The Effects of Partitioning Strategies on Energy Consumption in Distributed CNN Inference at The Edge
arxiv(2022)
摘要
Nowadays, many AI applications utilizing resource-constrained edge devices (e.g., small mobile robots, tiny IoT devices, etc.) require Convolutional Neural Network (CNN) inference on a distributed system at the edge due to limited resources of a single edge device to accommodate and execute a large CNN. There are four main partitioning strategies that can be utilized to partition a large CNN model and perform distributed CNN inference on multiple devices at the edge. However, to the best of our knowledge, no research has been conducted to investigate how these four partitioning strategies affect the energy consumption per edge device. Such an investigation is important because it will reveal the potential of these partitioning strategies to be used effectively for reduction of the per-device energy consumption when a large CNN model is deployed for distributed inference at the edge. Therefore, in this paper, we investigate and compare the per-device energy consumption of CNN model inference at the edge on a distributed system when the four partitioning strategies are utilized. The goal of our investigation and comparison is to find out which partitioning strategies (and under what conditions) have the highest potential to decrease the energy consumption per edge device when CNN inference is performed at the edge on a distributed system.
更多查看译文
关键词
cnn inference,energy consumption,partitioning strategies,edge
AI 理解论文
溯源树
样例
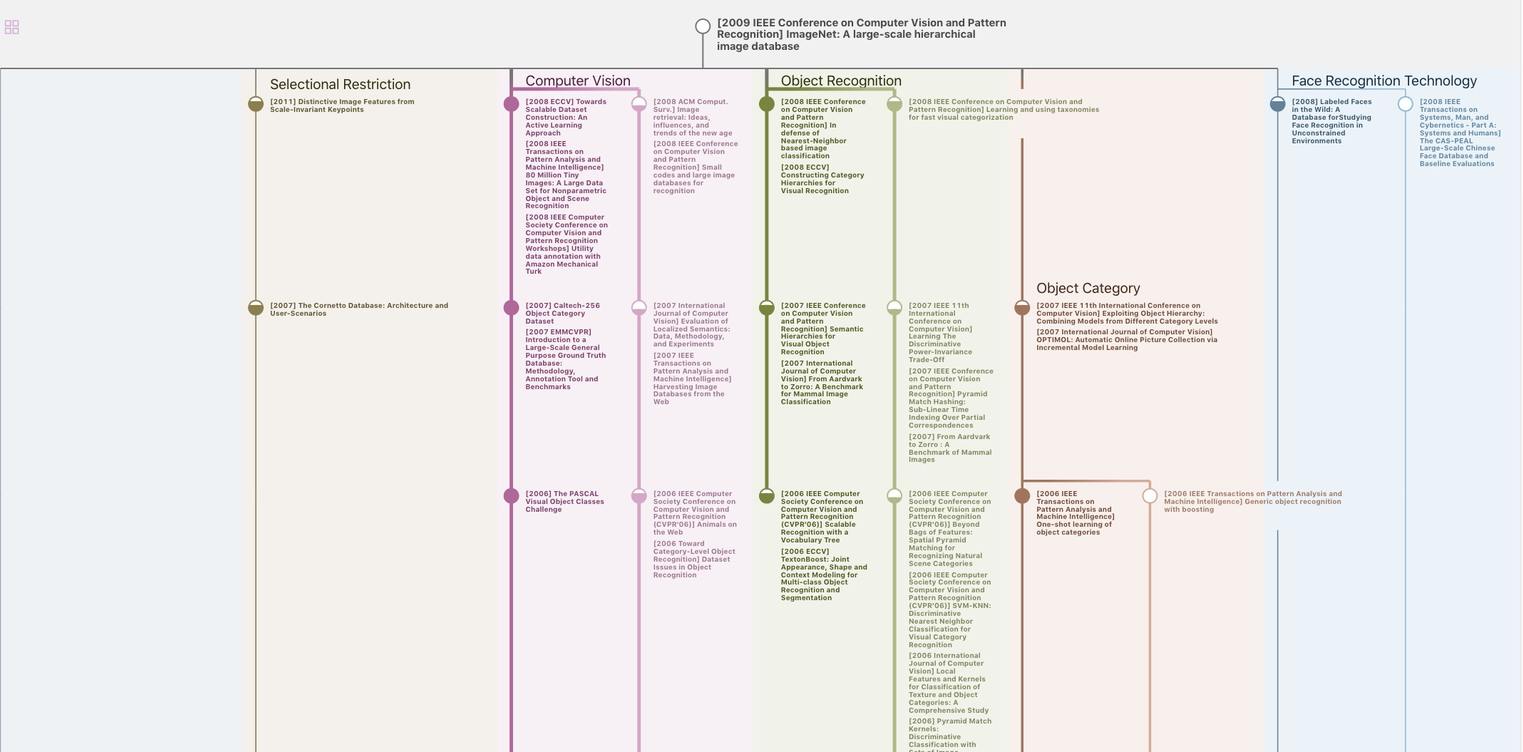
生成溯源树,研究论文发展脉络
Chat Paper
正在生成论文摘要