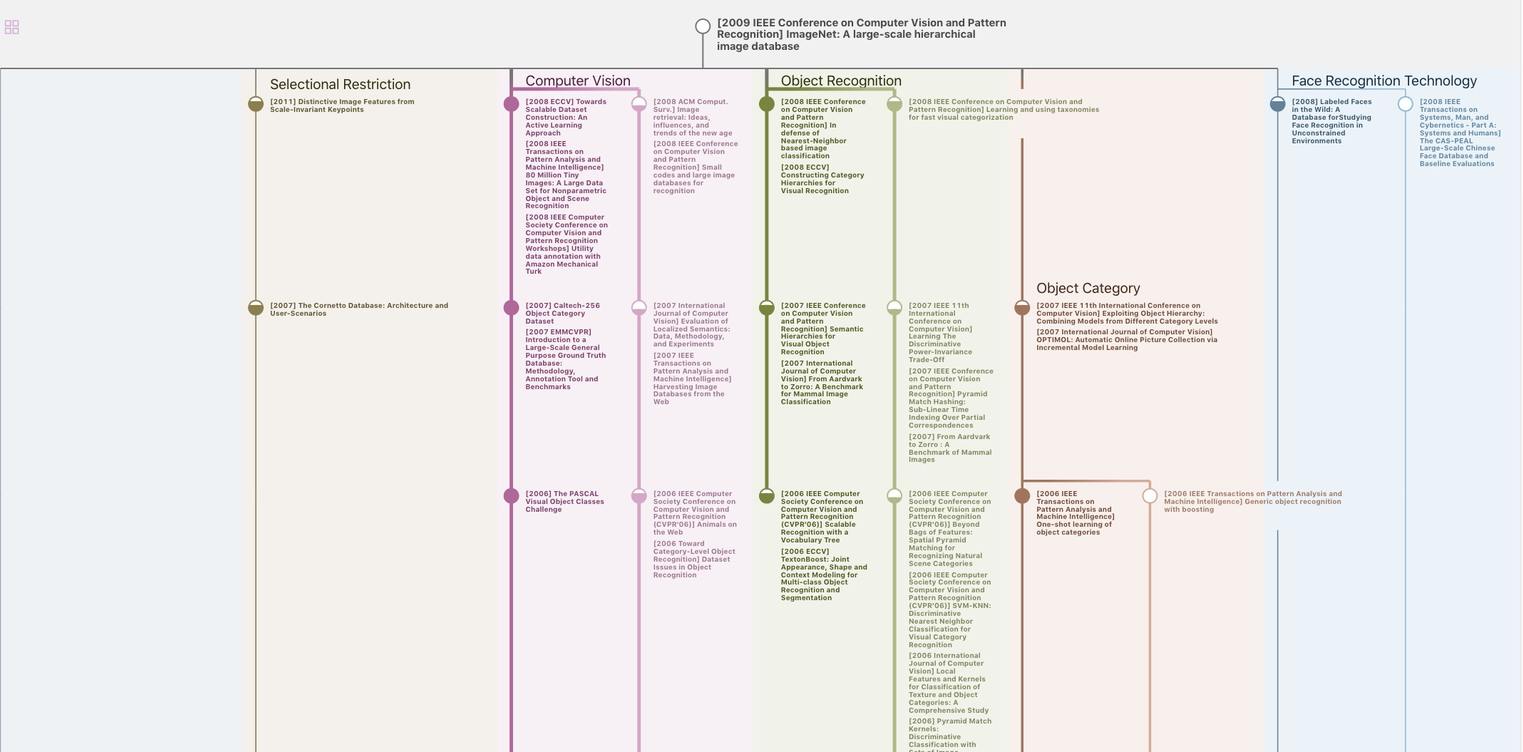
生成溯源树,研究论文发展脉络
Chat Paper
正在生成论文摘要