A Deep Reinforcement Learning Approach for Collaborative Mobile Edge Computing
IEEE International Conference on Communications (ICC)(2022)
摘要
Mobile edge computing (MEC) is a promising approach to reduce the network traffic load and alleviate the backhaul congestion by pushing computation down to the network edge (e.g., base stations) that are close to the origin of data. However, when many mobile devices (MDs) offload tasks to a base station (BS) in a dynamic and stochastic environment (e.g., with time-varying wireless channels and uncertain task models), it is often challenging for MDs to make offloading decisions in decentralized manner. In this work, we consider a collaborative MEC scenario, where an MD can offload its task to the associated BS or to other BSs through the associated BS. In such a scenario, we study the joint computation offloading and resource allocation problem, aiming at minimizing the expected long-term delay, taking the energy consumption constraint into consideration. The problem is challenging due to time-varying system and distributed decisions. To solve the problem in an online and decentralized manner, we propose a deep reinforcement learning (DRL) based distributed online algorithm. By incorporating the double deep Q network and dueling deep Q network technique, the proposed algorithm can improve the performance of the whole system significantly. Simulation results show that the proposed DRL-based algorithm outperforms baseline methods and can reduce the average delay of tasks by 76.4%-91.2%.
更多查看译文
关键词
collaborative mobile edge computing,network traffic load,back-haul congestion,network edge,base station,mobile devices offload tasks,MDs,dynamic environment,stochastic environment,time-varying wireless channels,uncertain task models,offloading decisions,collaborative MEC scenario,associated BS,joint computation offloading,resource allocation problem,long-term delay,energy consumption constraint,time-varying system,distributed decisions,online manner,deep reinforcement learning,double deep Q network,dueling deep Q network technique,DRL-based algorithm
AI 理解论文
溯源树
样例
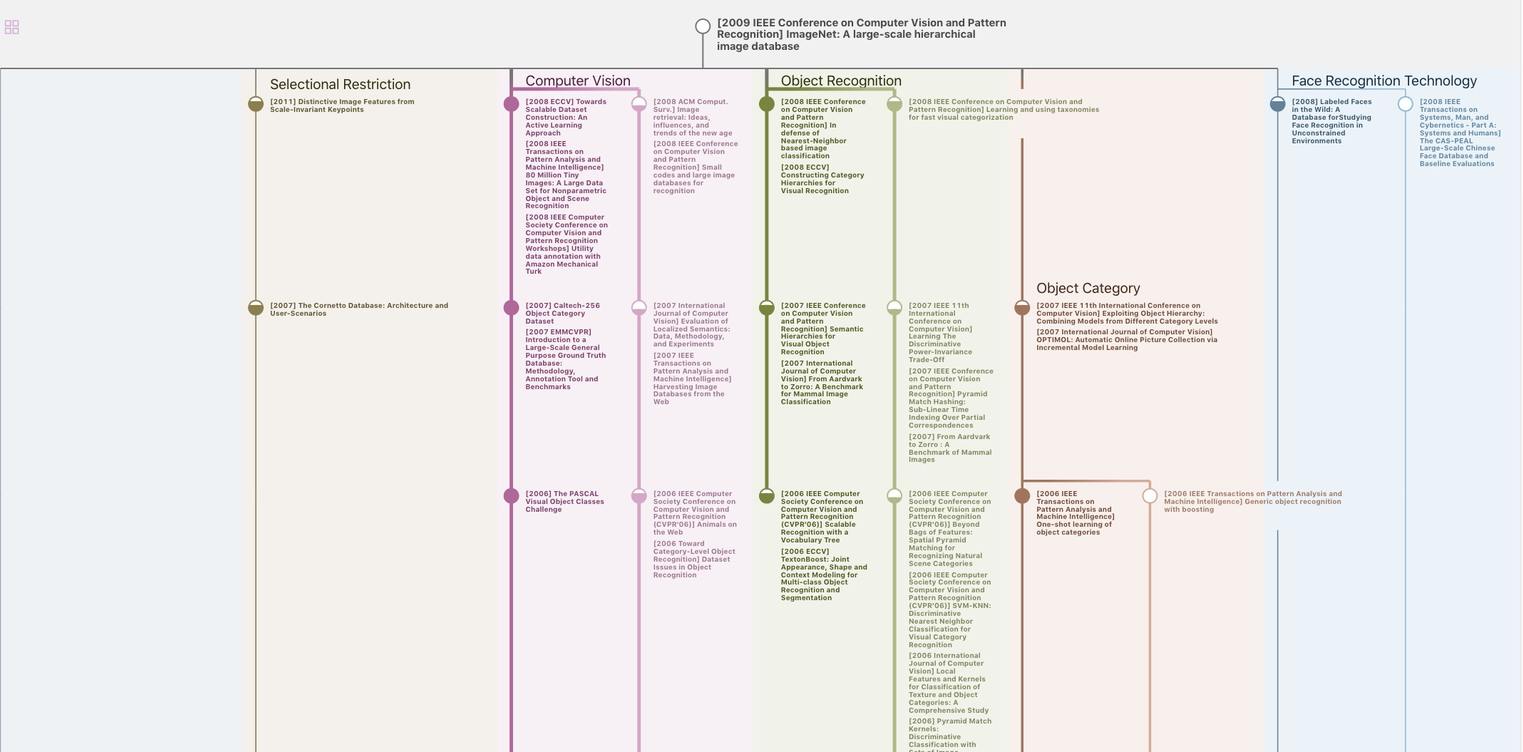
生成溯源树,研究论文发展脉络
Chat Paper
正在生成论文摘要