A double fixed rank kriging approach to spatial regression models with covariate measurement error
Environmetrics(2023)
Abstract
In many applications of spatial regression modeling, the spatially indexed covariates are measured with error, and it is known that ignoring this measurement error can lead to attenuation of the estimated regression coefficients. Classical measurement error techniques may not be appropriate in the spatial setting, due to the lack of validation data and the presence of (residual) spatial correlation among the responses. In this article, we propose a double fixed rank kriging (FRK) approach to obtain bias-corrected estimates of and inference on coefficients in spatial regression models, where the covariates are spatially indexed and subject to measurement error. Assuming they vary smoothly in space, the proposed method first fits an FRK model regressing the covariates against spatial basis functions to obtain predictions of the error-free covariates. These are then passed into a second FRK model, where the response is regressed against the predicted covariates plus another set of spatial basis functions to account for spatial correlation. A simulation study and an application to presence-absence records of Carolina wren from the North American Breeding Bird Survey demonstrate that the proposed double FRK approach can be effective in adjusting for measurement error in spatially correlated data.
MoreTranslated text
Key words
basis functions,Laplace approximation,regression calibration,sandwich covariance,simulation extrapolation,spatial generalized linear mixed model
AI Read Science
Must-Reading Tree
Example
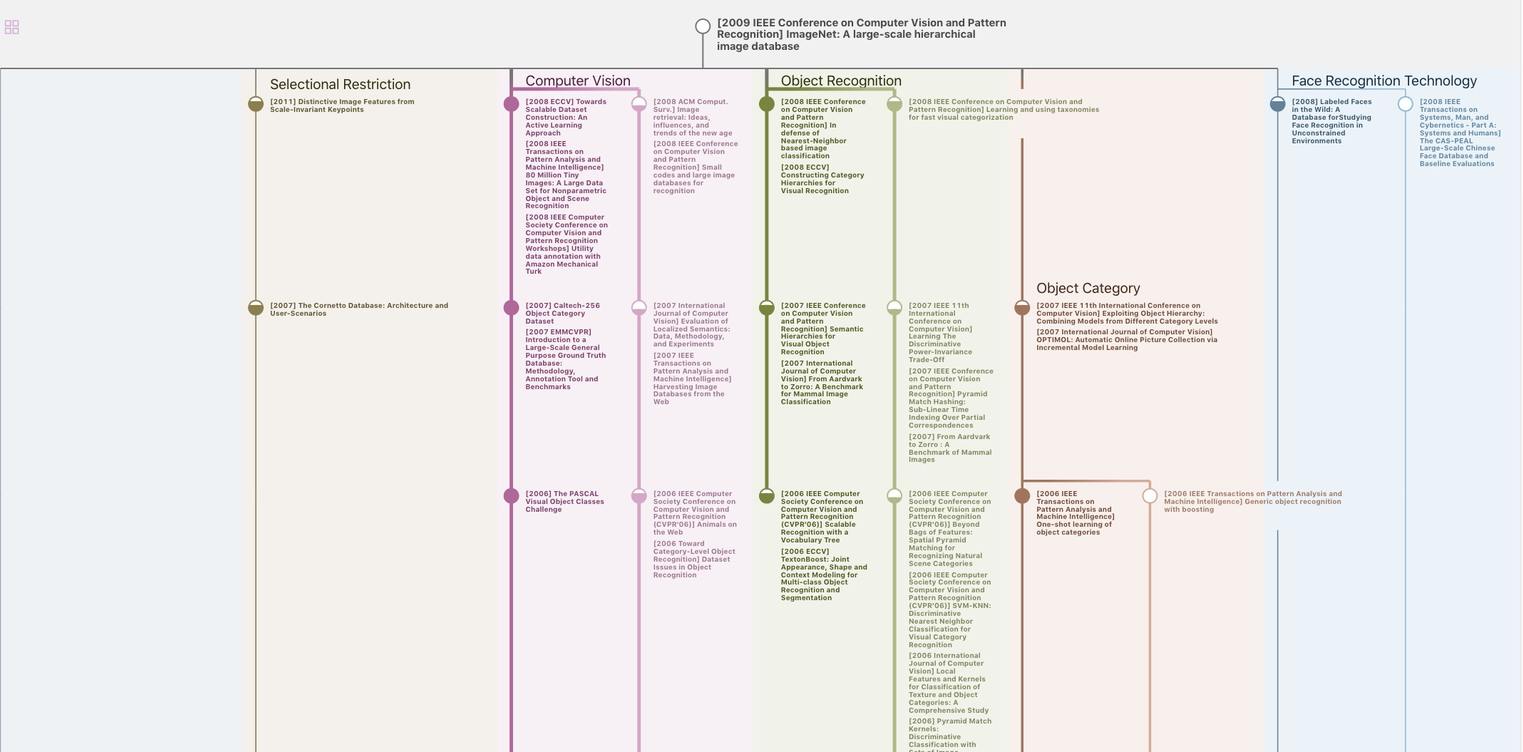
Generate MRT to find the research sequence of this paper
Chat Paper
Summary is being generated by the instructions you defined