Convolutional Neural Network Aided Chemical Species Tomography for Dynamic Temperature Imaging
2022 IEEE International Instrumentation and Measurement Technology Conference (I2MTC)(2022)
摘要
Chemical Species Tomography (CST) using Tunable Diode Laser Absorption Spectroscopy (TDLAS) is an in-situ technique to reconstruct the two-dimensional temperature distributions in combustion diagnosis. However, limited by the lack of projection data, traditionally computational tomographic algorithms are inherently rank-deficient, causing artefacts and severe uncertainty in the retrieved images. Recently, data-driven approaches, such as deep learning algorithms, have been validated to be more accurate and stable for CST. However, most attempts modelled the phantoms using two-dimensional Gaussian profiles to construct the training set, enabling reconstruction of only simple and static temperature fields and can seldom retrieve the dynamic and instantaneous temperature imaging. To address this problem, we use Fire Dynamics Simulator (FDS) to simulate the dynamic and fire-driven reacting flows for training set construction. Based on this training set, a Convolutional Neural Network (CNN) is designed. This newly introduced method is validated by numerical simulation, indicating good accuracy and sensitivity in monitoring dynamic flames.
更多查看译文
关键词
Dynamic,temperature imaging,Convolutional Neural Network (CNN),Tunable Diode Laser Absorption Spectroscopy (TDLAS),Chemical Species Tomography
AI 理解论文
溯源树
样例
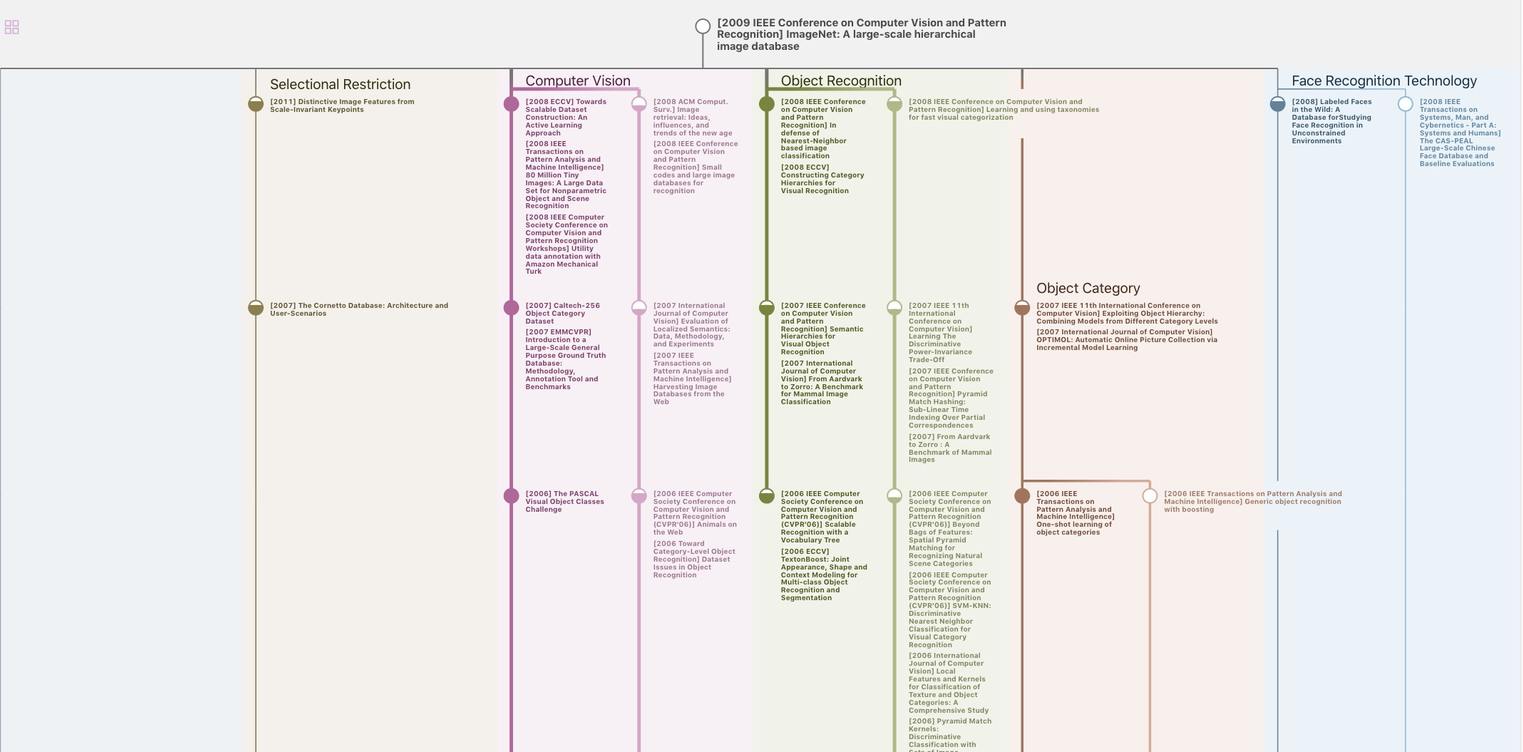
生成溯源树,研究论文发展脉络
Chat Paper
正在生成论文摘要