Acceleration of Neural Network Training on Hardware via HLS for an Edge-AI Device
2020 Innovations in Intelligent Systems and Applications Conference (ASYU)(2020)
摘要
The neural network has two computational steps: inference and training. In recent years, high-performance inference processing is accelerated by AI-specific hardware accelerators. However, training phase, which is the most important part of the computational load of a neural network, remains a difficult problem to solve in low-power edge devices. From a design productivity perspective, designing efficient hardware accelerator for training phase at RTL level is a time-consuming process due to cumbersome of the low-level hardware description languages. Therefore, we propose a high-level description (C) of the training algorithm: gradient descent algorithm using high-level synthesis-Vivado HLS, to generate a computationally efficient accelerator. Empirical results show that the resource constrained edge devices can also perform the training part while they are also inferring. In this study, we propose a system-on-chip solution to accelerate neural network training workloads for an edge-AI device.
更多查看译文
关键词
High-level synthesis,system-on-chip (SoC),FPGA,Edge-AI device
AI 理解论文
溯源树
样例
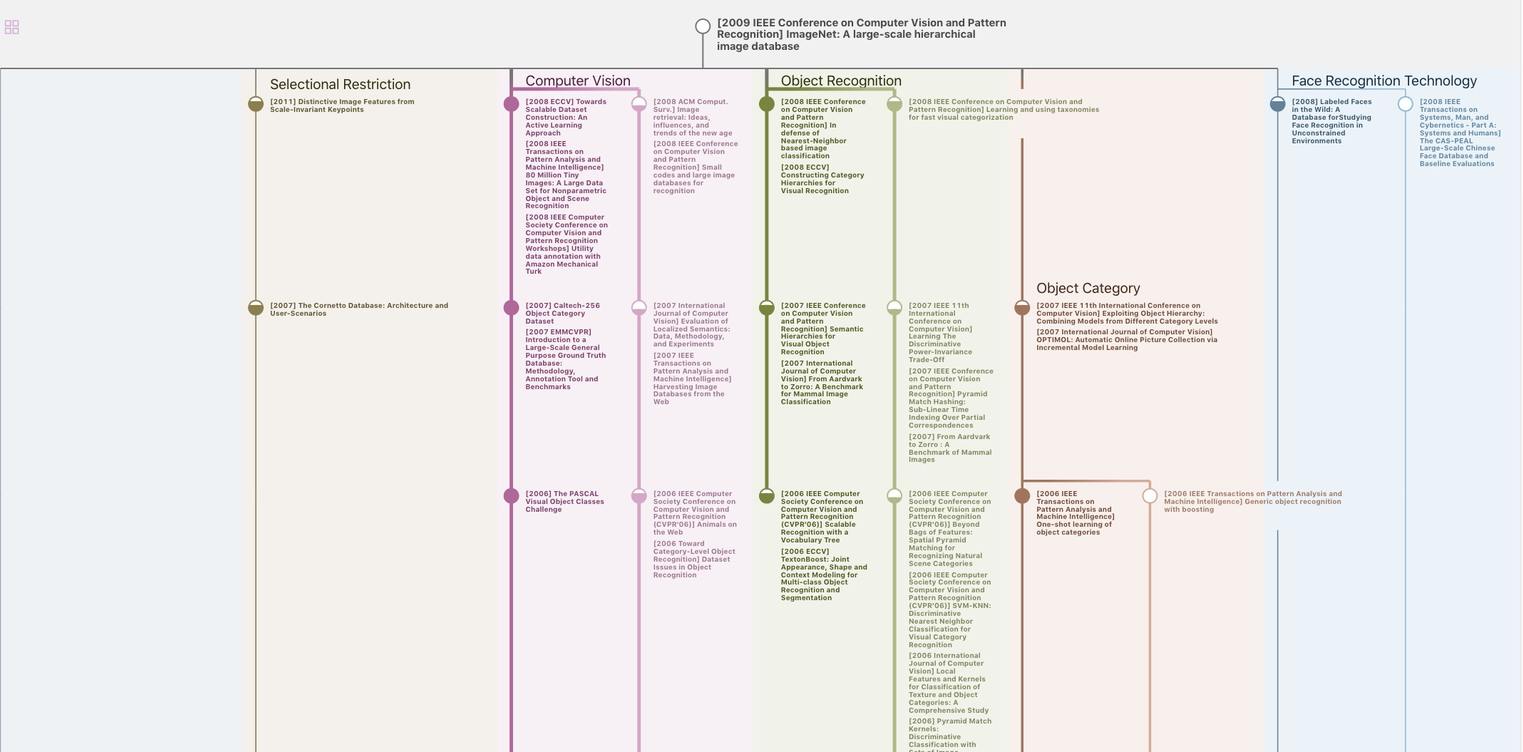
生成溯源树,研究论文发展脉络
Chat Paper
正在生成论文摘要