Graph Dictionary Learning for 3-D Point Cloud Compression
2022 Data Compression Conference (DCC)(2022)
摘要
3-D point clouds rendering solid representations of scenes or objects often carry a tremendous amount of points, compulsorily requesting high-efficiency compression for storage and transmission. In this paper, we propose a novel
$p$
-Laplacian embedding graph dictionary learning algorithm for 3-D point cloud attribute compression. The proposed method integrates the underlying graph topology to the learned graph dictionary capitalizing on
$p$
-Laplacian eigenfunctions and leads to parsimonious representations of 3-D point clouds. We further devise alternating optimization with the help of ADMM to efficiently solve the resulting non-convex minimization problem.
更多查看译文
关键词
high-efficiency compression,novel p-Laplacian embedding graph dictionary,underlying graph topology,learned graph dictionary,3-D point cloud compression
AI 理解论文
溯源树
样例
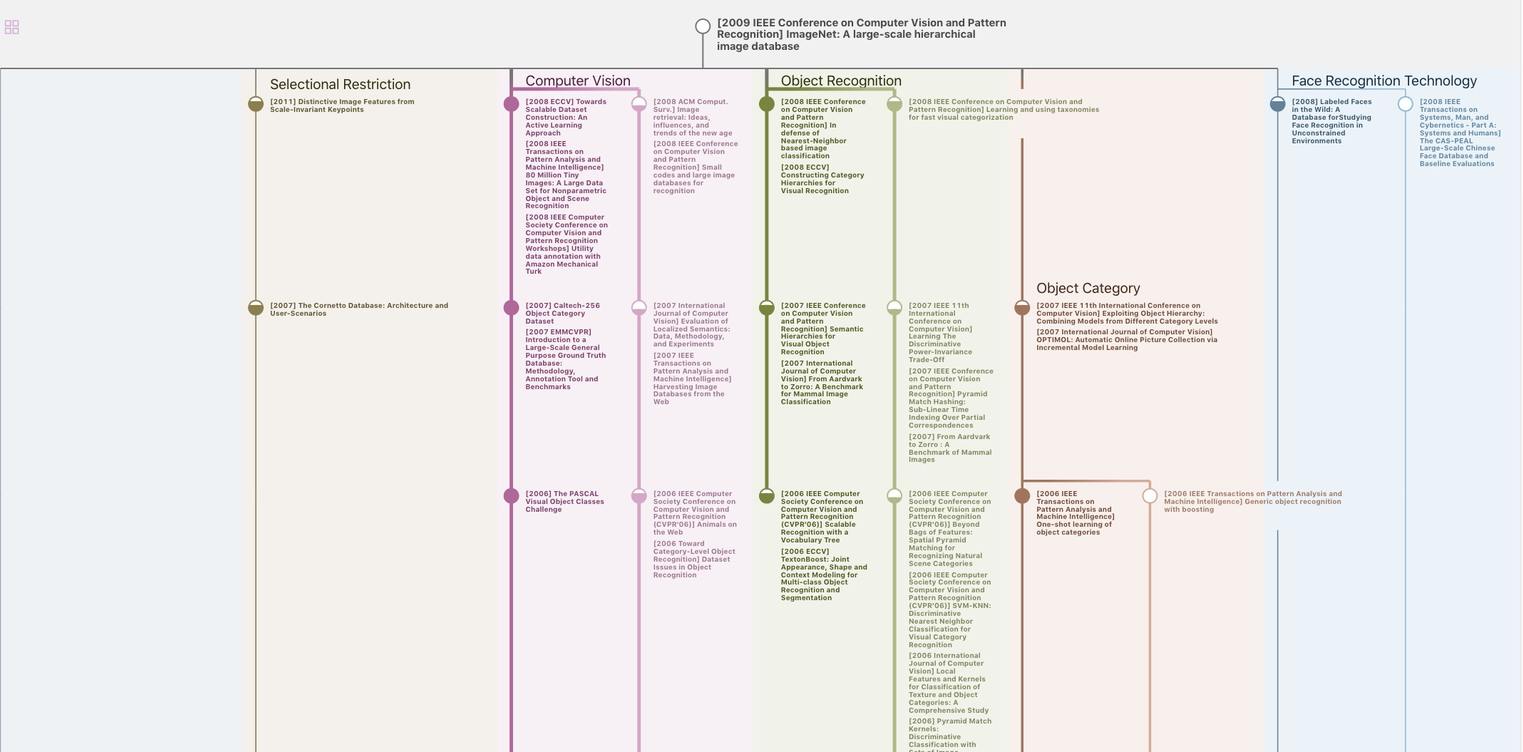
生成溯源树,研究论文发展脉络
Chat Paper
正在生成论文摘要