Explainability Metrics and Properties for Counterfactual Explanation Methods
EXPLAINABLE AND TRANSPARENT AI AND MULTI-AGENT SYSTEMS, EXTRAAMAS 2022(2022)
摘要
The increasing application of Explainable AI (XAI) methods to enhance the transparency and trustworthiness of AI systems designates the need to quantitatively assess and analyze the theoretical and behavioral characteristics of explanations generated by these methods. A fair amount of metrics and properties exist, however these metrics are method-specific, complex and at times hard to interpret. This work focuses on (i) identification of these metrics and properties applicable to the selected post-hoc counterfactual explanation methods (a mechanism for generating explanations), (ii) assessing the applicability of the identified metrics and properties to compare counterfactual examples across explanation methods, and (iii) analyzing the properties of those counterfactual explanation methods. A pipeline is designed to implement the proof-of-concept tool, comprising of the following steps-selecting a data set, training some suitable classifier, deploying counterfactual generation method(s), and implementing defined XAI metrics to infer properties satisfied by explanation methods. The outcome of the experiments reflects that desirable properties for counterfactual explanations are more or less satisfied as measured by different metrics. Certain inconsistencies were identified in the counterfactual explanation methods such as the resulting counterfactual instances failed to be pushed to the desired class, defeating one of the main purposes of obtaining counterfactual explanations. Besides, several other properties have been discussed to analyze counterfactual explanation methods.
更多查看译文
关键词
Explainable AI, XAI metrics, Counterfactual explanations, Trustworthy AI
AI 理解论文
溯源树
样例
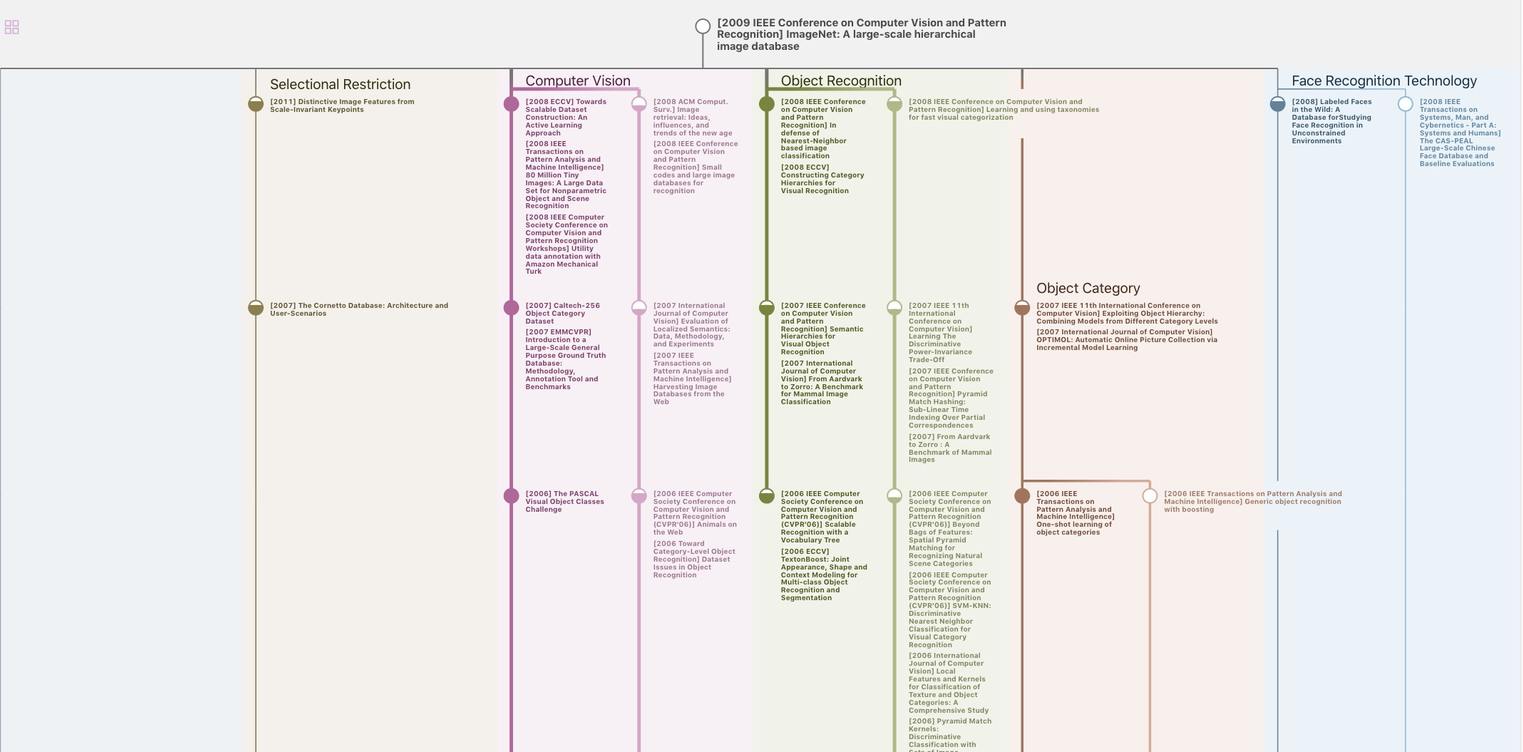
生成溯源树,研究论文发展脉络
Chat Paper
正在生成论文摘要