Comparative Analysis of Neural Networks Techniques for Lithium-ion Battery SOH Estimation
2022 IEEE 46TH ANNUAL COMPUTERS, SOFTWARE, AND APPLICATIONS CONFERENCE (COMPSAC 2022)(2022)
摘要
Li-ion batteries have become the most important technology for electric mobility. One of the most pressing challenges is the development of reliable methods for battery state-of-health (SOH) diagnosis and estimation of remaining useful life. In electric mobility scenario, battery capacity degradation prediction is crucial to ensure service availability and life duration. This research work provides a comprehensive comparative analysis of neural networks for a data-driven approach suitable for SOH estimation on single cells, stressed under laboratory conditions. For this purpose, different neural networks (i.e., LSTM, GRU, 1D-CNN, CNN-LSTM) are trained and optimized on NASA Randomized Battery Usage dataset. Experimental results demonstrate that data-driven neural networks generally performed well SOH estimation on single cells. In detail, the 1D-CNN best predicts SOH and has the lowest variance in the output. The LSTM have the highest variance in estimating SOH, while GRU and CNN-LSTM tend to overestimate and underestimate the value of SOH, respectively.
更多查看译文
关键词
Li-ion battery, State-of-Health, Electric Vehicles, neural networks, Deep Learning
AI 理解论文
溯源树
样例
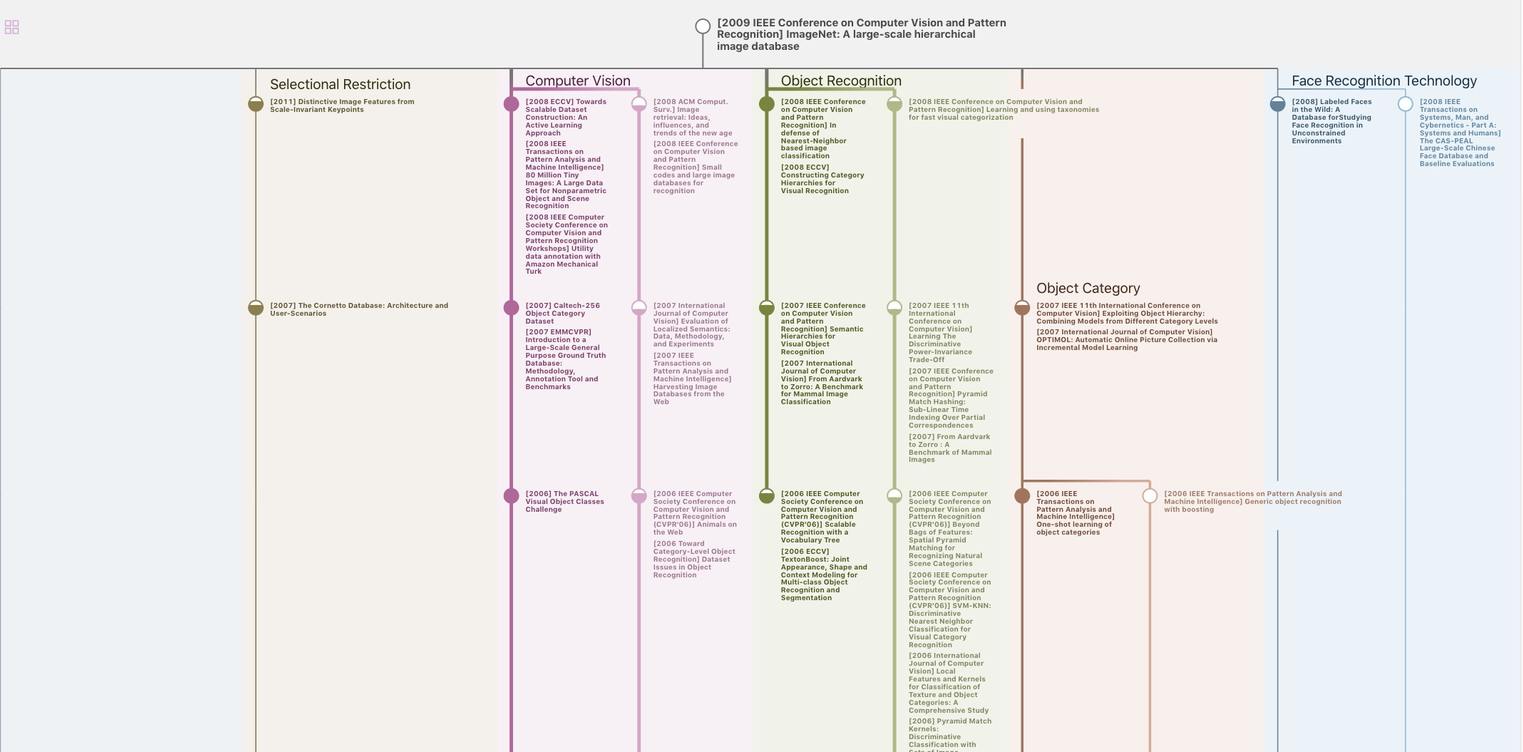
生成溯源树,研究论文发展脉络
Chat Paper
正在生成论文摘要