RoS-KD: A Robust Stochastic Knowledge Distillation Approach for Noisy Medical Imaging
arxiv(2022)
摘要
AI-powered Medical Imaging has recently achieved enormous attention due to its ability to provide fast-paced healthcare diagnoses. However, it usually suffers from a lack of high-quality datasets due to high annotation cost, interobserver variability, human annotator error, and errors in computer-generated labels. Deep learning models trained on noisy labelled datasets are sensitive to the noise type and lead to less generalization on the unseen samples. To address this challenge, we propose a Robust Stochastic Knowledge Distillation (RoS-KD) framework which mimics the notion of learning a topic from multiple sources to ensure deterrence in learning noisy information. More specifically, RoS-KD learns a smooth, well-informed, and robust student manifold by distilling knowledge from multiple teachers trained on overlapping subsets of training data. Our extensive experiments on popular medical imaging classification tasks (cardiopulmonary disease and lesion classification) using real-world datasets, show the performance benefit of RoS-KD, its ability to distill knowledge from many popular large networks (ResNet-50, DenseNet-121, MobileNetV2) in a comparatively small network, and its robustness to adversarial attacks (PGD, FSGM). More specifically, RoS-KD achieves >2% and > 4% improvement on F1-score for lesion classification and cardiopulmonary disease classification tasks, respectively, when the underlying student is ResNet-18 against recent competitive knowledge distillation baseline. Additionally, on cardiopulmonary disease classification task, RoS-KD outperforms most of the SOTA baselines by ~1% gain in AUC score.
更多查看译文
关键词
Cardiopulmonary Disease Classification,Knowledge distillation,Lesion Classification,Noisy Learning
AI 理解论文
溯源树
样例
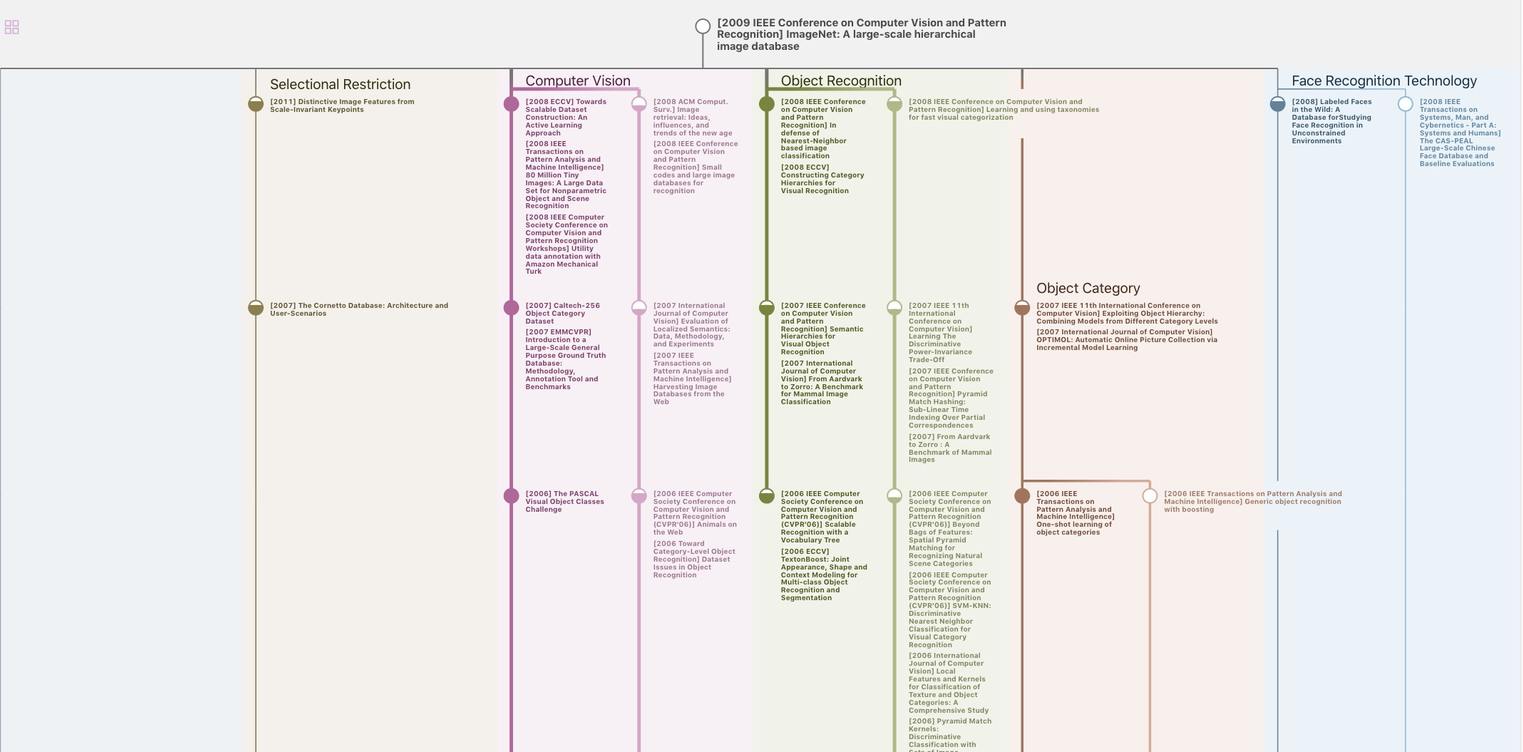
生成溯源树,研究论文发展脉络
Chat Paper
正在生成论文摘要