Performance enhancement of CAP-VLC system utilizing GRU neural network based equalizer
Optics Communications(2022)
Abstract
In this paper, a gated recurrent unit (GRU) neural network based equalizer is proposed for the first time to compensate for linear and nonlinear distortions in carrier-less amplitude phase (CAP) band-limited visible light communication (VLC) systems. The equalization scheme is mainly based on the GRU algorithm, which captures the dependencies between sequences within the memory size through a gating mechanism. Moreover, we experimentally compare the performance of traditional finite impulse response (FIR) based equalizer using real-valued GRU neural network (RV-GRUNN) with and without memory as well as separate complex-valued GRU neural network (SCV-GRUNN) with and without memory. Compared with the memoryless networks, the networks with memory improve the convergence speed and fitting accuracy at the expense of complexity. Compared with real-valued networks, the separate complex-valued networks have lower complexity and better generalization ability. By deploying SCV-GRUNN (M=3), a 1.8-m 560 Mb/s CAP128 VLC system is successfully demonstrated with Q factor improvement of 3.81 dB, 2.92 dB, 1.42 dB and 1.05 dB over the second-order Volterra Series, RV-GRUNN(M=1), SCV-GRUNN (M=1) and RV-GRUNN (M=3), respectively.
MoreTranslated text
Key words
Visible light communication (VLC),Post-equalization,Deep learning,Carrier-less amplitude phase (CAP)
AI Read Science
Must-Reading Tree
Example
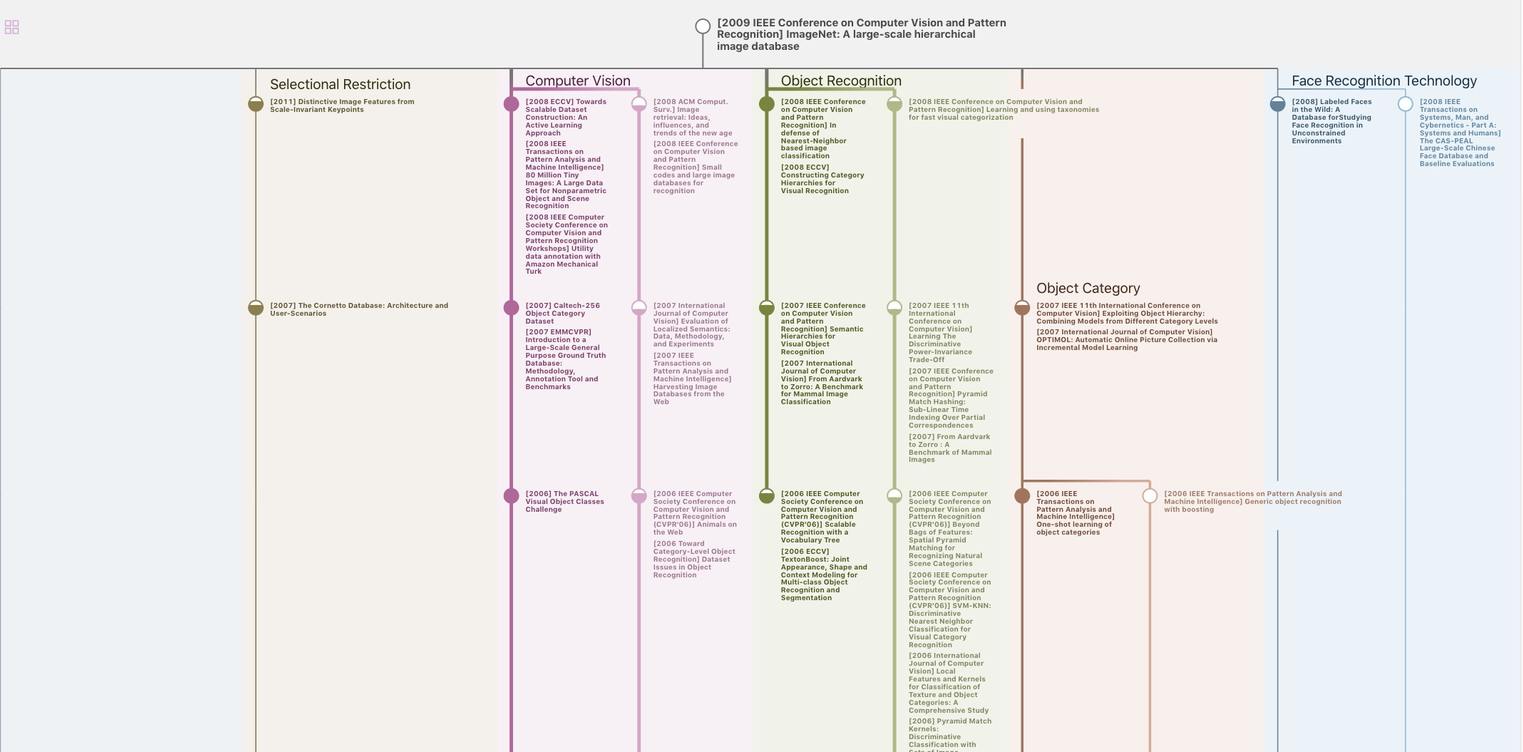
Generate MRT to find the research sequence of this paper
Chat Paper
Summary is being generated by the instructions you defined