On Distributionally Robust Multistage Convex Optimization: Data-driven Models and Performance
arxiv(2022)
摘要
This paper presents a novel algorithmic study with extensive numerical experiments of distributionally robust multistage convex optimization (DR-MCO). Following the previous work on dual dynamic programming (DDP) algorithmic framework for DR-MCO [48], we focus on data-driven DR-MCO models with Wasserstein ambiguity sets that allow probability measures with infinite supports. These data-driven Wasserstein DR-MCO models have out-of-sample performance guarantees and adjustable in-sample conservatism. Then by exploiting additional concavity or convexity in the uncertain cost functions, we design exact single stage subproblem oracle (SSSO) implementations that ensure the convergence of DDP algorithms. We test the data-driven Wasserstein DR-MCO models against multistage robust convex optimization (MRCO), risk-neutral and risk-averse multistage stochastic convex optimization (MSCO) models on multi-commodity inventory problems and hydro-thermal power planning problems. The results show that our DR-MCO models could outperform MRCO and MSCO models when the data size is small.
更多查看译文
关键词
optimization,convex,multistage,data-driven
AI 理解论文
溯源树
样例
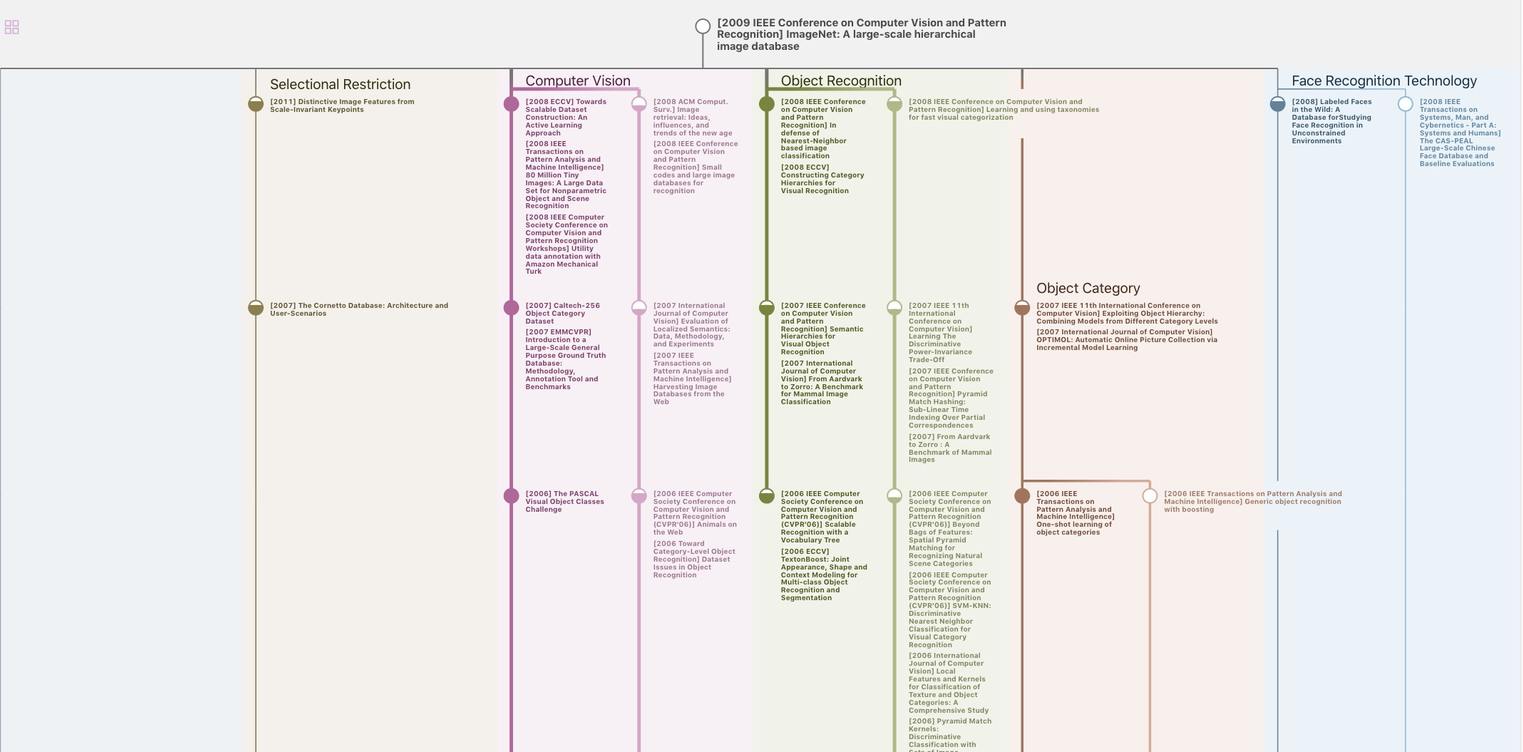
生成溯源树,研究论文发展脉络
Chat Paper
正在生成论文摘要