Knowledge Distillation via Module Replacing for Automatic Speech Recognition with Recurrent Neural Network Transducer
Conference of the International Speech Communication Association (INTERSPEECH)(2022)
摘要
Automatic Speech Recognition (ASR) is increasingly used by edge applications such as intelligent virtual assistants. However, state-of-the-art ASR models such as Recurrent Neural Network - Transducer (RNN-T) are computationally intensive on resource-constrained edge devices. Knowledge Distillation (KD) is a promising approach to compress large models by using a large model ("teacher") to train a small model ("student"). This paper proposes a novel KD method called Log-Curriculum based Module Replacing (LCMR) for RNN-T. LCMR compresses RNN-T and addresses its unique characteristics by replacing teacher modules including multiple LSTM/Dense layers with substitutional student modules that contain less Long Short Term Memory (LSTM)/Dense layers. LCMR employs a novel nonlinear Curriculum Learning driven replacement strategy to further improve the performance by updating replacing rates with a dynamic, smoothing mechanism. Under LCMR, the student and teacher are able to interact at gradient level, and tranfser knowledge more effectively than conventional KD. Evaluation shows that LCMR reduces word-error-rate (WER) by 14.47%-33.24% relative compared to conventional KD.
更多查看译文
关键词
recurrent neural network transducer,automatic speech recognition,knowledge
AI 理解论文
溯源树
样例
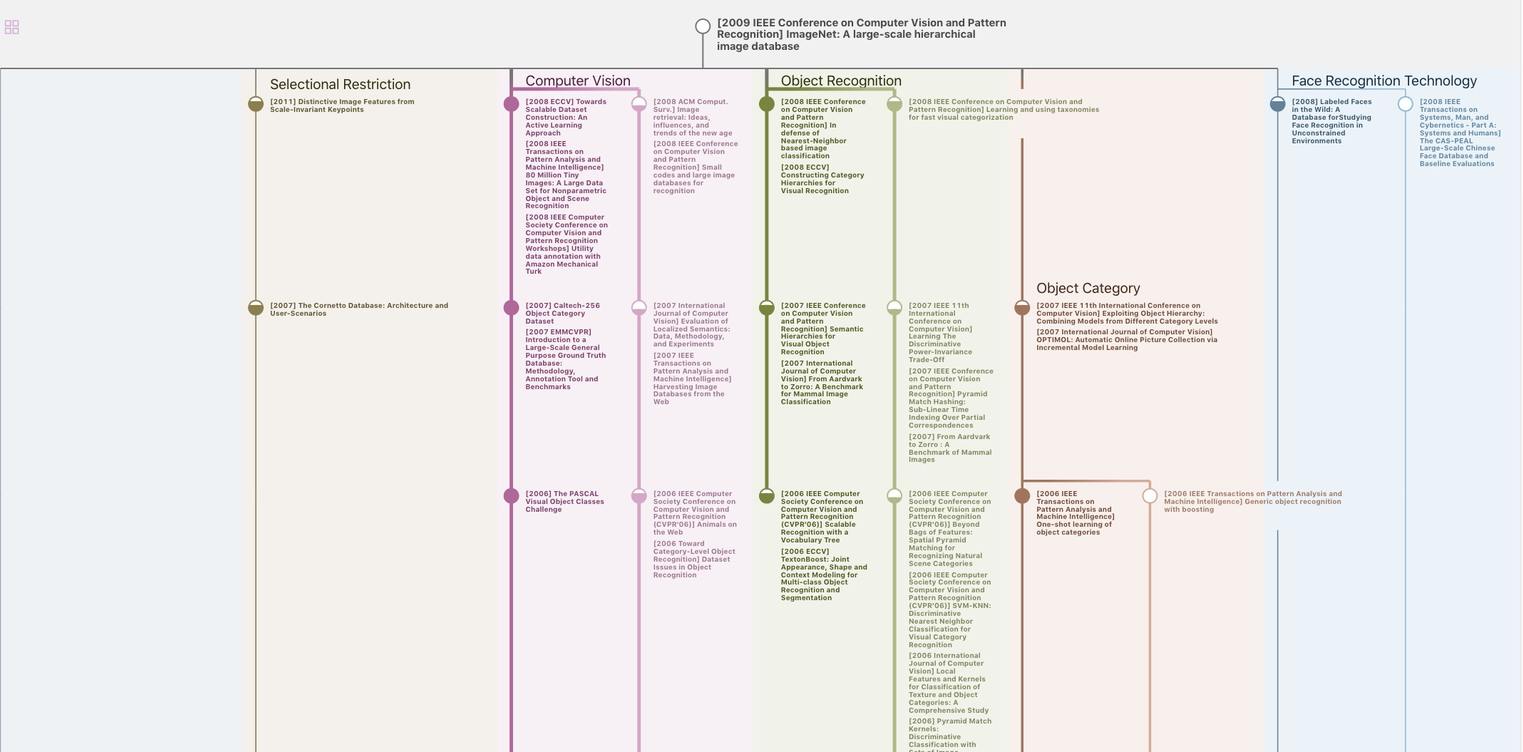
生成溯源树,研究论文发展脉络
Chat Paper
正在生成论文摘要