SiD-WaveFlow: A Low-Resource Vocoder Independent of Prior Knowledge
Conference of the International Speech Communication Association (INTERSPEECH)(2022)
摘要
Flow-based neural vocoders have demonstrated their effectiveness in generating high-fidelity speech in real-time. However, most flow-based vocoders are computationally heavy models which rely on large amounts of speech for model training. Witnessing the limitations of these vocoders, a new flow-based vocoder, namely Semi-inverse Dynamic WaveFlow (SiD-WaveFlow), for low-resource speech synthesis is proposed. SiD-WaveFlow can generate high-quality speech in real-time with the constraint of limited training data. Specifically, in SiD-WaveFlow, a module named Semi-inverse Dynamic Transformation (SiDT) is proposed to improve the synthesis quality as well as the computational efficiency by replacing the affine coupling layers (ACL) used in WaveGlow. In addition, a pre-emphasis operation is introduced to the training process of SiD-WaveFlow to further improve the quality of the synthesized speech. Experimental results have corroborated that SiD-WaveFlow can generate speech with better quality compared with its counterparts. Particularly, the TTS system integrating SiD-WaveFlow vocoder achieves 3.416 and 2.968 mean MOS on CSMSC and LJ speech dataset, respectively. Besides, SiD-WaveFlow converges much faster than WaveGlow at the training stage. Last but not least, SiD-WaveFlow is a lightweight model and can generate speech on edge devices with a much faster inference speed. The source code and demos are available at https://slptongji.github.io/.
更多查看译文
关键词
speech synthesis, generative models, low-resource, neural vocoder
AI 理解论文
溯源树
样例
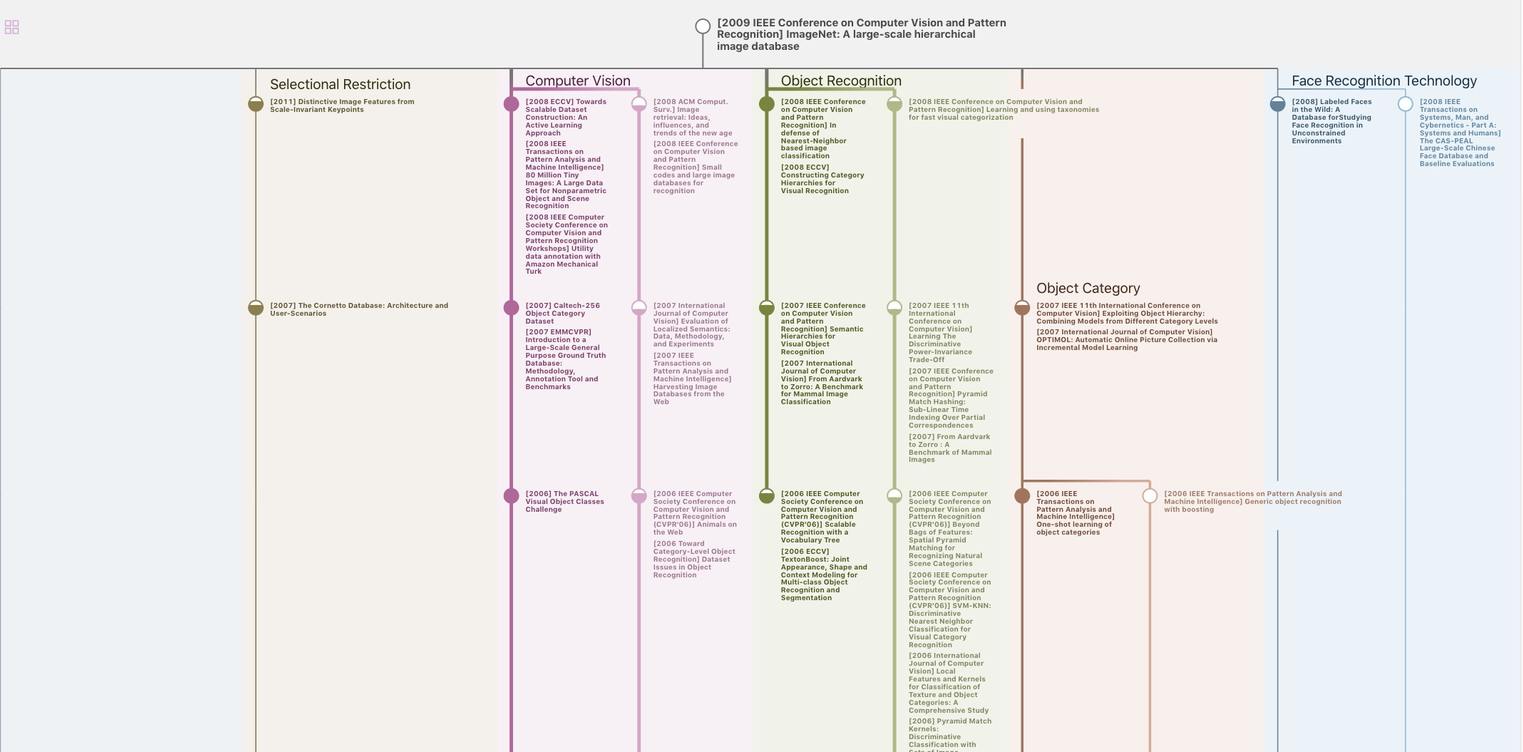
生成溯源树,研究论文发展脉络
Chat Paper
正在生成论文摘要