Relevance-Based Big Data Exploration for Smart Road Maintenance
International Conference on Cooperative Information Systems (CoopIS)(2022)
摘要
In the latest years, the progressive digitalisation of Smart City ecosystems has fuelled an increasing availability of data from sensor networks, which is considered as a valuable asset for improving mobility resilience. In particular, data coming from sensors in vehicles can be leveraged to obtain useful information about the quality of the area-wide road surface in near real-time, and may be used by road maintainers to focus monitoring and maintenance activities on urban and public infrastructure. To bring such application scenario into the field, road maintainers should be equipped with valuable tools to gain insights from the data and ensure a safer and more efficient infrastructure. In this paper, we present a methodological approach, based on big data exploration techniques, applied to support road maintainers in analysing and assessing surface conditions of roads. Specifically, the proposed approach is grounded on three components: (i) a multi-dimensional model, apt to represent the road network and to enable data exploration; (ii) data summarisation techniques, in order to simplify overall view over high volumes of data collected by vehicles; (iii) a measure of relevance, aimed at focusing the attention of the maintainers on relevant data only. The paper illustrates the design and implementation of multiple exploration scenarios on top of the three components and their implementation and preliminary evaluation in an ongoing research project on sustainable and resilient mobility.
更多查看译文
关键词
smart road maintenance,big data exploration,relevance-based
AI 理解论文
溯源树
样例
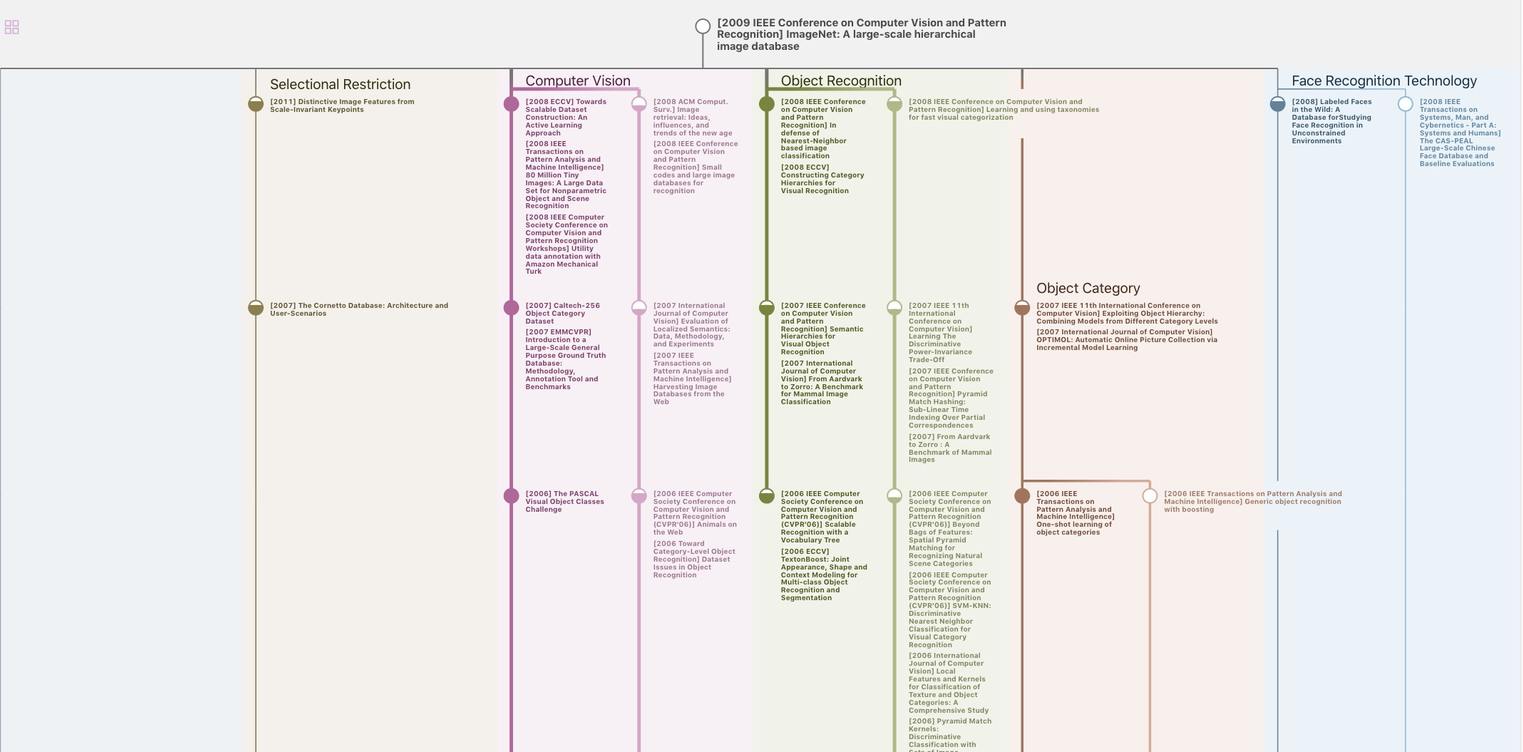
生成溯源树,研究论文发展脉络
Chat Paper
正在生成论文摘要