Self-Improving SLAM in Dynamic Environments: Learning When to Mask
arxiv(2022)
摘要
Visual SLAM - Simultaneous Localization and Mapping - in dynamic environments typically relies on identifying and masking image features on moving objects to prevent them from negatively affecting performance. Current approaches are suboptimal: they either fail to mask objects when needed or, on the contrary, mask objects needlessly. Thus, we propose a novel SLAM that learns when masking objects improves its performance in dynamic scenarios. Given a method to segment objects and a SLAM, we give the latter the ability of Temporal Masking, i.e., to infer when certain classes of objects should be masked to maximize any given SLAM metric. We do not make any priors on motion: our method learns to mask moving objects by itself. To prevent high annotations costs, we created an automatic annotation method for self-supervised training. We constructed a new dataset, named ConsInv, which includes challenging real-world dynamic sequences respectively indoors and outdoors. Our method reaches the state of the art on the TUM RGB-D dataset and outperforms it on KITTI and ConsInv datasets.
更多查看译文
关键词
slam,dynamic environments,learning,self-improving
AI 理解论文
溯源树
样例
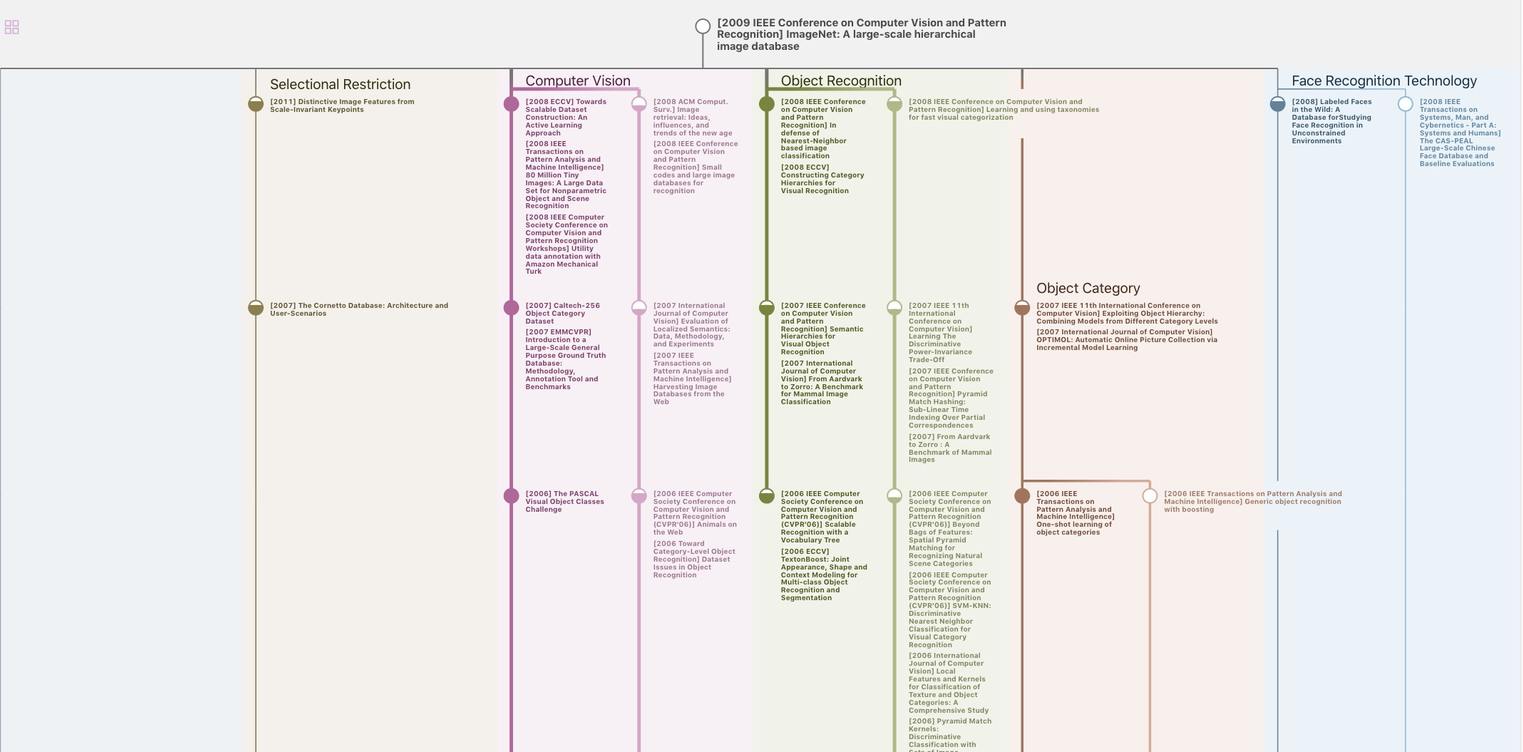
生成溯源树,研究论文发展脉络
Chat Paper
正在生成论文摘要