Improving Semantic Matching through Dependency-Enhanced Pre-trained Model with Adaptive Fusion
arxiv(2022)
摘要
Transformer-based pre-trained models like BERT have achieved great progress on Semantic Sentence Matching. Meanwhile, dependency prior knowledge has also shown general benefits in multiple NLP tasks. However, how to efficiently integrate dependency prior structure into pre-trained models to better model complex semantic matching relations is still unsettled. In this paper, we propose the \textbf{D}ependency-Enhanced \textbf{A}daptive \textbf{F}usion \textbf{A}ttention (\textbf{DAFA}), which explicitly introduces dependency structure into pre-trained models and adaptively fuses it with semantic information. Specifically, \textbf{\emph{(i)}} DAFA first proposes a structure-sensitive paradigm to construct a dependency matrix for calibrating attention weights. It adopts an adaptive fusion module to integrate the obtained dependency information and the original semantic signals. Moreover, DAFA reconstructs the attention calculation flow and provides better interpretability. By applying it on BERT, our method achieves state-of-the-art or competitive performance on 10 public datasets, demonstrating the benefits of adaptively fusing dependency structure in semantic matching task.
更多查看译文
关键词
semantic matching,adaptive fusion,dependency-enhanced,pre-trained
AI 理解论文
溯源树
样例
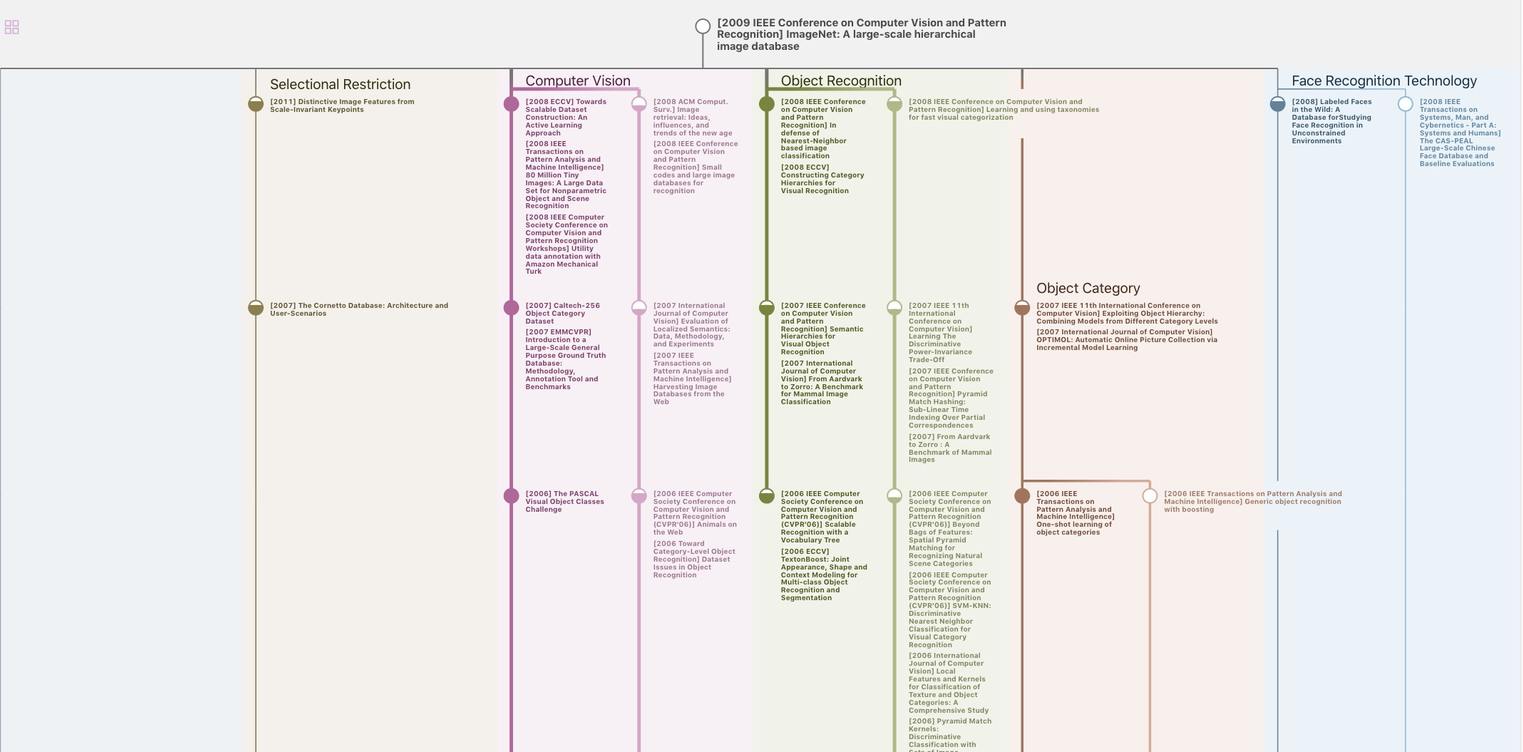
生成溯源树,研究论文发展脉络
Chat Paper
正在生成论文摘要