Compressing Transformer-Based ASR Model by Task-Driven Loss and Attention-Based Multi-Level Feature Distillation
IEEE International Conference on Acoustics, Speech, and Signal Processing (ICASSP)(2022)
摘要
The current popular knowledge distillation (KD) methods effectively compress the transformer-based end-to-end speech recognition model. However, existing methods fail to utilize complete information of the teacher model, and they distill only a limited number of blocks of the teacher model. In this study, we first integrate a task-driven loss function into the decoder's intermediate blocks to generate task-related feature representations. Then, we propose an attention-based multi-level feature distillation to automatically learn the feature representation summarized by all blocks of the teacher model. Under the 1.1M parameters model, the experimental results on the Wall Street Journal dataset reveal that our approach achieves a 12.1% WER reduction compared with the baseline system.
更多查看译文
关键词
speech recognition,feature distillation,model compression,task-driven loss,transformer
AI 理解论文
溯源树
样例
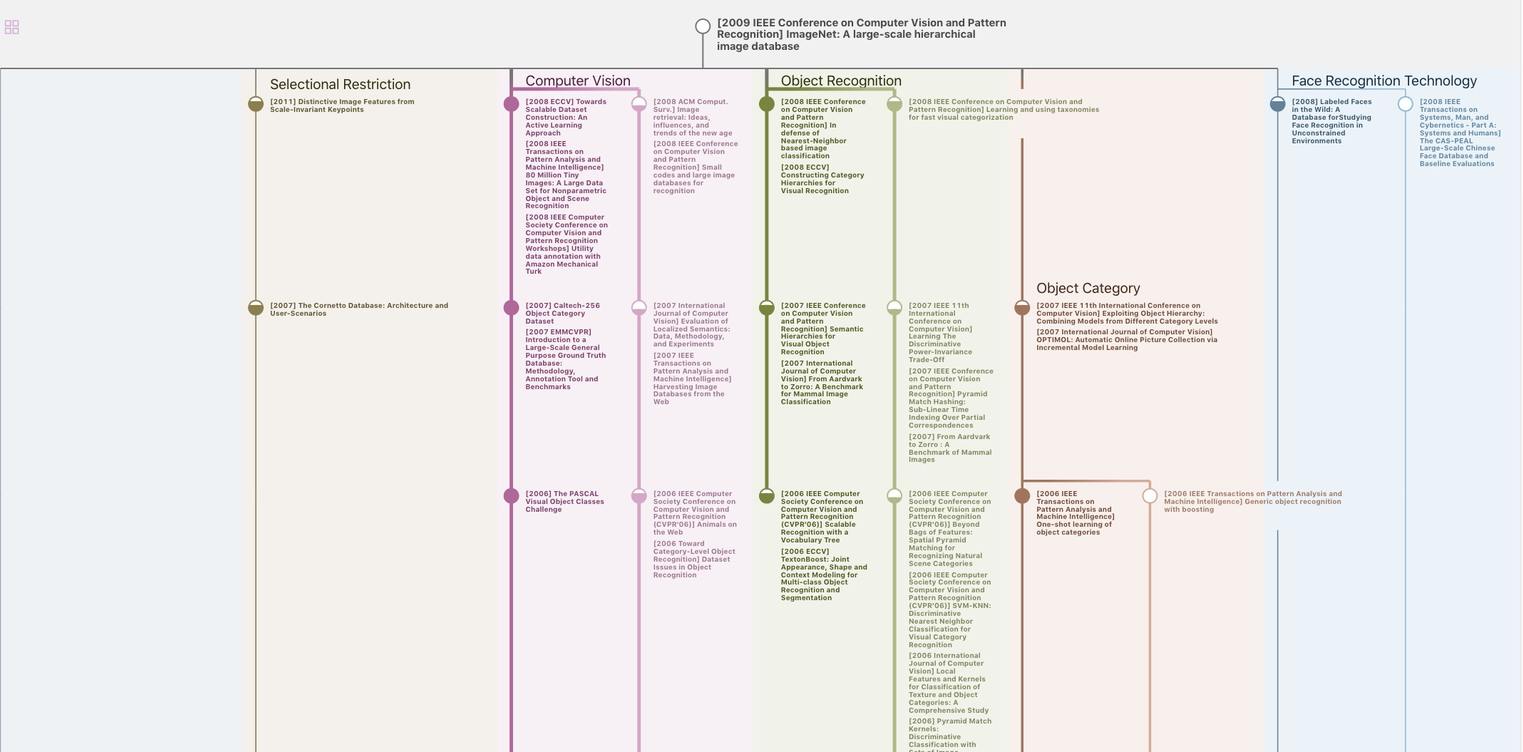
生成溯源树,研究论文发展脉络
Chat Paper
正在生成论文摘要