A study on constraining Connectionist Temporal Classification for temporal audio alignment
Conference of the International Speech Communication Association (INTERSPEECH)(2022)
摘要
Connectionist Temporal Classification (CTC) has become a standard for deep learning-based temporal alignment allowing relevant probabilistic distributions to be learned. However, by nature, CTC is a transcription objective that can be minimized without guaranteeing any alignment properties. This work aims to study several constraints to help CTC generating alignments. With a fully convolutional architecture coupled with multi-head attention, we investigate the task of phonetic alignment for clean speech and singing signals. The focus is set on the impact of additional losses, namely spectral envelope reconstruction, temporal structure invariance and guided monotony. Results show that, once scaled to have identical temporal dependence, combining all of these constraints produces best performances.
更多查看译文
关键词
connectionist temporal classification,alignment
AI 理解论文
溯源树
样例
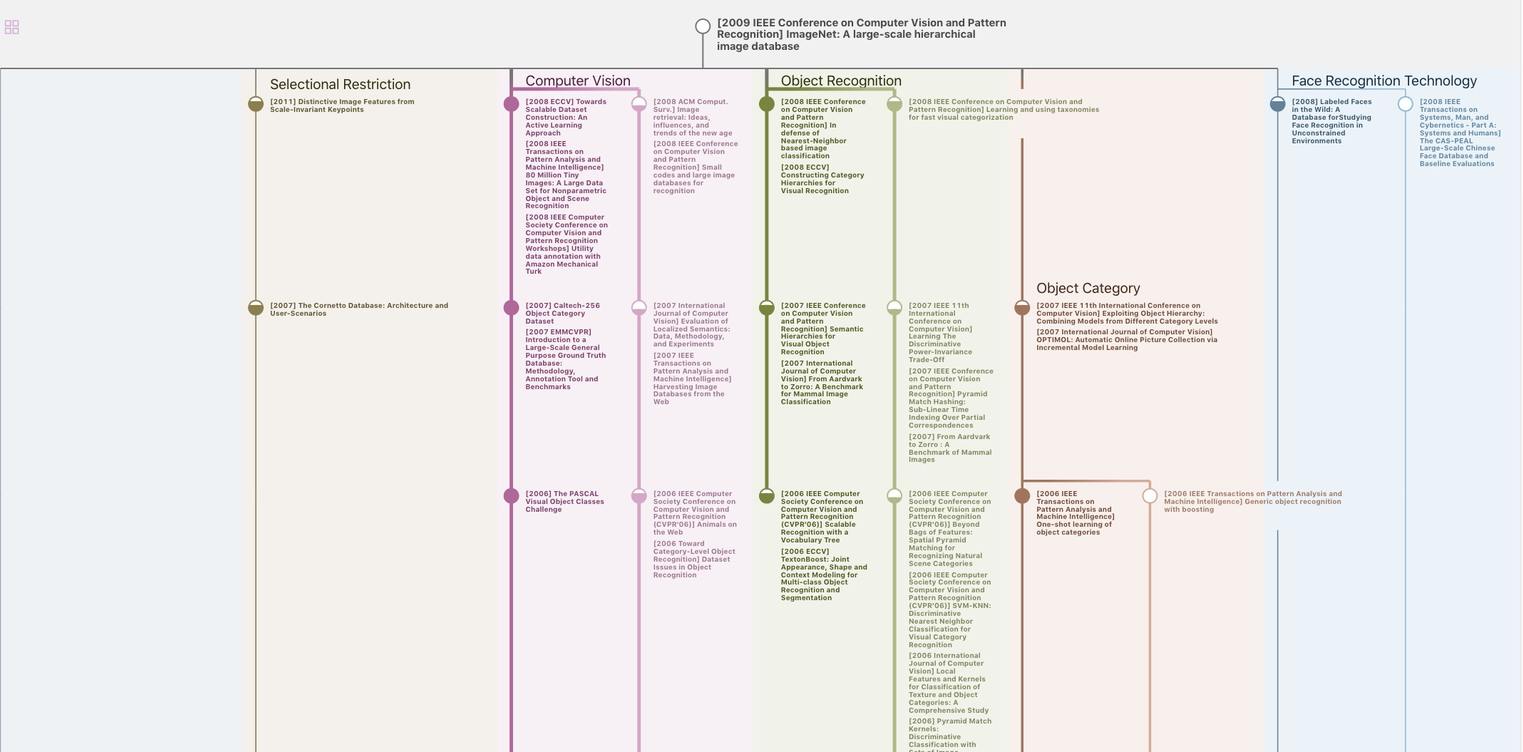
生成溯源树,研究论文发展脉络
Chat Paper
正在生成论文摘要