An Efficient Small for Gestational Age Prognosis System Using Stacked Generalization Scheme (SGS)
2022 IEEE 46TH ANNUAL COMPUTERS, SOFTWARE, AND APPLICATIONS CONFERENCE (COMPSAC 2022)(2022)
Abstract
Background: Classification of infants has always been considered a crucial task in the literature related to predicting small for gestational age (SGA) infants. Traditional medical guidance becomes increasingly unsatisfactory, as patients' care should be centered not only on clinical symptoms but also on socio-economic and demographic factors. Infants with excessive gestational weight exhibit serious maternal complications that require early intervention to streamline the progression of the disease. Methods: This research proposes to use the Stacked Generalization Scheme (SGS) to predict Small for Gestational (SGA) Infants on the dataset collected from the National Pre-Pregnancy and Examination Program of China. A Cleaned Feature Vector (CFV) is created that entertains issues related to missing values, discretization of fields, and data imbalance. Later, Knowledge-Driven Data (KDD) Features are extracted from the obtained CFV, and the proposed scheme is applied to predict SGA infants. The proposed scheme superposed the existing baseline approaches by achieving the highest precision, recall, and AUC scores of 0.94, 0.85, and 0.89, respectively. Conclusion: The proposed SGS can predict SGA infants accurately compared to existing baseline schemes using KDD parameters, which can help pediatricians develop an efficient SGA Prognosis process.
MoreTranslated text
Key words
Small for gestational age, feature selection, stacked generalization, knowledge driven features, machine learning
AI Read Science
Must-Reading Tree
Example
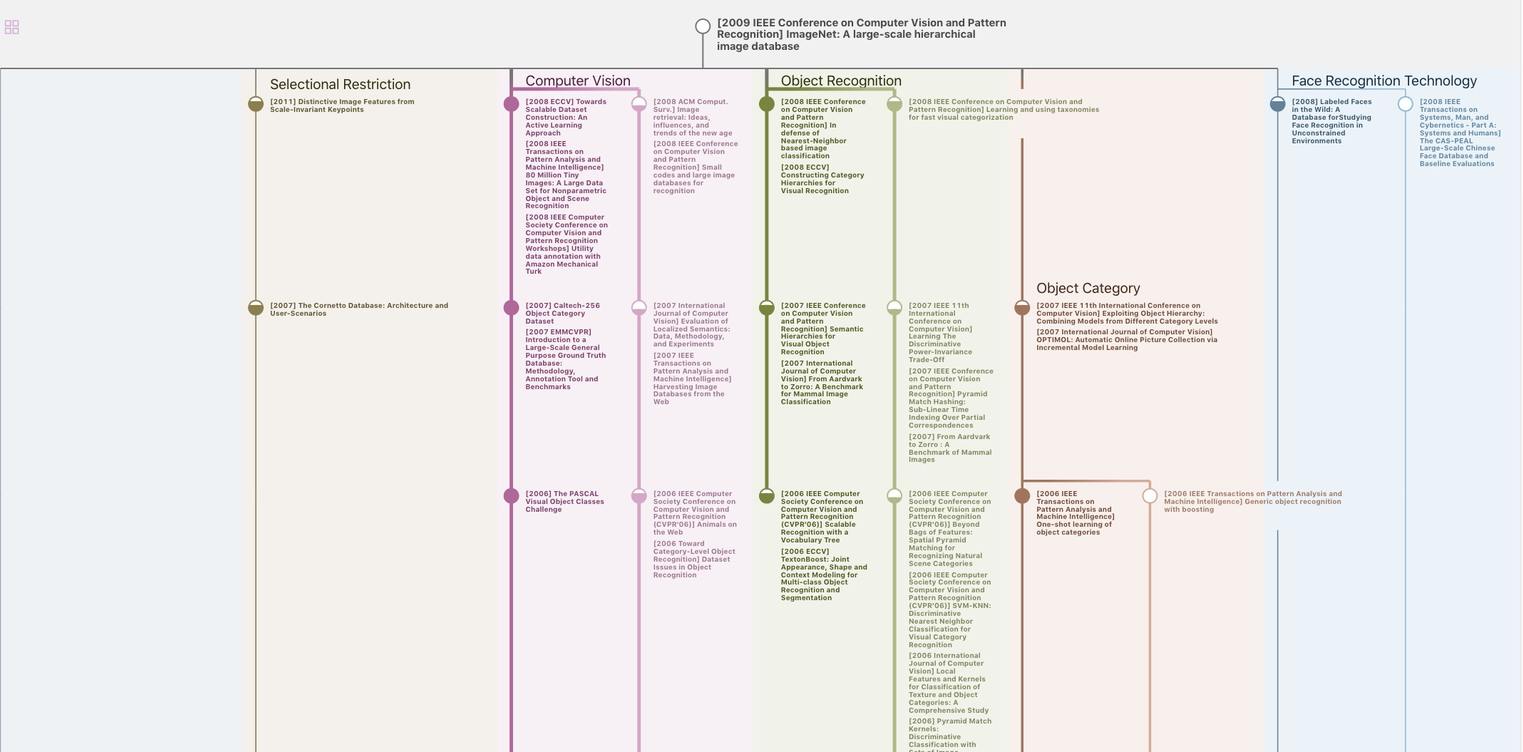
Generate MRT to find the research sequence of this paper
Chat Paper
Summary is being generated by the instructions you defined