Probabilistic Categorical Adversarial Attack & Adversarial Training
arxiv(2022)
摘要
The existence of adversarial examples brings huge concern for people to apply Deep Neural Networks (DNNs) in safety-critical tasks. However, how to generate adversarial examples with categorical data is an important problem but lack of extensive exploration. Previously established methods leverage greedy search method, which can be very time-consuming to conduct successful attack. This also limits the development of adversarial training and potential defenses for categorical data. To tackle this problem, we propose Probabilistic Categorical Adversarial Attack (PCAA), which transfers the discrete optimization problem to a continuous problem that can be solved efficiently by Projected Gradient Descent. In our paper, we theoretically analyze its optimality and time complexity to demonstrate its significant advantage over current greedy based attacks. Moreover, based on our attack, we propose an efficient adversarial training framework. Through a comprehensive empirical study, we justify the effectiveness of our proposed attack and defense algorithms.
更多查看译文
关键词
adversarial attacks,robustness,discrete input model
AI 理解论文
溯源树
样例
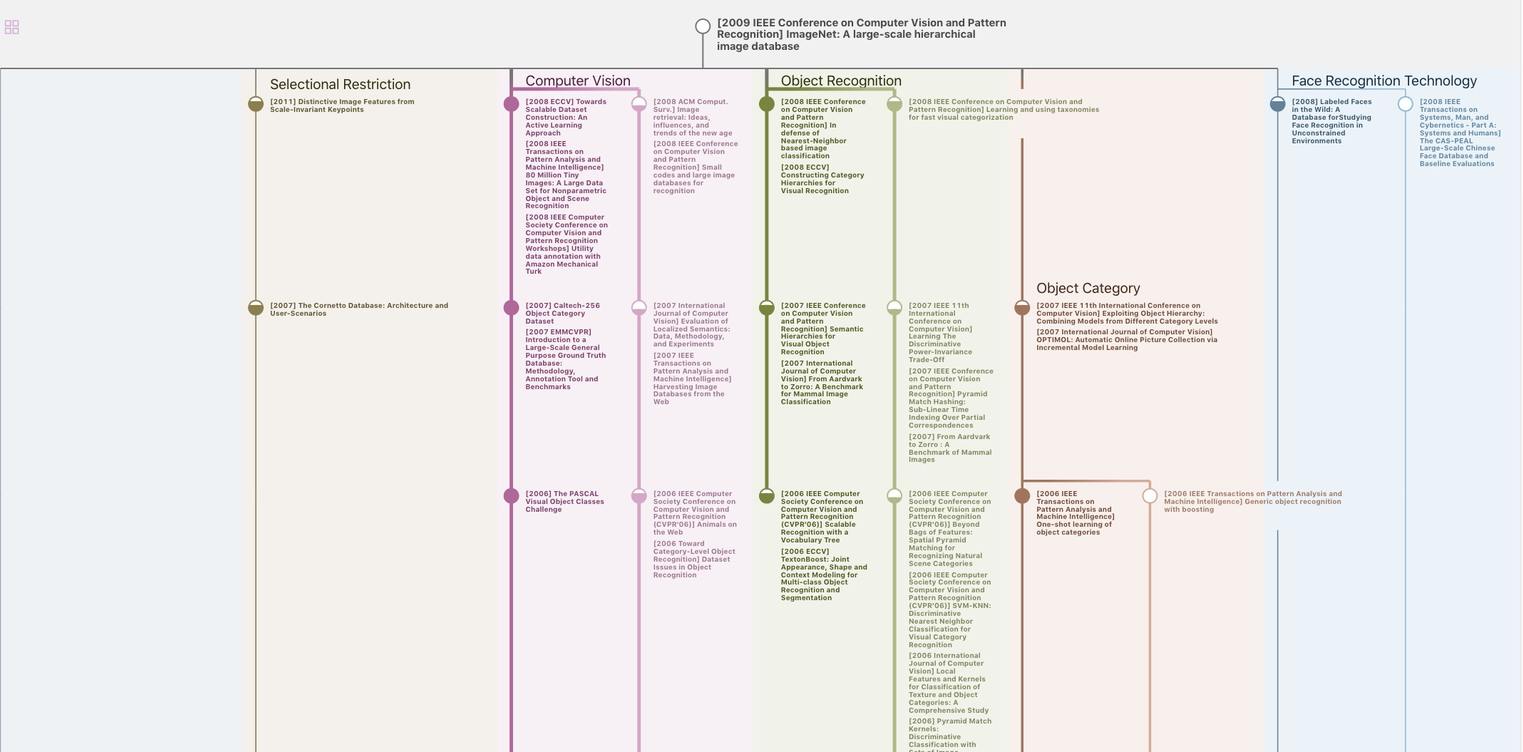
生成溯源树,研究论文发展脉络
Chat Paper
正在生成论文摘要