CoVisPose: Co-visibility Pose Transformer for Wide-Baseline Relative Pose Estimation in 360 degrees Indoor Panoramas
European Conference on Computer Vision(2022)
Abstract
We present CoVisPose, a new end-to-end supervised learning method for relative camera pose estimation in wide baseline 360 degrees indoor panoramas. To address the challenges of occlusion, perspective changes, and textureless or repetitive regions, we generate rich representations for direct pose regression by jointly learning dense bidirectional visual overlap, correspondence, and layout geometry. We estimate three image column-wise quantities: co-visibility (the probability that a given column's image content is seen in the other panorama), angular correspondence (angular matching of columns across panoramas), and floor layout (the vertical floor-wall boundary angle). We learn these dense outputs by applying a transformer over the image-column feature sequences, which cover the full 360 degrees field-of-view (FoV) from both panoramas. The resultant rich representation supports learning robust relative poses with an efficient 1D convolutional decoder. In addition to learned direct pose regression with scale, our network also supports pose estimation through a RANSAC-based rigid registration of the predicted corresponding layout boundary points. Our method is robust to extremely wide baselines with very low visual overlap, as well as significant occlusions. We improve upon the SOTA by a large margin, as demonstrated on a large-scale dataset of real homes, ZInD.
MoreTranslated text
Key words
Indoor,360 degrees Panorama,Indoor,Pose estimation,Camera localization,Structure-from-motion,Layout
AI Read Science
Must-Reading Tree
Example
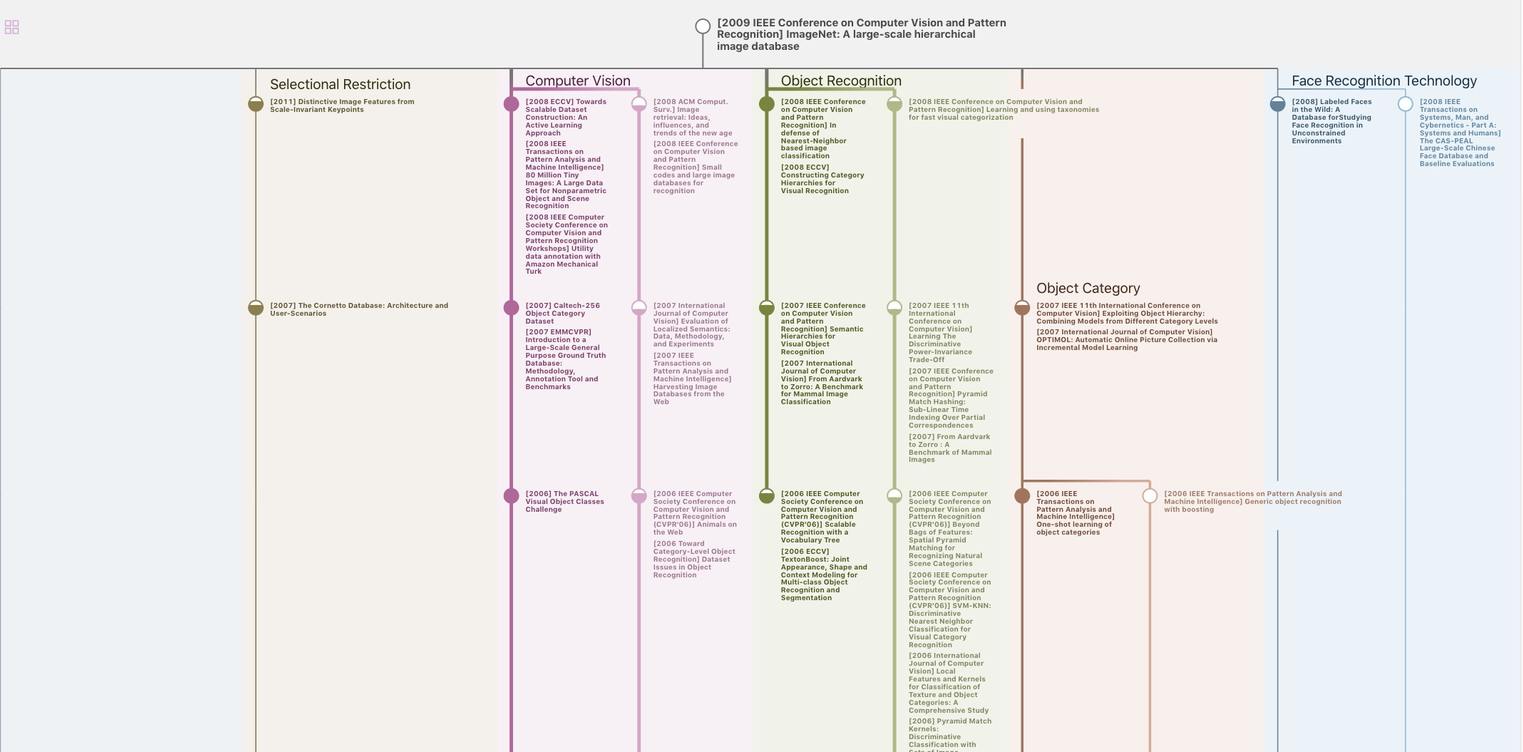
Generate MRT to find the research sequence of this paper
Chat Paper
Summary is being generated by the instructions you defined