Traffic Data Scheduling of Frequent Application Sets for Task Offloading in Multi-access Edge Computing
IEEE International Conference on Communications (ICC)(2022)
Abstract
Frequently task offloading in the Multi-access Edge Computing (MEC) system takes up a lot of network resources, which leads to serious network congestion problems. To deal with such problems, applying data pricing strategies in task offloading to schedule traffic data is perceived as a promising solution for Internet service providers (ISPs). However, the traditional application-oriented data pricing strategies do not consider user satisfaction of the task executing process, resulting in lower ISP profit. In this paper, a novel scheme named Appset Usage Patterns-Aware task offloading scheme (AUPA) is proposed to alleviate the tension between traffic data supply and user satisfaction. We mine the sequential patterns in frequent application sets to extract temporal association rules. Then these association rules are used to design a smart data pricing strategy to guide the task offloading decision. Finally, we formulate the ISP's profit maximization problem as a nonlinear programming (NLP) problem based on partial offloading, and we simulate the scheduling processuse using the Stackelberg game model. The performance of our solution is evaluated in terms of ISP's profit, consumers' surplus, capacity utilization, and traffic efficiency. The results show that our scheme significantly improves the ISP's profit by about 20% while ensuring capacity constraints compared with other baseline schemes.
MoreTranslated text
Key words
task offloading,multi-access edge computing,data pricing,sequential pattern mining
AI Read Science
Must-Reading Tree
Example
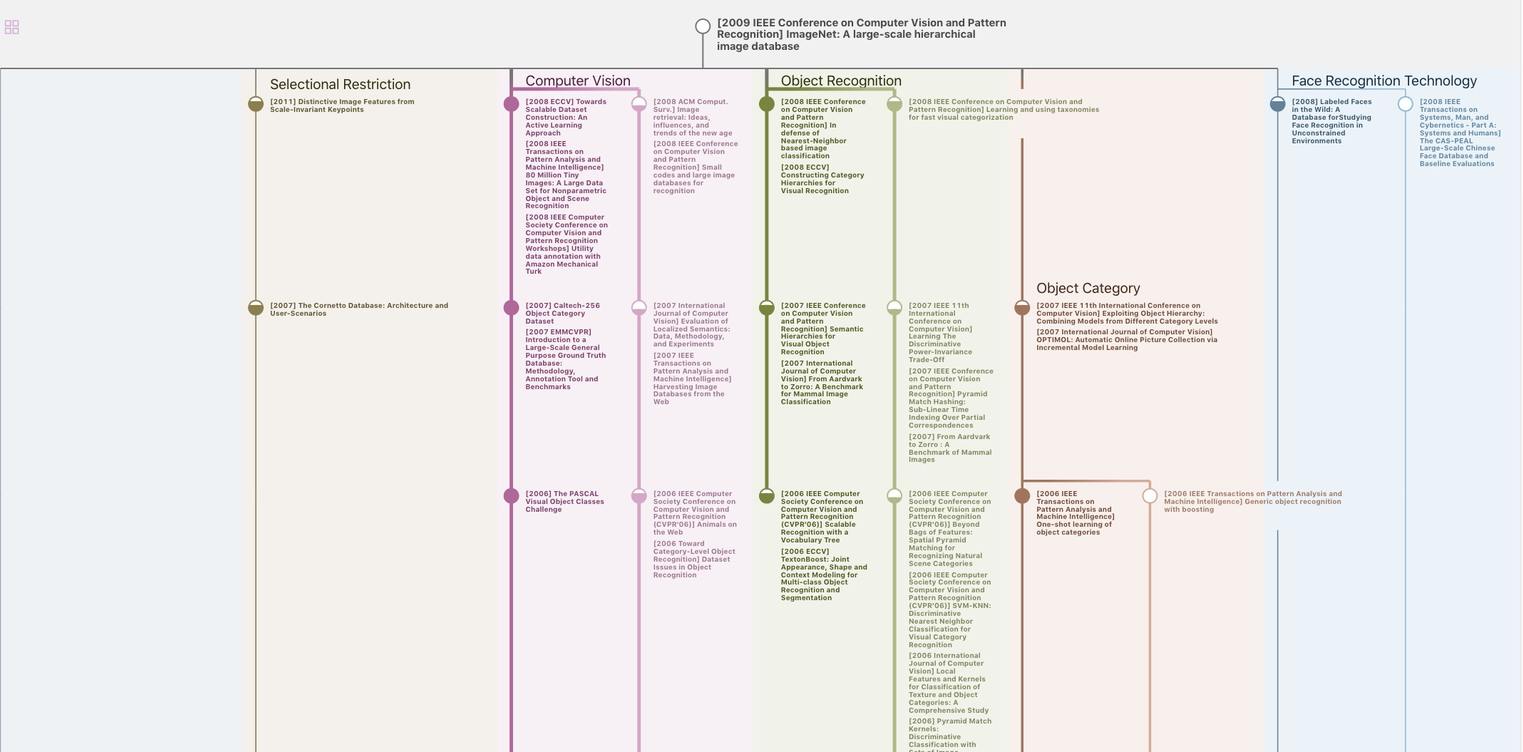
Generate MRT to find the research sequence of this paper
Chat Paper
Summary is being generated by the instructions you defined