An Incremental Malware Classification Approach Based on Few-Shot Learning
IEEE International Conference on Communications (ICC)(2022)
摘要
Malware classification plays a fundamental role among all the related tasks. Researchers and anti-virus vendors have proposed deep learning (DL) methods to deal with the fast emerging-malware families and samples. However, for ordinary deep learning methods, once the model is trained, the set of families that can be recognized is fixed. This is troublesome in practice to deal with emerging malware families or unknown families with scarce samples. To resolve this issue, we propose an incremental classification approach called IMC (Incremental Malware Classification) based on few-shot learning, and the classifier is implemented as a cosine similarity function between extracted features and feature vectors of target classes. IMC can efficiently extend the pre-trained model to unknown families dynamically with a handful of samples without losing the ability to recognize the families it has "seen". In the process of adaptation, no re-training is needed and fast inference is realized by a single forward pass. We extensively evaluate our approach on a dataset named APIMDS where the framework achieves incremental ability to classify the unknown families with high accuracy while maintaining the ability to recognize the known families. To our best knowledge, this is the first approach to meet the requirements to unify the classification of both unknown and known malware families in a few-shot manner.
更多查看译文
关键词
Malware classification,Few-shot learning,Incremental classification,Generalization to unknown families,APIMDS
AI 理解论文
溯源树
样例
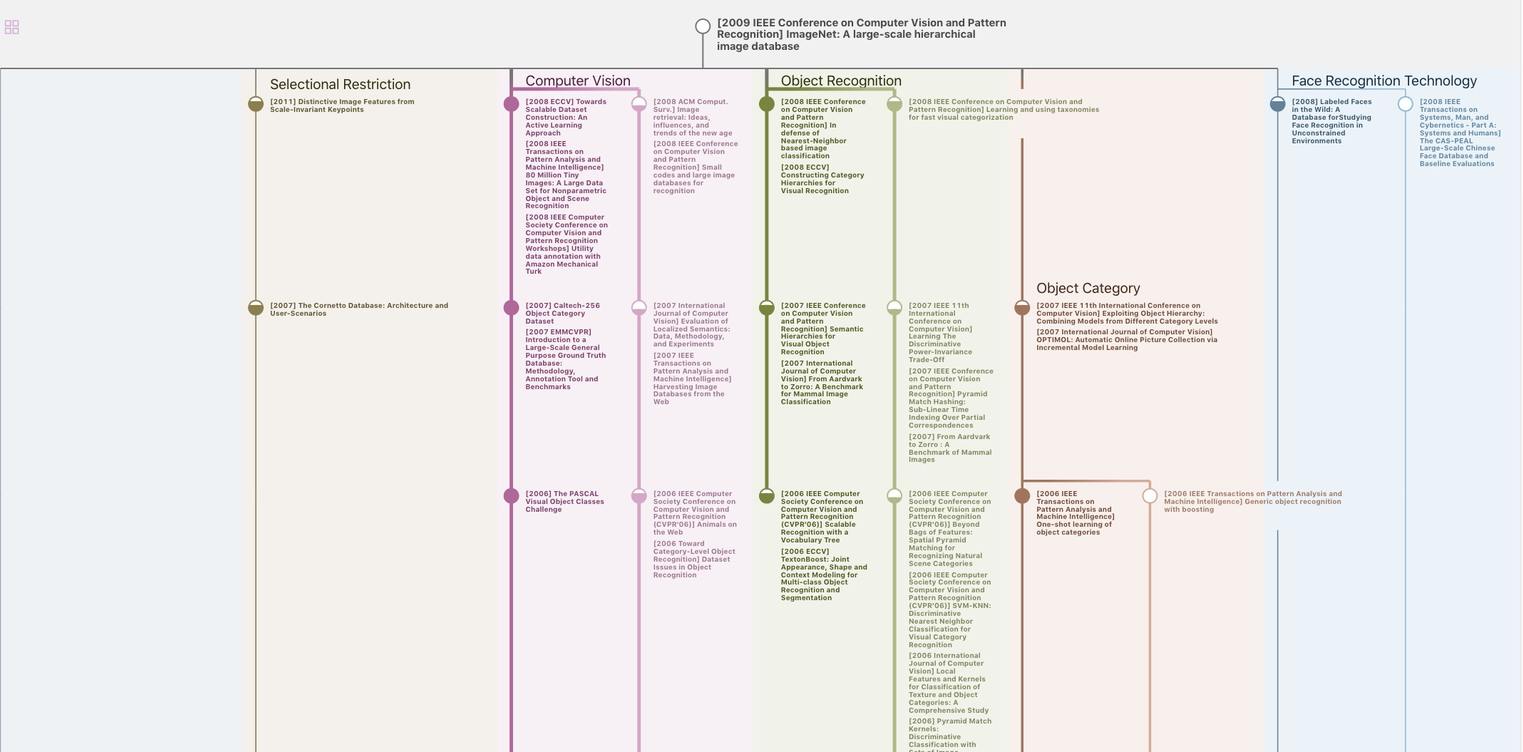
生成溯源树,研究论文发展脉络
Chat Paper
正在生成论文摘要