GenoPPML – a framework for genomic privacy-preserving machine learning
2022 IEEE 15th International Conference on Cloud Computing (CLOUD)(2022)
摘要
We present a framework GenoPPML for privacy-preserving machine learning in the context of sensitive genomic data processing. The technology combines secure multiparty computation techniques based on the recently proposed Manticore framework for model training and fully homomorphic encryption based on TFHE for model inference. The framework was successfully used to solve breast cancer prediction problems on gene expression datasets coming from distinct private sources while preserving their privacy - the solution winning 1st place for both Tracks I and III of the genomic privacy competition iDASH’2020. Extensive benchmarks and comparisons to existing works are performed. Our 2-party logistic regression computation is 11× faster than the one in [1] on the same dataset and it uses only one CPU core.
更多查看译文
关键词
privacy-preserving machine learning,multiparty computation,homomorphic encryption,genomic privacy,differential privacy
AI 理解论文
溯源树
样例
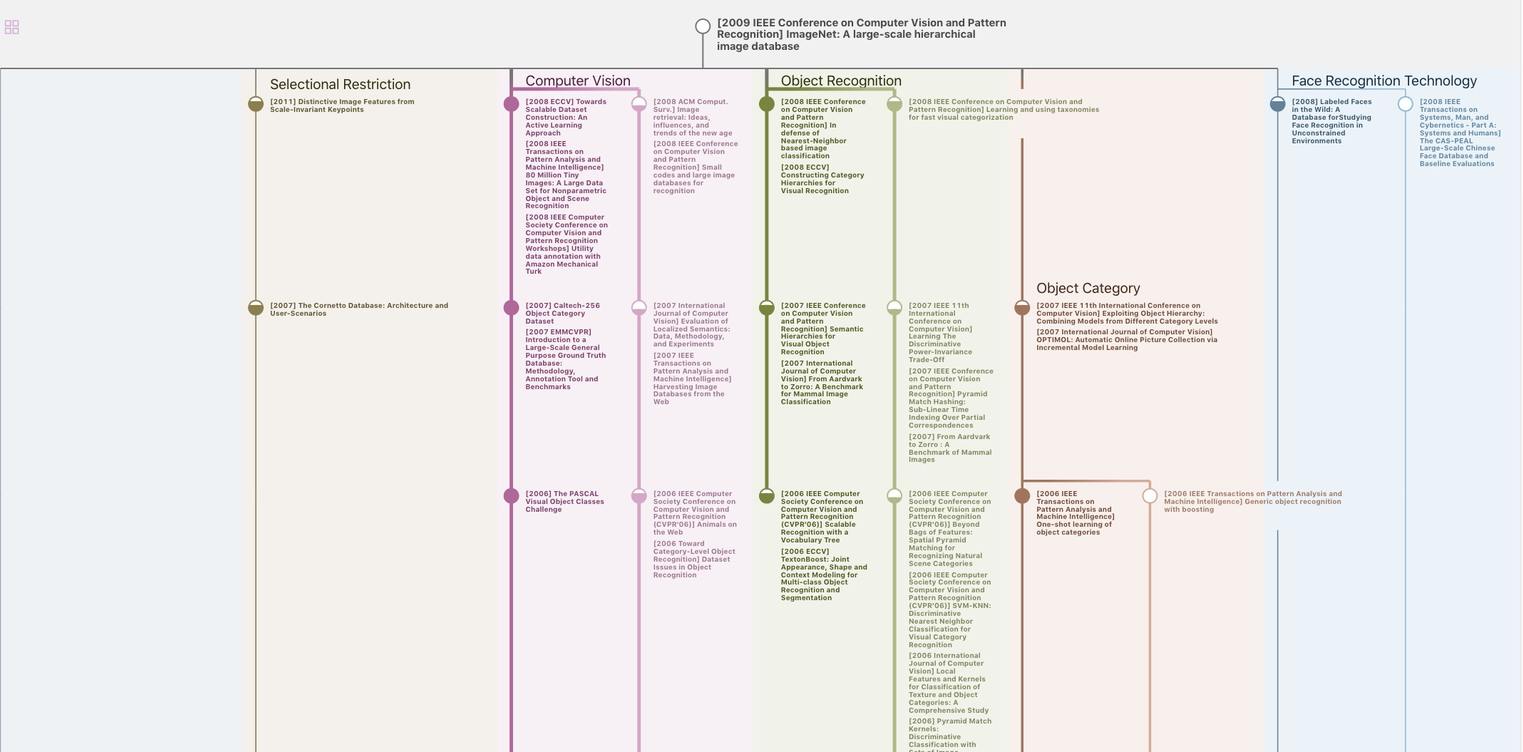
生成溯源树,研究论文发展脉络
Chat Paper
正在生成论文摘要