Leveraging MIMO Transmit Diversity for Channel-Agnostic Device Identification
IEEE International Conference on Communications (ICC)(2022)
摘要
The accurate identification of wireless devices is critical for enabling automated network access monitoring and authenticated data communication in large-scale networks; e.g., IoT networks. RF fingerprinting has emerged as a potential solution for device identification by leveraging the transmitter unique manufacturing impairments of the RF components. Although deep learning is proven efficient in classifying devices based on the hardware impairments, trained models perform poorly due to channel variations. That is, although training and testing neural networks using data generated during the same period achieve reliable classification, testing them on data generated at different times degrades the accuracy substantially. To the best of our knowledge, we are the first to propose to leverage MIMO capabilities to mitigate the channel effect and provide a channel-resilient device classification. For the proposed technique we show that, for Rayleigh channels, blind partial channel estimation enabled by MIMO increases the testing accuracy by up to 40% when the models are trained and tested over the same channel, and by up to 60% when the models are tested on a channel that is different from that used for training.
更多查看译文
关键词
RF fingerprinting,transmitter unique manufacturing impairments,deep learning,channel variations,neural networks,channel-resilient device classification,Rayleigh channels,blind partial channel estimation,MIMO transmit diversity,channel-agnostic device identification,wireless devices,automated network access monitoring,authenticated data communication,large-scale networks,IoT networks
AI 理解论文
溯源树
样例
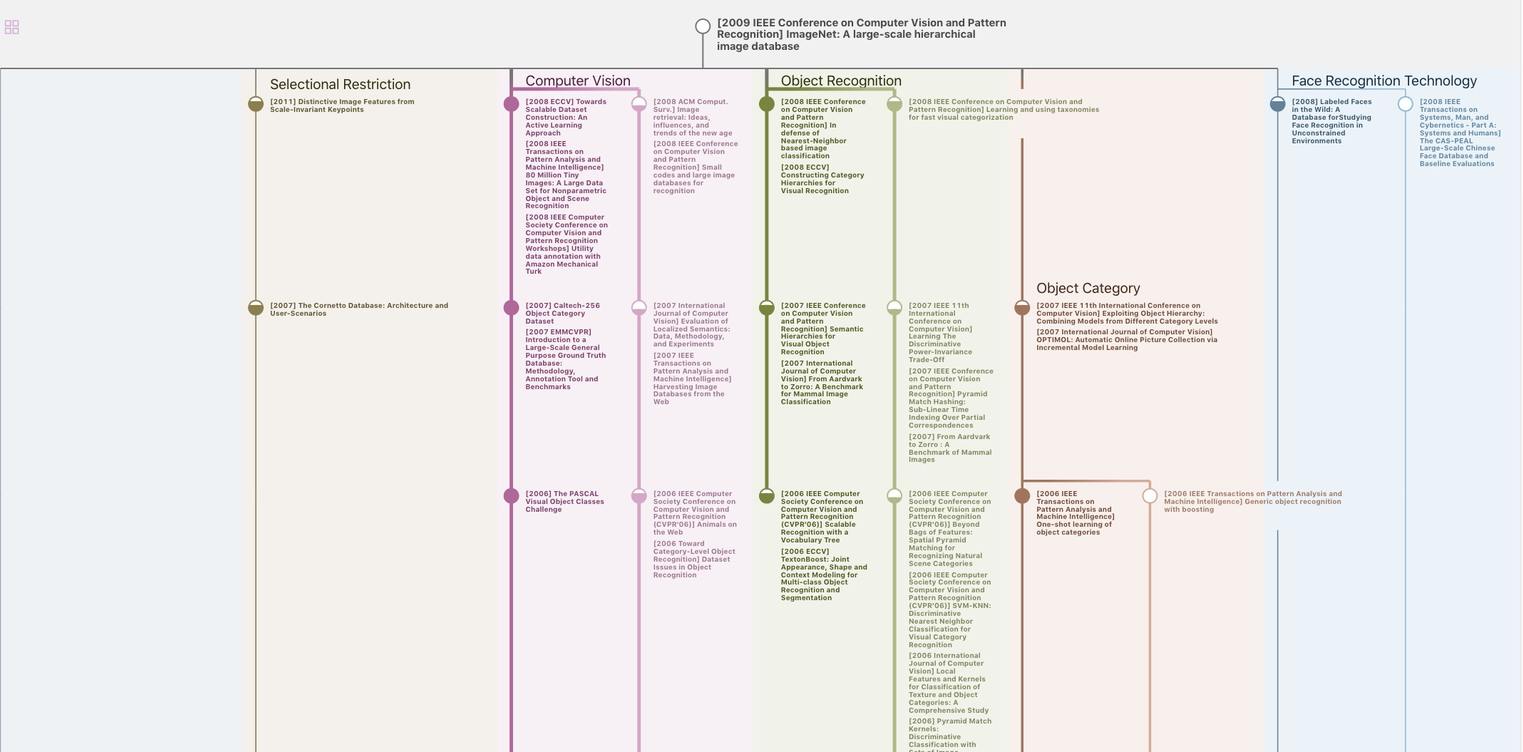
生成溯源树,研究论文发展脉络
Chat Paper
正在生成论文摘要