YOLOv5-GE Vehicle Detection Algorithm Integrating Global Attention Mechanism
2022 3rd International Conference on Information Science, Parallel and Distributed Systems (ISPDS)(2022)
关键词
YOLOv5-GE algorithm,global attention mechanism,Loss function,styling,vehicle detection
AI 理解论文
溯源树
样例
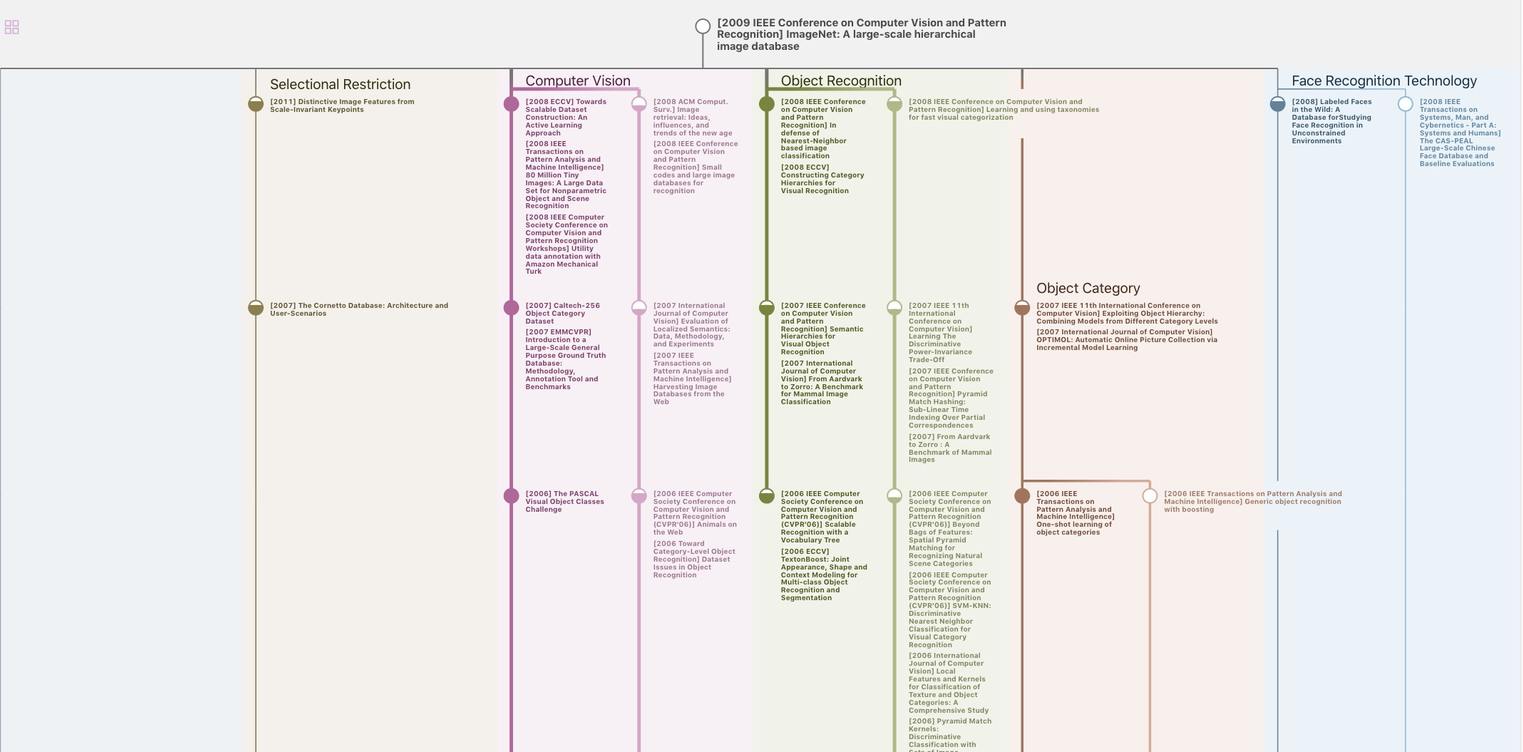
生成溯源树,研究论文发展脉络
Chat Paper
正在生成论文摘要