Correlation Structured Low-Rank Subspace Clustering
2020 IEEE 4th Information Technology, Networking, Electronic and Automation Control Conference (ITNEC)(2020)
摘要
High-dimensional data is usually drawn from the union of multiple low-dimensional subspaces. The task of subspace clustering is to cluster high-dimensional data into their corresponding subspace. Subspace clustering algorithm based on spectral clustering is one of the important methods of subspace clustering. This method is proposed by the spectral clustering framework. This type of method is usually divided into two steps, namely learning the affinity matrix and obtaining the segmentation matrix by spectral clustering. However, the results obtained this way are often sub-optimal. Meanwhile many state-of-the-art algorithms do not consider consistency of correlation data, resulting in the affinity matrix either too dense to make the algorithm contain a lot of erroneous information, or too sparse to make the algorithm lose correlation information. In this paper, we propose Correlation Structured Low-Rank Subspace Clustering (CSLRSC). The CSLRSC is based on the model of low rank representation by adding new
$l_{1}$
norm to solve the problem that the affinity matrix established by the previous method is too dense. Adding the structured norm to the model can integrate affinity matrix learning and spectral clustering into one step to obtain an approximate optimal solution of the algorithm. The experimental results that we obtain on Extended Yale B face dataset, MINIST hand-write dataset, and ORL dataset show that our algorithm is superior to others.
更多查看译文
关键词
Low-Rank representation,subspace clustering,correlation data,spectral clustering,consistency
AI 理解论文
溯源树
样例
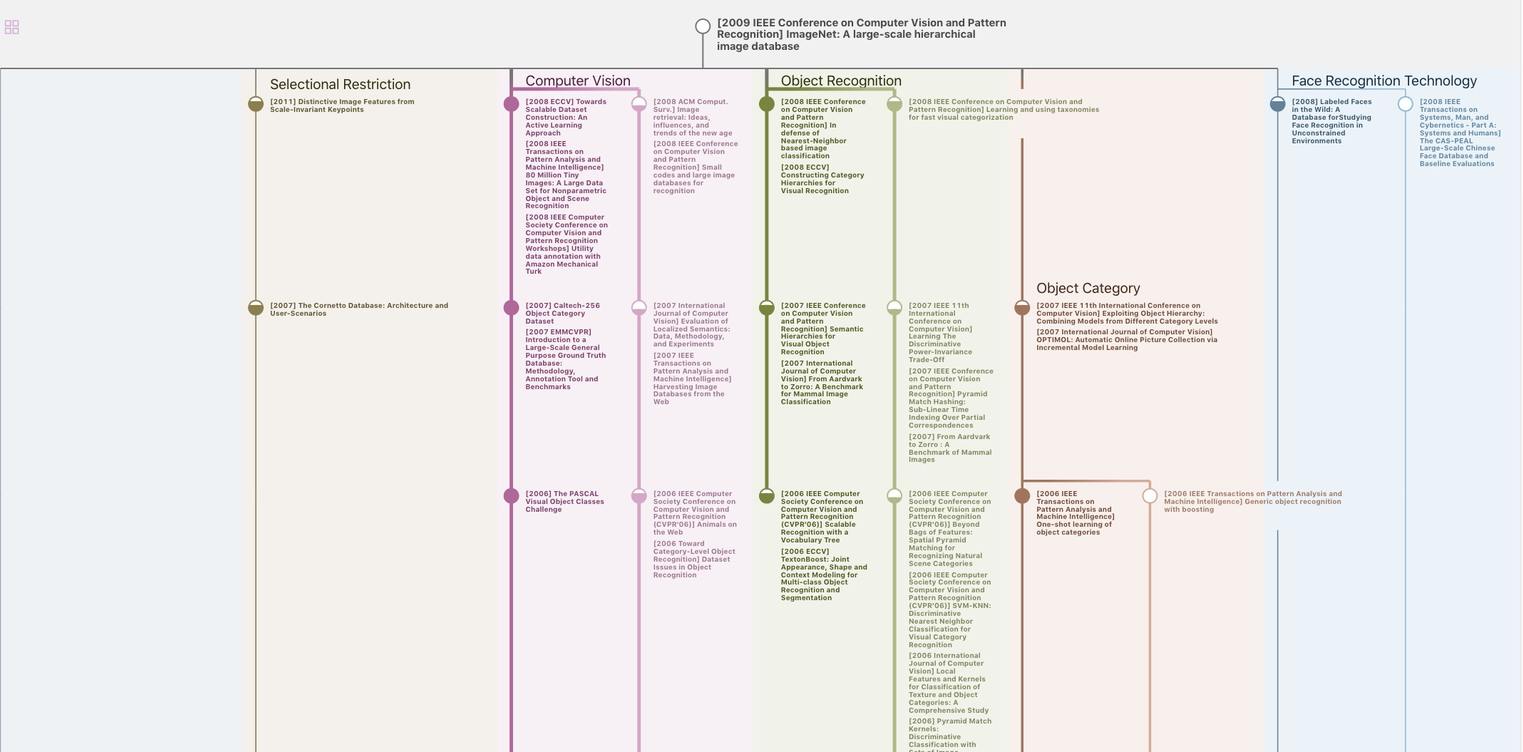
生成溯源树,研究论文发展脉络
Chat Paper
正在生成论文摘要