ADAM: An Attentional Data Augmentation Method for Extreme Multi-label Text Classification
ADVANCES IN KNOWLEDGE DISCOVERY AND DATA MINING, PAKDD 2022, PT I(2022)
摘要
Extreme Multi-label text Classification (XMC) is a fundamental text mining task, which aims to assign multiple labels related to the given text from a large-scale label set. Various models and many data augmentation methods are proposed to improve classification performance. However, the classification performance is limited due to the long tail distribution of labels, which is an essential characteristic of XMC. To address this problem, we propose a novel data augmentation method named Attentional Data Augmentation Method (ADAM) for long tail labels. Specifically, we split each sentence into several segments of equal length and use an attention-based neural network to explore the core segments of long tail labels. The unimportant segments of each instance from the dataset are considered to be replaced by those segments related to the long tail labels. Extensive experiments show that ADAM has an improvement based on the XMC method, especially on the prediction of long tail labels.
更多查看译文
关键词
Extreme multi-label text classification, Long tail labels, Data augmentation
AI 理解论文
溯源树
样例
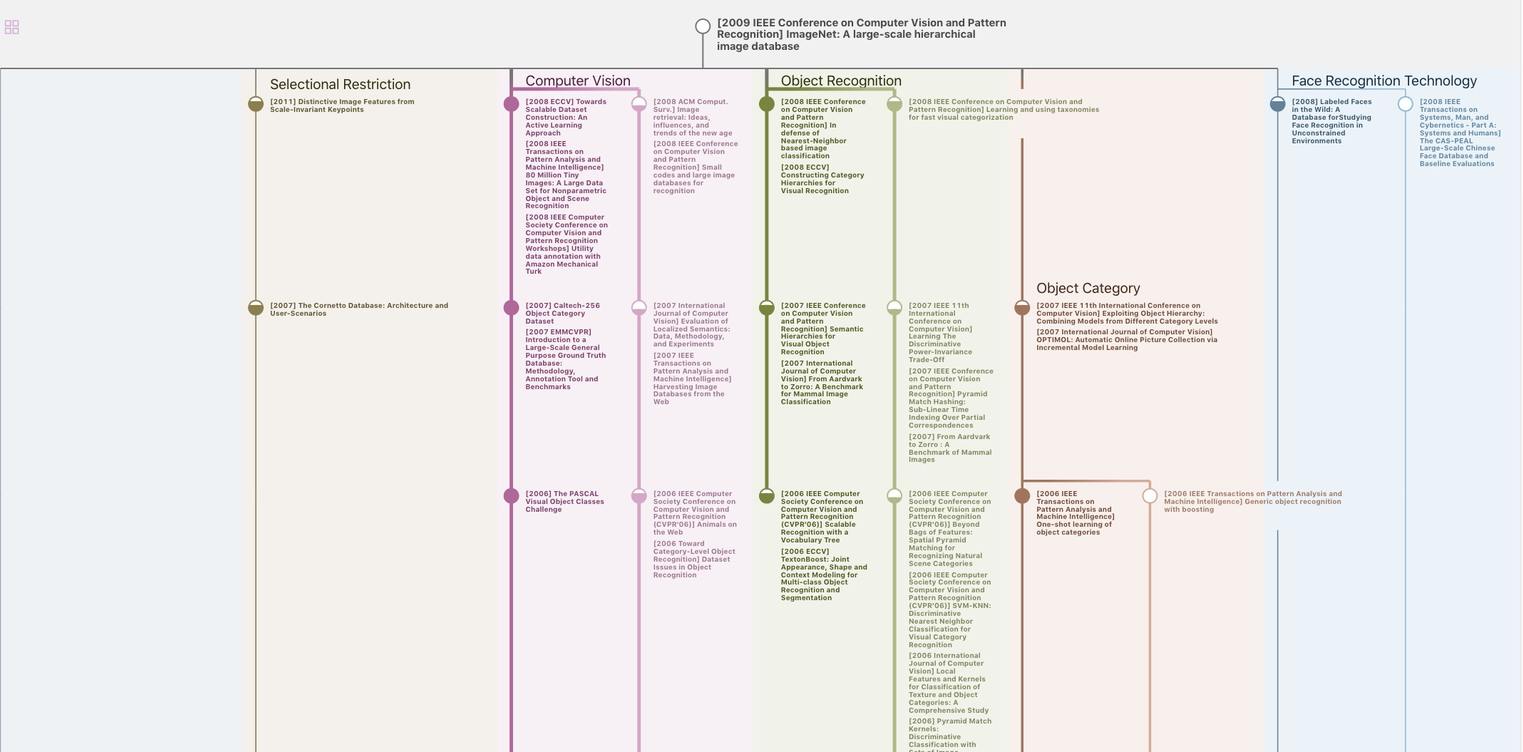
生成溯源树,研究论文发展脉络
Chat Paper
正在生成论文摘要