A Contrastive-Learning-Based Method for Alert-Scene Categorization
2022 IEEE Intelligent Vehicles Symposium (IV)(2022)
Abstract
Whether it’s a driver warning or an autonomous driving system, ADAS needs to decide when to alert the driver of danger or take over control. This research formulates the problem as an alert-scene categorization one and proposes a method using contrastive learning. Given a front-view video of a driving scene, a set of anchor points is marked by a human driver, where an anchor point indicates that the semantic attribute of the current scene is different from that of the previous one. The anchor frames are then used to generate contrastive image pairs to train a feature encoder and obtain a scene similarity measure, so as to expand the distance of the scenes of different categories in the feature space. Each scene category is explicitly modeled to capture the meta pattern on the distribution of scene similarity values, which is then used to infer scene categories. Experiments are conducted using front-view videos that were collected during driving at a cluttered dynamic campus. The scenes are categorized into no alert, longitudinal alert, and lateral alert. The results are studied at both feature encoding, category modeling, and reasoning aspects. By comparing precision with two full supervised end-to-end baseline models, the proposed method demonstrates competitive or superior performance. However, it remains still questions: how to generate ground truth data and how to evaluate performance in ambiguous situations, which leads to future works.
MoreTranslated text
Key words
scene similarity measure,scene category,scene similarity values,front-view video,alert alert,longitudinal alert, alert,lateral alert,feature encoding,category modeling,alert-scene categorization,driver warning,autonomous driving system,contrastive learning,driving scene,anchor point,human driver,current scene,anchor frames,contrastive image pairs,feature encoder
AI Read Science
Must-Reading Tree
Example
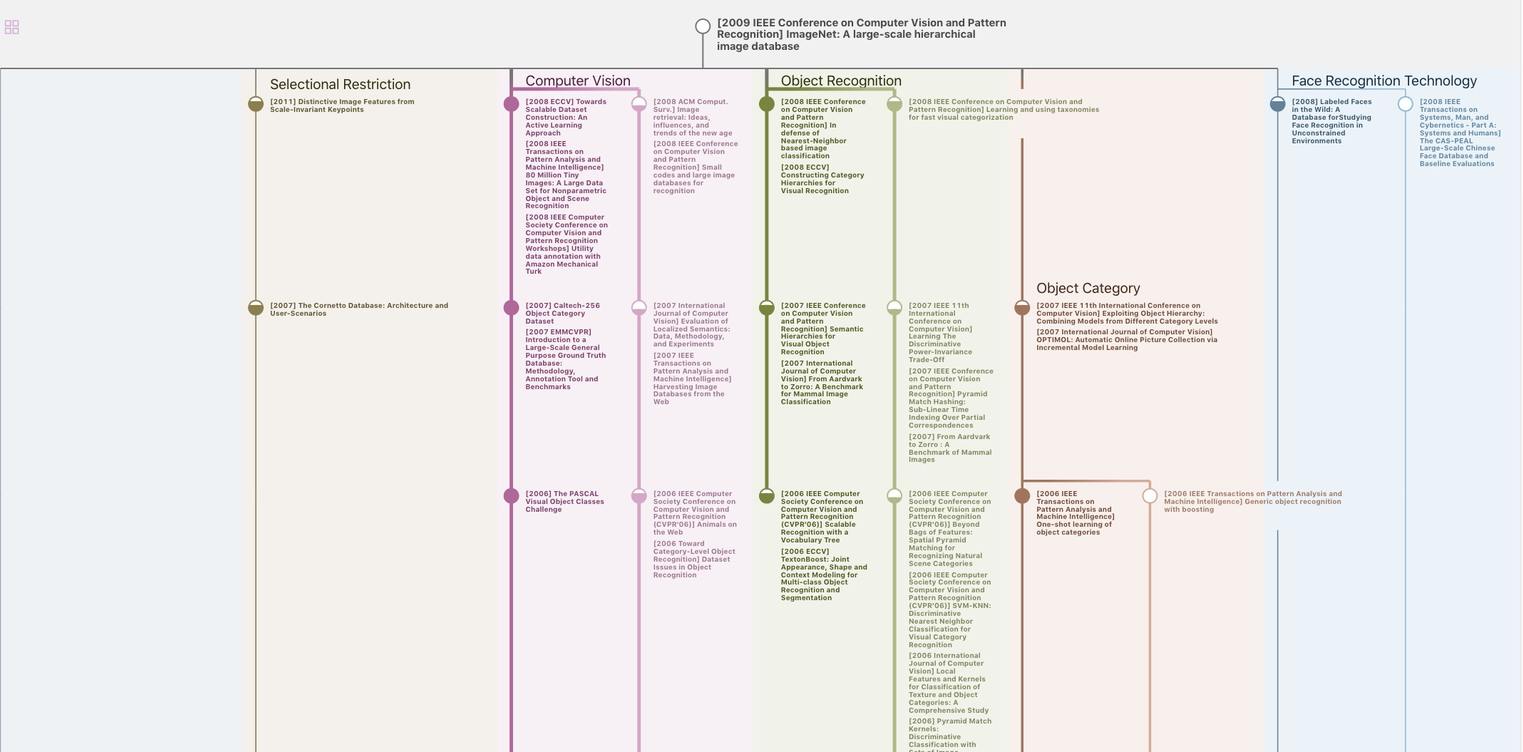
Generate MRT to find the research sequence of this paper
Chat Paper
Summary is being generated by the instructions you defined