A Symbolic Representation of Human Posture for Interpretable Learning and Reasoning
CoRR(2022)
Abstract
Robots that interact with humans in a physical space or application need to think about the person's posture, which typically comes from visual sensors like cameras and infra-red. Artificial intelligence and machine learning algorithms use information from these sensors either directly or after some level of symbolic abstraction, and the latter usually partitions the range of observed values to discretize the continuous signal data. Although these representations have been effective in a variety of algorithms with respect to accuracy and task completion, the underlying models are rarely interpretable, which also makes their outputs more difficult to explain to people who request them. Instead of focusing on the possible sensor values that are familiar to a machine, we introduce a qualitative spatial reasoning approach that describes the human posture in terms that are more familiar to people. This paper explores the derivation of our symbolic representation at two levels of detail and its preliminary use as features for interpretable activity recognition.
MoreTranslated text
AI Read Science
Must-Reading Tree
Example
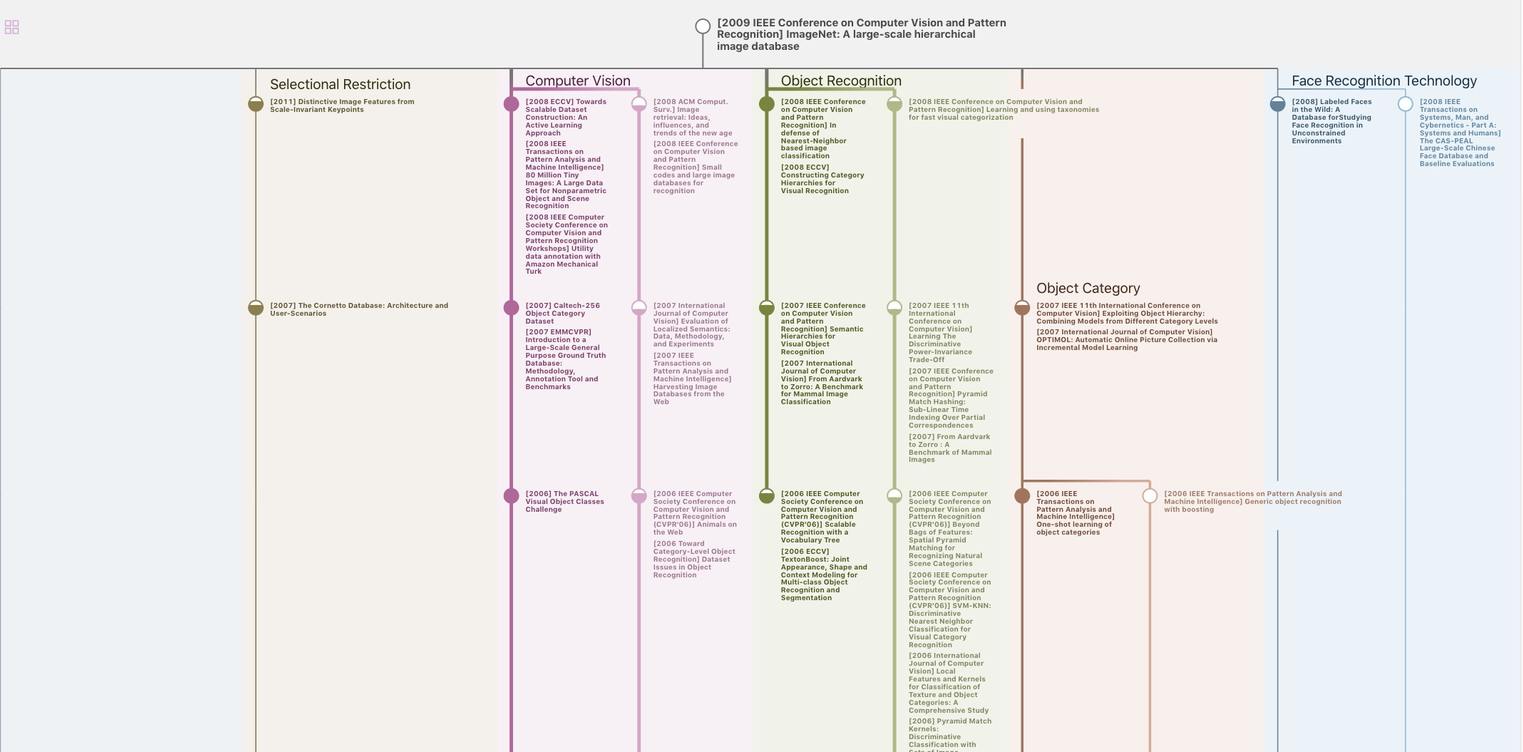
Generate MRT to find the research sequence of this paper
Chat Paper
Summary is being generated by the instructions you defined