Out-of-Distribution Identification: Let Detector Tell Which I Am Not Sure.
European Conference on Computer Vision(2022)
摘要
The superior performance of object detectors is often established under the condition that the test samples are in the same distribution as the training data. However, in most practical applications, out-of-distribution (OOD) instances are inevitable and usually lead to detection uncertainty. In this work, the Feature structured OOD-IDentification (FOOD-ID) model is proposed to reduce the uncertainty of detection results by identifying the OOD instances. Instead of outputting each detection result directly, FOOD-ID uses a likelihood-based measuring mechanism to identify whether the feature satisfies the corresponding class distribution and outputs the OOD results separately. Specifically, the clustering-oriented feature structuration is firstly developed using class-specified prototypes and Attractive-Repulsive loss for more discriminative feature representation and more compact distribution. With the structured features space, the density distribution of all training categories is estimated based on a class-conditional normalizing flow, which is then used for the OOD identification in the test stage. The proposed FOOD-ID can be easily applied to various object detectors including anchor-based frameworks and anchor-free frameworks. Extensive experiments on the PASCAL VOC-IO dataset and an industrial defect dataset demonstrate that FOOD-ID achieves satisfactory OOD identification performance, with which the certainty of detection results is improved significantly.
更多查看译文
关键词
Out-of-distribution,Identification,Object detection
AI 理解论文
溯源树
样例
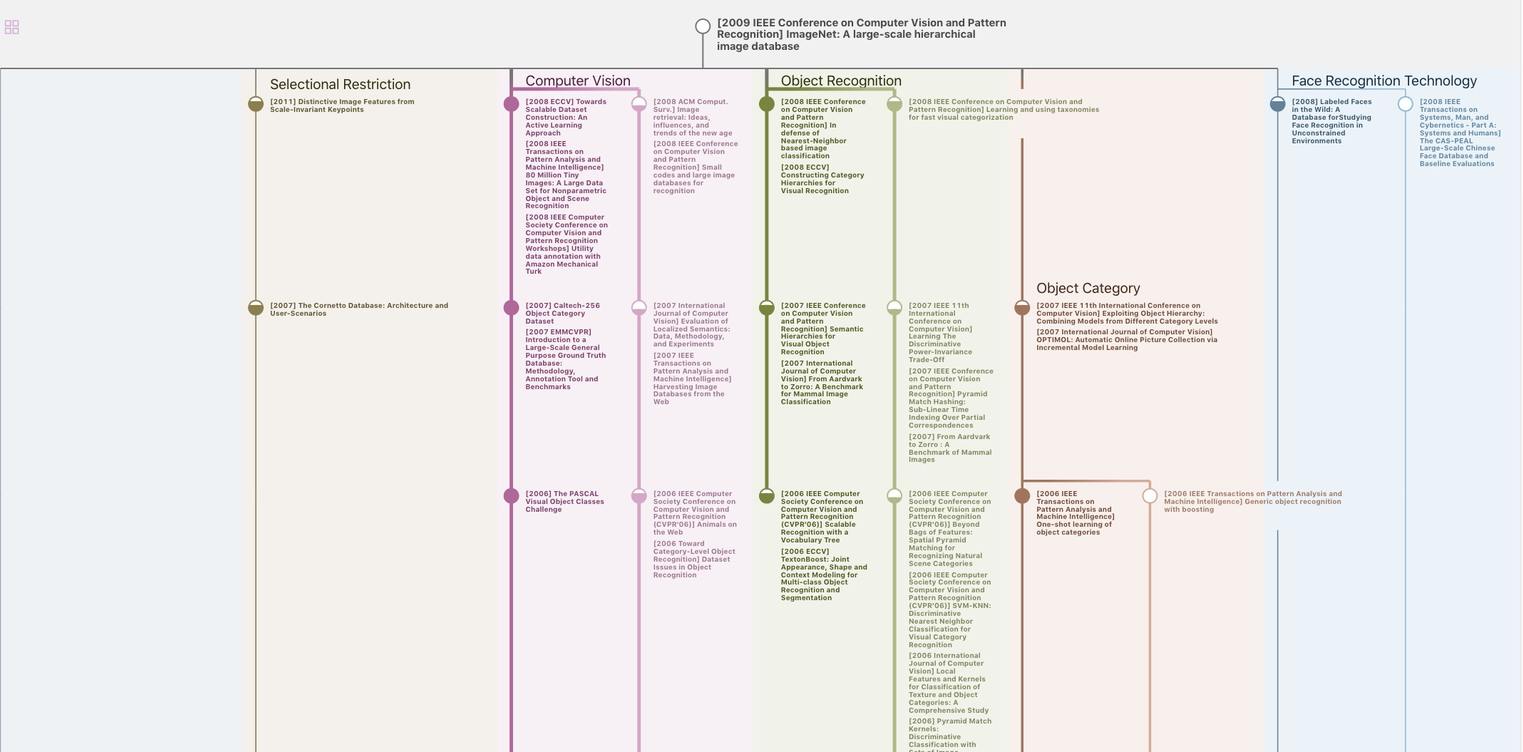
生成溯源树,研究论文发展脉络
Chat Paper
正在生成论文摘要