Coarse-to-fine Prediction With Local and Nonlocal Correlations for Intra Coding
2022 Data Compression Conference (DCC)(2022)
Abstract
Recently many efforts have been devoted to learning non-linear predictions from neighboring samples with deep neural networks. However, existing methods mainly generate predictions with local reference samples, regardless of nonlocal self-similarity. In this paper, we aim to incorporate local and nonlocal correlations for intra prediction and propose a two-stage coarse-to-fine network (CTFN), which is integrated into VVC codec as an optional intra prediction mode. The prediction process of CTFN is decomposed into two stages. In the first stage, we train a set of networks to generate a coarse result with local reference samples. In the second stage, we extract sufficient features from nonlocal region using the coarse result as priors and transform the features into a fine prediction result. In particular, a patch-wise attention layer (PAL) is designed in the second stage that can fully explore nonlocal correlations in feature domain and assign weights to each nonlocal feature adaptively, as shown in Fig. 1. As such, the proposed CTFN can not only learn a non-linear mapping from local context, but also explicitly borrow similar features from nonlocal region in a weighted form. Different from image inpainting tasks, the patch synthesis problem is converted to patch matching problem with the CTFN, yielding more reliable predictions. More-over, we construct a classified dataset based on Pearson Correlation Coefficient for network training to better handle contents that are highly correlated. Experiments on VTM-11.0 show that the proposed network achieves 1.77% ED-rate reductions under all intra configuration, which outperforms the state-of-the-art methods.
MoreTranslated text
Key words
intra coding,deep neural networks,local reference samples,nonlocal self-similarity,nonlocal correlations,two-stage coarse-to-fine network,CTFN,optional intra prediction mode,prediction process,sufficient feature extraction,nonlocal region,patch-wise attention layer,feature domain,nonlocal feature,nonlinear mapping,Pearson correlation coefficient,network training,intra configuration,coarse-to-fine prediction,local correlations,nonlinear prediction learning,VVC codec,image inpainting tasks,patch synthesis problem,weighted form,classified dataset
AI Read Science
Must-Reading Tree
Example
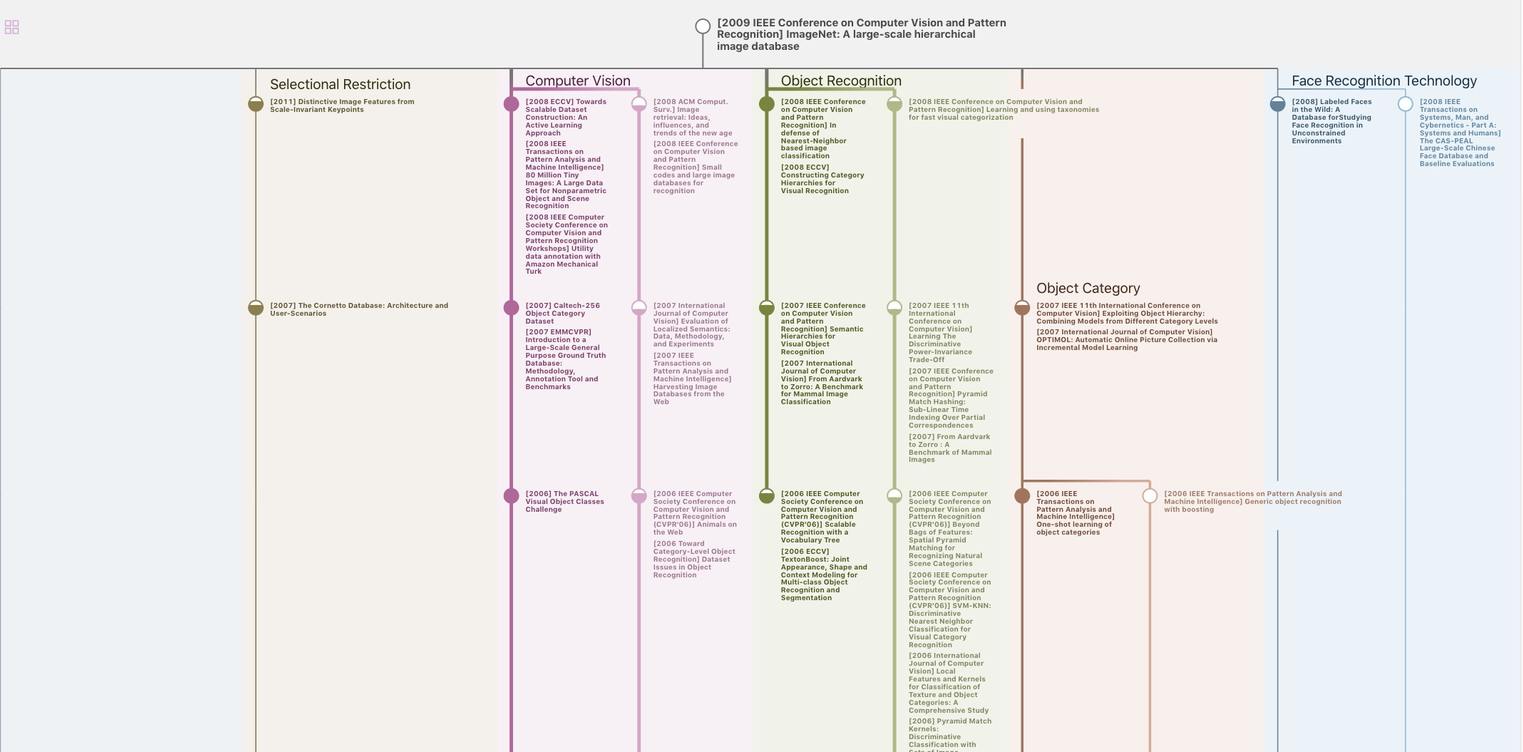
Generate MRT to find the research sequence of this paper
Chat Paper
Summary is being generated by the instructions you defined