Effective Malicious URL Detection by Using Generative Adversarial Networks
WEB ENGINEERING (ICWE 2022)(2022)
摘要
Malicious URL, a.k.a. malicious website, pose a great threat to Web security. In particular, concept drift caused by variants of malicious URL degrades the performance of existing detection methods based on the available attack patterns. In this paper, We conduct an extensive measurement study of the realistic URL and find that the hierarchical semantics feature is suitable for identifying malicious URL. Therefore, we propose URLGAN, a deep neural network model equipped with the hierarchical semantics features, to detect distinguish between malicious and normal URL. Firstly, we embed the entire URL into a hierarchical semantics structure. Secondly, hierarchical semantics features are extracted from the hierarchical semantics structure through BERT. Then, the extracted features are combined with features generated by the generator, similar but slightly different, to enable the condition discriminator to extract the essential difference between normal and malicious URL. Notably, with the features generated by the generator, we enhance the robustness of the system to detect malicious URL variants. Extensive experiments on the public dataset and our data collected from specific targets demonstrate that our method achieves superior performance to other methods and protects specific targets from the susceptibility of malicious URL.
更多查看译文
关键词
Web security, Deep learning, Malicious URL Detection, Hierarchical semantics features, Concept drift
AI 理解论文
溯源树
样例
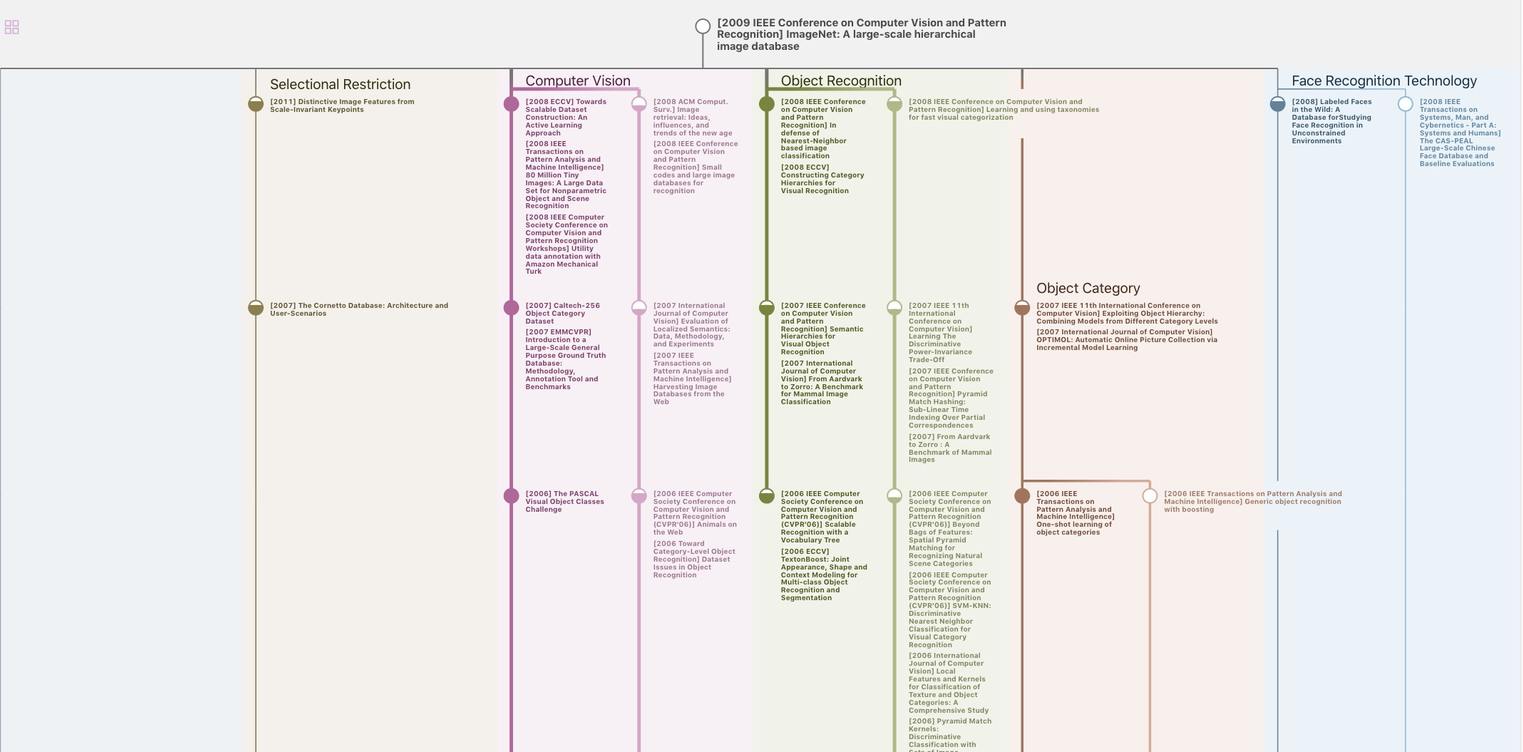
生成溯源树,研究论文发展脉络
Chat Paper
正在生成论文摘要