RefTextLAS: Reference Text Biased Listen, Attend, and Spell Model For Accurate Reading Evaluation
Conference of the International Speech Communication Association (INTERSPEECH)(2022)
摘要
We present an automatic reading evaluator that listens to novice young readers and offers feedback based on the reading accuracy. In order to not discourage the reader, the model should not misrecognize correctly read tokens (false rejects), which may come at the expense of tolerating some reading mistakes (false accepts). To minimize the former, we explore two approaches to provide reference text - the text user is supposed to read as context to automatic speech recognition (ASR) models: 1) a finite state transducer (FST) based error detection procedure, that restricts the grammar to tokens from reference text and an out of vocabulary (OOV) catch-all token, and 2) RefTextLAS, an attention-based end-to-end (E2E) ASR model, that takes tokens from reference text as an additional input. Our biasing approaches reduce false reject rate (FRR) by 38-56% on an in-house dataset compared to a baseline hybrid model, whose language model (LM) is trained on book texts, with 65-82% compromise on false accept rate (FAR). To reduce FAR, we present an ensemble approach that uses both baseline and RefTextLAS models to determine reading accuracy. The ensemble approach limits the relative degradation on FAR to 26.4% while providing a 42.7% improvement on FRR.
更多查看译文
关键词
reference reftextlas biased listen,spell model,reading
AI 理解论文
溯源树
样例
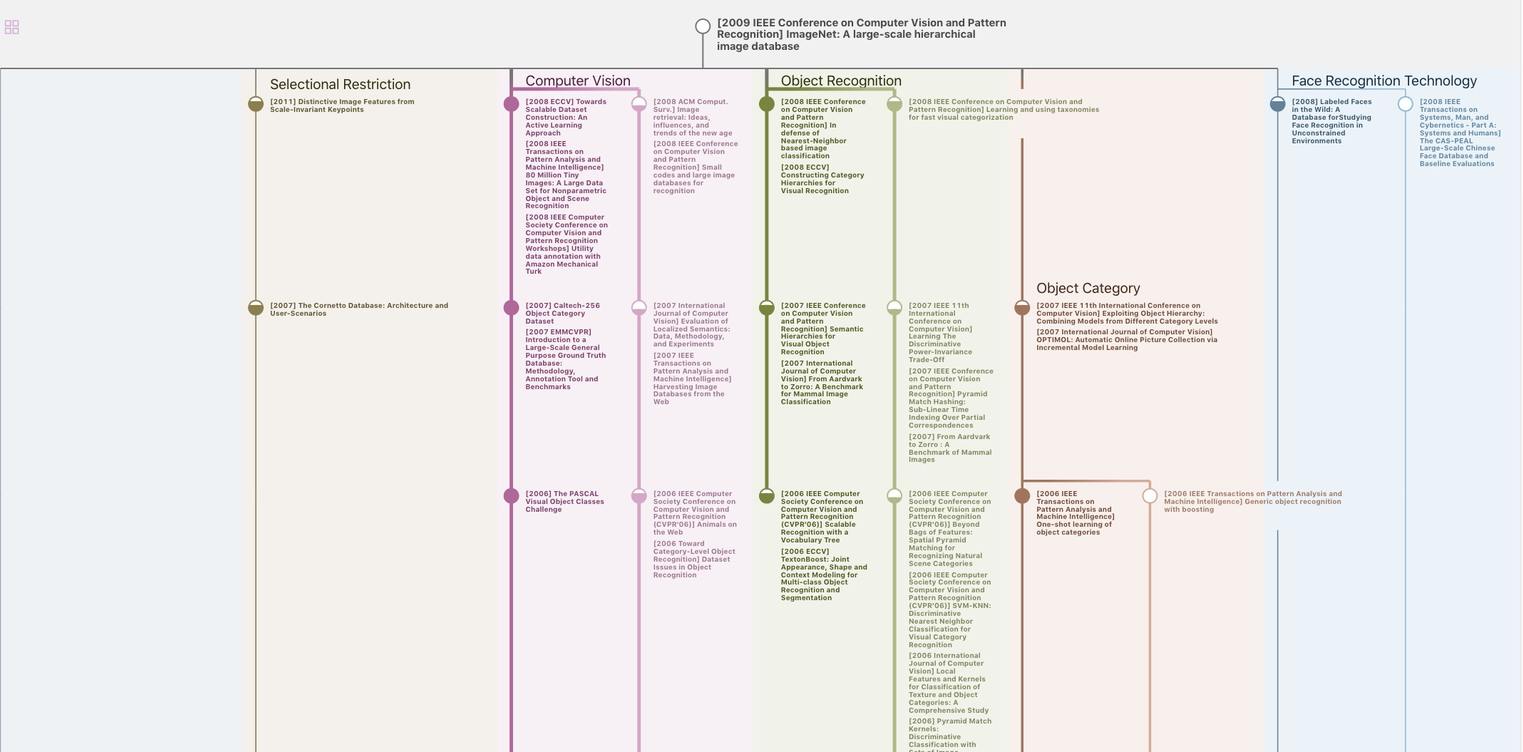
生成溯源树,研究论文发展脉络
Chat Paper
正在生成论文摘要