Macaw: The Machine Learning Magnetometer Calibration Workflow
arxiv(2022)
摘要
In Earth Systems Science, many complex data pipelines combine different data sources and apply data filtering and analysis steps. Typically, such data analysis processes are historically grown and implemented with many sequentially executed scripts. Scientific workflow management systems (SWMS) allow scientists to use their existing scripts and provide support for parallelization, reusability, monitoring, or failure handling. However, many scientists still rely on their sequentially called scripts and do not profit from the out-of-the-box advantages a SWMS can provide. In this work, we transform the data analysis processes of a Machine Learning-based approach to calibrate the platform magnetometers of non-dedicated satellites utilizing neural networks into a workflow called Macaw (MAgnetometer CAlibration Workflow). We provide details on the workflow and the steps needed to port these scripts to a scientific workflow. Our experimental evaluation compares the original sequential script executions on the original HPC cluster with our workflow implementation on a commodity cluster. Our results show that through porting, our implementation decreased the allocated CPU hours by 50.2% and the memory hours by 59.5%, leading to significantly less resource wastage. Further, through parallelizing single tasks, we reduced the runtime by 17.5%.
更多查看译文
关键词
Geomagnetism, Platform Magnetometer, Scientific Workflow, Workflow Management System, Resource Efficiency
AI 理解论文
溯源树
样例
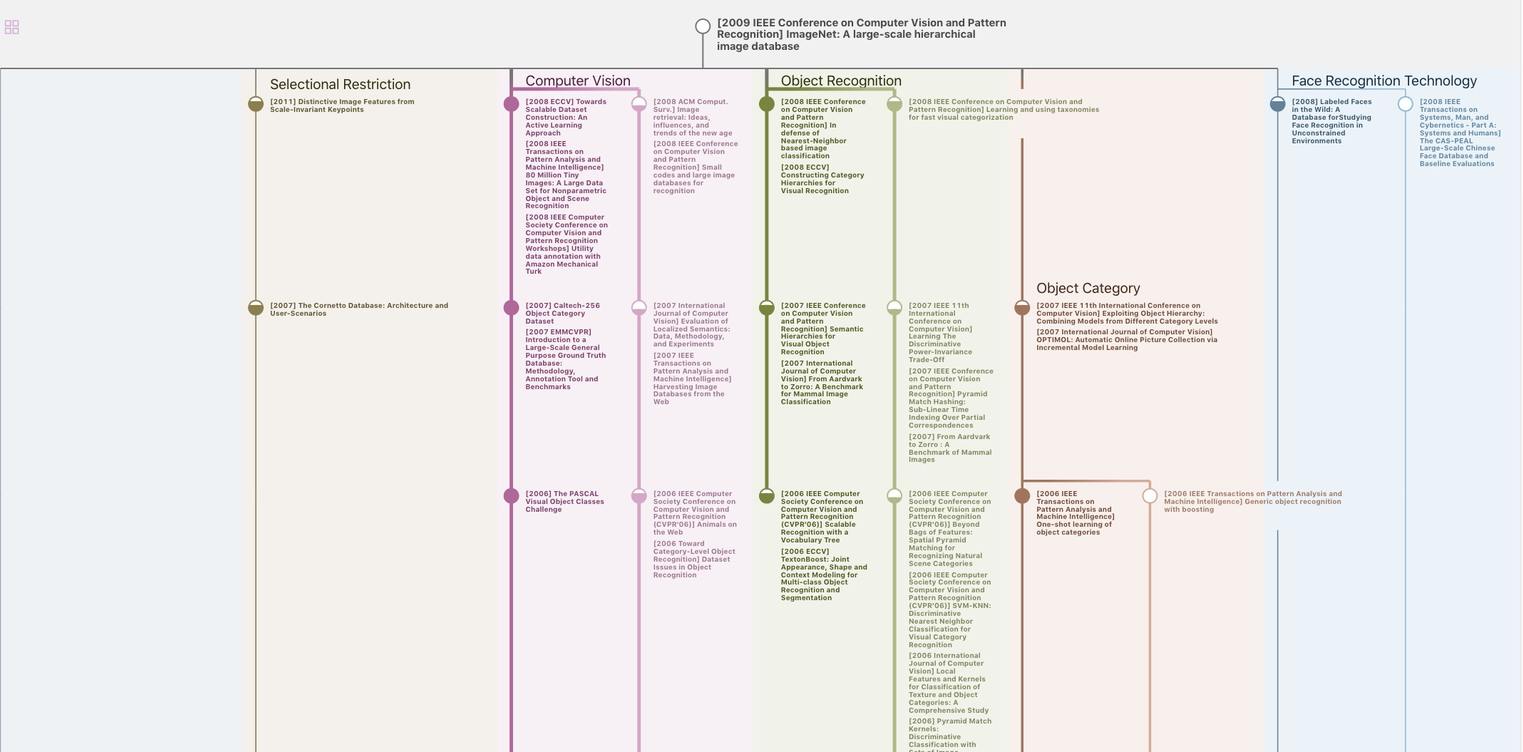
生成溯源树,研究论文发展脉络
Chat Paper
正在生成论文摘要