Providing Error Detection for Deep Learning Image Classifiers Using Self-Explainability
arxiv(2022)
摘要
This paper proposes a self-explainable Deep Learning (SE-DL) system for an image classification problem that performs self-error detection. The self-error detection is key to improving the DL system's safe operation, especially in safety-critical applications such as automotive systems. A SE-DL system outputs both the class prediction and an explanation for that prediction, which provides insight into how the system makes its predictions for humans. Additionally, we leverage the explanation of the proposed SE-DL system to detect potential class prediction errors of the system. The proposed SE-DL system uses a set of concepts to generate the explanation. The concepts are human-understandable lower-level image features in each input image relevant to the higher-level class of that image. We present a concept selection methodology for scoring all concepts and selecting a subset of them based on their contribution to the error detection performance of the proposed SE-DL system. Finally, we present different error detection schemes using the proposed SE-DL system to compare them against an error detection scheme without any SE-DL system.
更多查看译文
关键词
deep learning image classifiers,error detection,deep learning,self-explainability
AI 理解论文
溯源树
样例
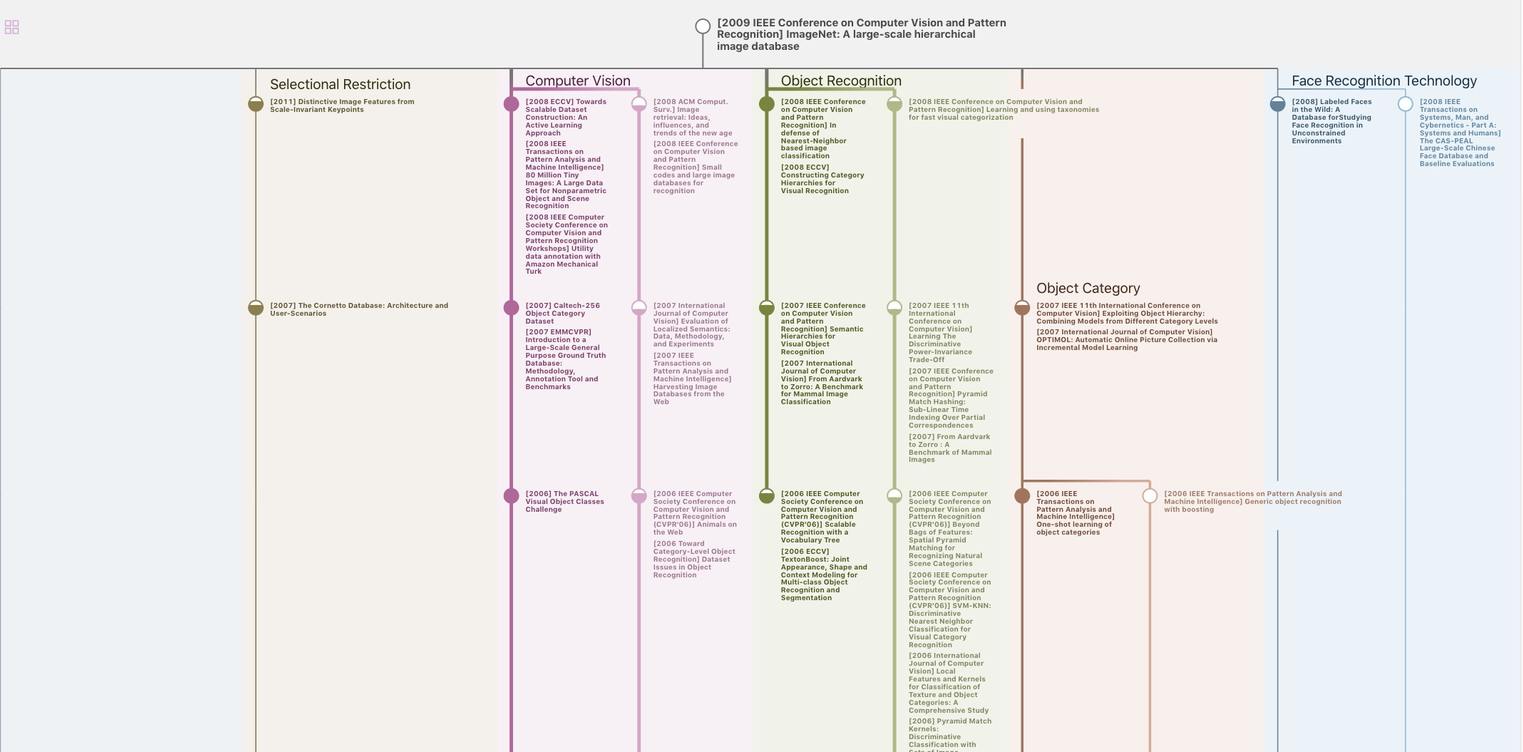
生成溯源树,研究论文发展脉络
Chat Paper
正在生成论文摘要