No Pairs Left Behind: Improving Metric Learning with Regularized Triplet Objective
CoRR(2022)
Abstract
We propose a novel formulation of the triplet objective function that improves metric learning without additional sample mining or overhead costs. Our approach aims to explicitly regularize the distance between the positive and negative samples in a triplet with respect to the anchor-negative distance. As an initial validation, we show that our method (called No Pairs Left Behind [NPLB]) improves upon the traditional and current state-of-the-art triplet objective formulations on standard benchmark datasets. To show the effectiveness and potentials of NPLB on real-world complex data, we evaluate our approach on a large-scale healthcare dataset (UK Biobank), demonstrating that the embeddings learned by our model significantly outperform all other current representations on tested downstream tasks. Additionally, we provide a new model-agnostic single-time health risk definition that, when used in tandem with the learned representations, achieves the most accurate prediction of subjects' future health complications. Our results indicate that NPLB is a simple, yet effective framework for improving existing deep metric learning models, showcasing the potential implications of metric learning in more complex applications, especially in the biological and healthcare domains.
MoreTranslated text
Key words
Deep Metric Learning,Representation Learning,Machine Learning for Healthcare,Triplet Loss
AI Read Science
Must-Reading Tree
Example
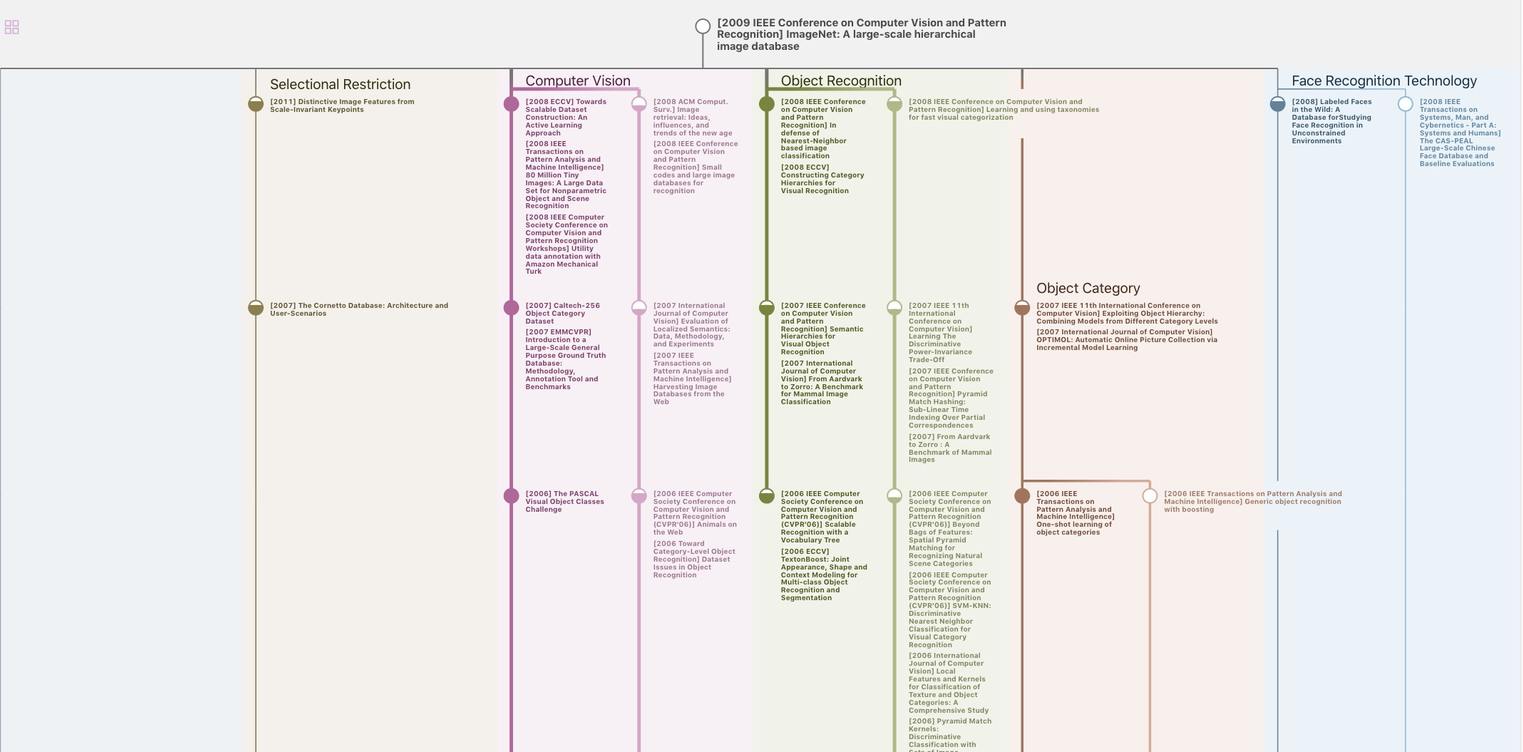
Generate MRT to find the research sequence of this paper
Chat Paper
Summary is being generated by the instructions you defined