Zero-Shot Learners for Natural Language Understanding via a Unified Multiple-Choice Perspective
IEEE ACCESS(2023)
摘要
Zero-shot learning is an approach where models generalize to unseen tasks without direct training on them. We introduce the Unified Multiple-Choice (UniMC) framework, which is format-independent, compatible with various formats, and applicable to tasks like text classification and sentiment analysis. Furthermore, we design a two-stage tuning method, initially training on multiple-choice formats to develop format-agnostic capabilities, and subsequently enabling direct predictions on unseen tasks for zero-shot learning. Our methodology avoids issues in large-scale models like FLAN, enhancing generalization and reducing parameters. In experiments, UniMC shows State-of-the-Art (SOTA) performance across out-of-domain and in-domain benchmarks, with only 235M parameters, far fewer than previous methods. Moreover, the UniMC-Chinese model excels beyond human performance on benchmarks like EPRSTMT and CHID-FC, underscoring its generalization capacity across languages. Additionally, ablation experiments demonstrate the effectiveness of our design. The code and model weights are available at https://github.com/IDEA-CCNL/Fengshenbang-LM/tree/main/fengshen/examples/unimc.
更多查看译文
关键词
Multitasking,Multi-task learning,natural language understanding,zero-shot learning
AI 理解论文
溯源树
样例
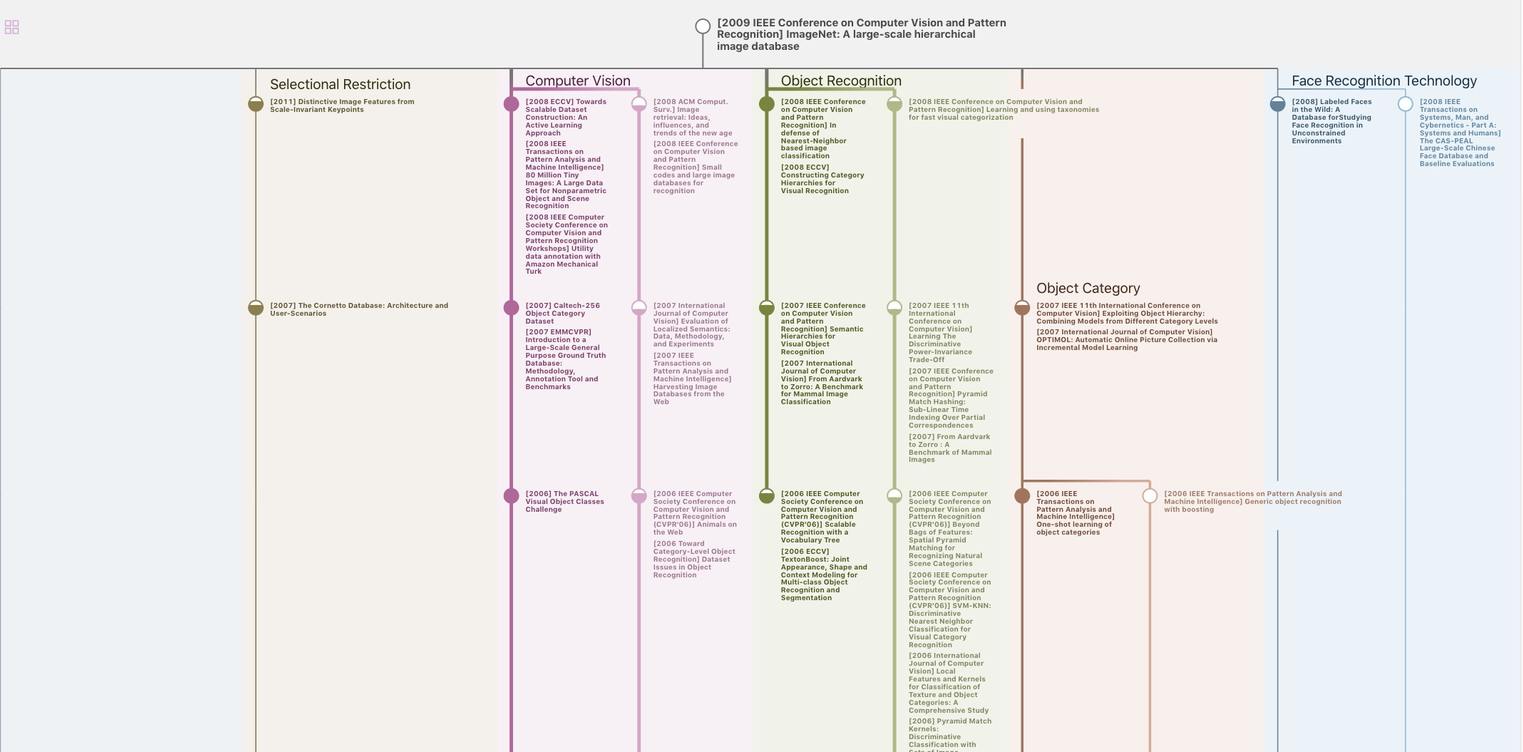
生成溯源树,研究论文发展脉络
Chat Paper
正在生成论文摘要