An attentive interpretable approach for identifying and quantifying malware-infected internet-scale IoT bots behind a NAT
ACM International Conference on Computing Frontiers (CF)(2022)
摘要
The explosive growth of the Internet-of-Things (IoT) paradigm has brought the rise of malicious activity targeting the Internet. Indeed, the lack of basic security protocols and measures in IoT devices is allowing attackers to use exploited Internet-scale IoT devices to organize malicious botnets, and cause significant damage to the Internet through Denial of Service (DoS) attacks, illicit scraping, and cryptojacking attacks. Such IoT botnets can be Internet-facing, or can also be deployed behind Network Address Translation (NAT) gateways that provide anonymity to the exploited bots. In this paper, we aim at detecting compromised IoT bots behind NAT gateways which could possibly generate malicious activities towards the Internet by leveraging large-scale macroscopic one-way darknet data. To the best of our knowledge, we are among the first to explore the capabilities of attentive interpretable tabular transformers to capture the nature of such nodes operating on one-way network traffic. Our results, which employed 2.6GB of darknet data, show that our approach was able to classify malware-infected NATed IoT bots with an accuracy of 93%, outperforming the state-of-the-art machine learning (ML) approaches. Additionally, we were able to infer around 4 million Internet-scale Mirai-infected NATed IoT bots and 16,871 unique NATed IP addresses. Results from this work put forward interesting future work in the area of network traffic analysis of NATed IoT bots for better Internet security, while highlighting the need for addressing the notions of attention and interpretability.
更多查看译文
关键词
Network traffic analysis, Internet of Things, IoT fingerprinting, Network Address Translation, Network Telescope, Machine Learning, Transformers, Attention
AI 理解论文
溯源树
样例
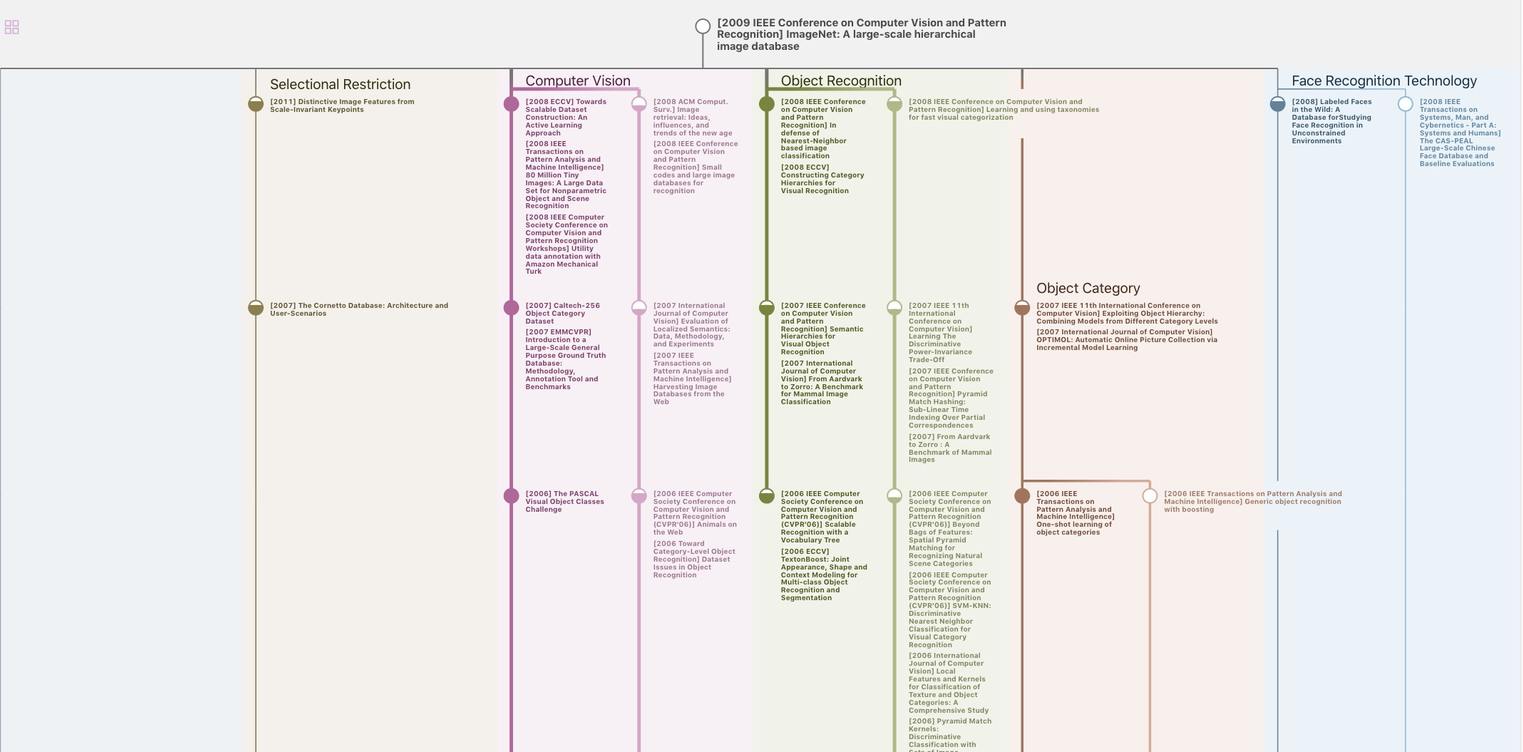
生成溯源树,研究论文发展脉络
Chat Paper
正在生成论文摘要